Dpanet: A Novel Network Based On Dense Pyramid Feature Extractor And Dual Correlation Analysis Attention Modules For Colon Glands Segmentation
UNCERTAINTY FOR SAFE UTILIZATION OF MACHINE LEARNING IN MEDICAL IMAGING AND CLINICAL IMAGE-BASED PROCEDURES(2019)
摘要
Accurate segmentation of glands from histology images is a crucial step to obtain reliable morphological statistics for quantitative diagnosis. However, this task is formidable because of the enormous variability in glandular appearance and the difficulty in distinguishing between glandular and non-glandular histological structures. To address this challenge, a novel neural network is proposed to effectively extract benign or malignant colon glands from histology images. Our network has the following innovations: (1) Under the same resolution space, multi-receptive field pyramid features are captured from dense multi-rate dilated convolution architecture with sounder dilated rate setting for accurate gland segmentation. (2) Furthermore, the extracted features are down-sampled by B-spline algorithm to provide different resolution information for the network. (3) Specifically, we embed spatial-channel soft attention modules before each deconvolution operation in the decoder phase, which can better reintegrate features and support clear semantic similarity for network. All these unique operations boost the performance of our method on gland segmentation task. We achieve state-of-the-art performance on the publicly available Warwick-QU dataset.
更多查看译文
关键词
Colon glands segmentation, Correlation analysis, Attention module, Spatial pyramid feature
AI 理解论文
溯源树
样例
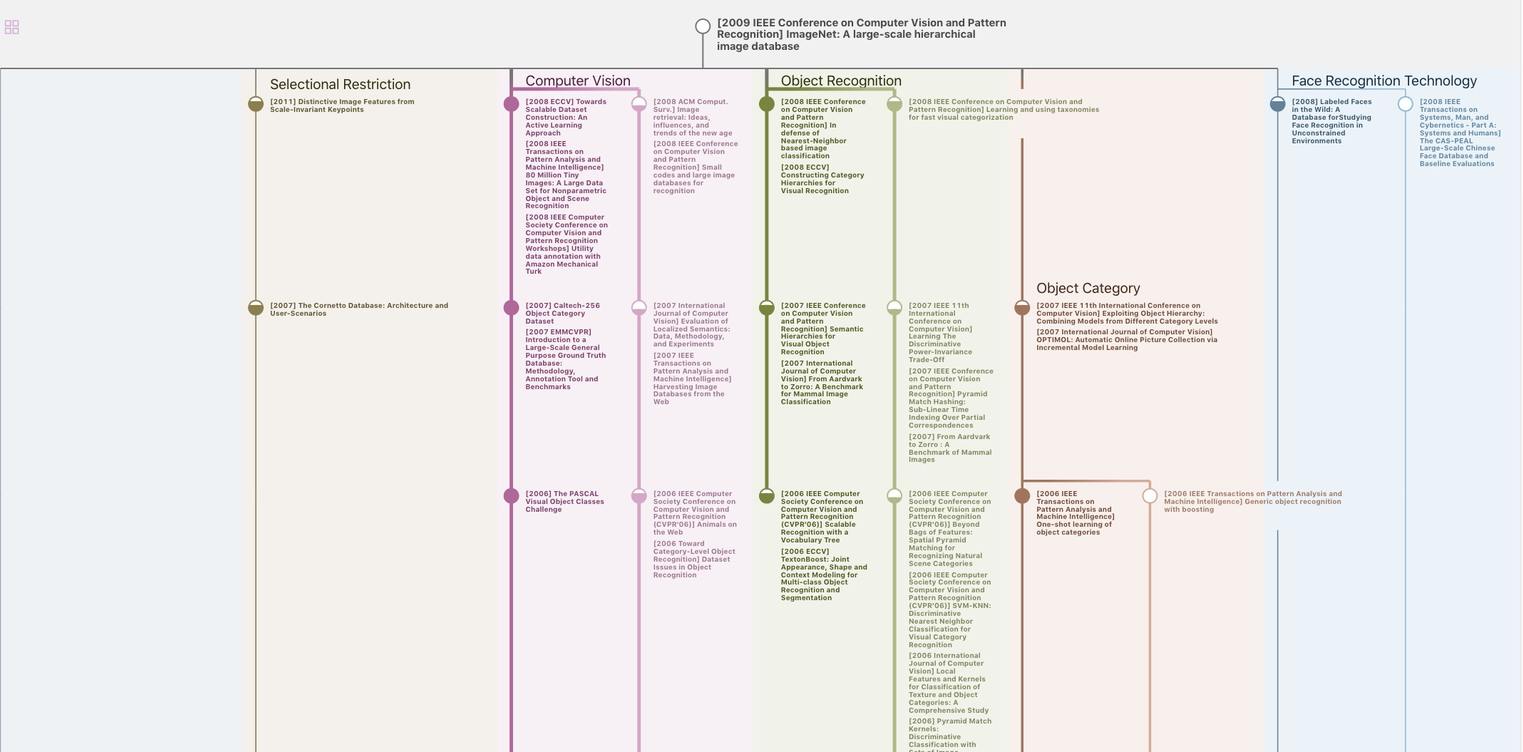
生成溯源树,研究论文发展脉络
Chat Paper
正在生成论文摘要