Adaptive Transfer Learning of Multi-View Time Series Classification
arxiv(2019)
摘要
Time Series Classification (TSC) has been an important and challenging task in data mining, especially on multivariate time series and multi-view time series data sets. Meanwhile, transfer learning has been widely applied in computer vision and natural language processing applications to improve deep neural network's generalization capabilities. However, very few previous works applied transfer learning framework to time series mining problems. Particularly, the technique of measuring similarities between source domain and target domain based on dynamic representation such as density estimation with importance sampling has never been combined with transfer learning framework. In this paper, we first proposed a general adaptive transfer learning framework for multi-view time series data, which shows strong ability in storing inter-view importance value in the process of knowledge transfer. Next, we represented inter-view importance through some time series similarity measurements and approximated the posterior distribution in latent space for the importance sampling via density estimation techniques. We then computed the matrix norm of sampled importance value, which controls the degree of knowledge transfer in pre-training process. We further evaluated our work, applied it to many other time series classification tasks, and observed that our architecture maintained desirable generalization ability. Finally, we concluded that our framework could be adapted with deep learning techniques to receive significant model performance improvements.
更多查看译文
关键词
adaptive transfer learning,classification,multi-view
AI 理解论文
溯源树
样例
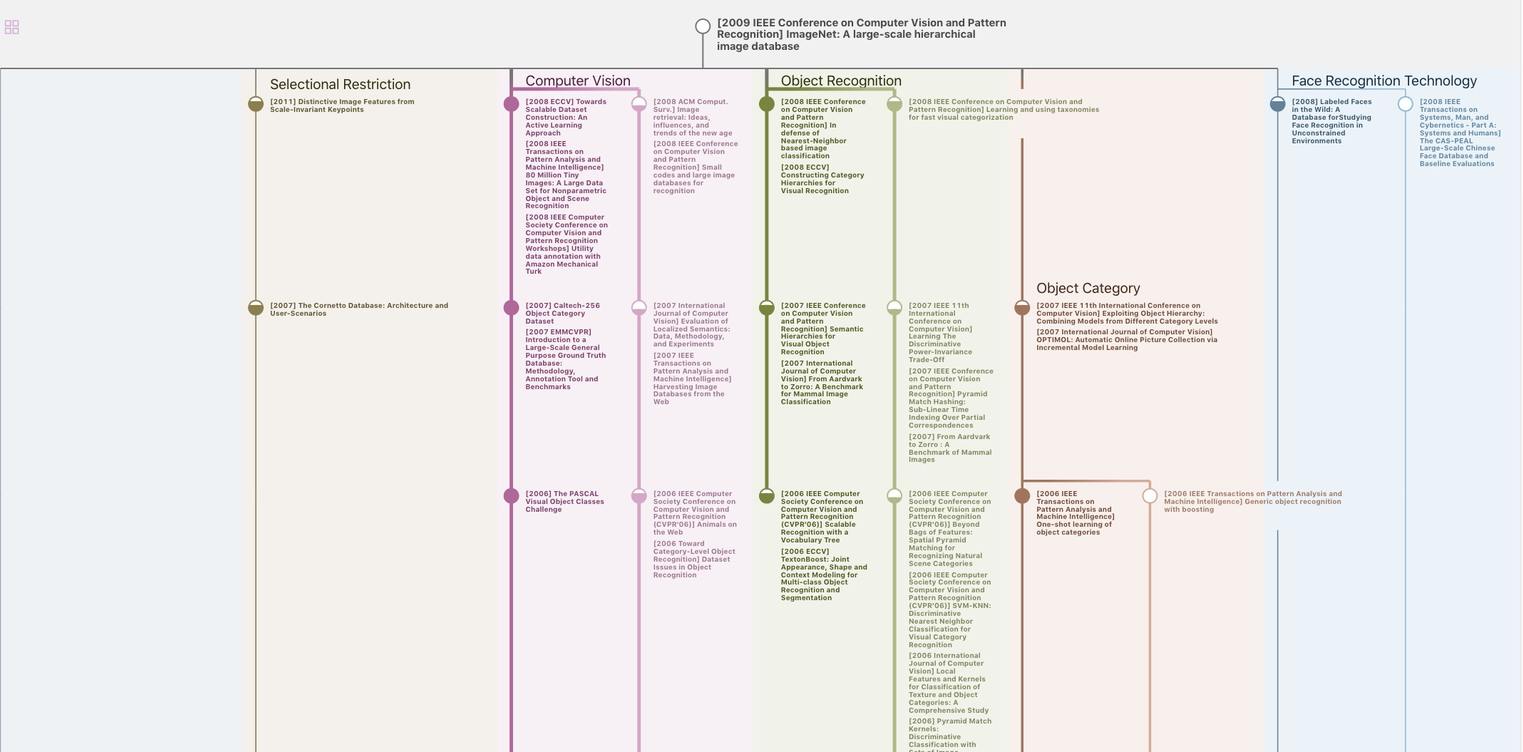
生成溯源树,研究论文发展脉络
Chat Paper
正在生成论文摘要