Automated Left Ventricle Dimension Measurement in 2D Cardiac Ultrasound via an Anatomically Meaningful CNN Approach
SUSI/PIPPI@MICCAI(2019)
摘要
Two-dimensional echocardiography (2DE) measurements of left ventricle (LV) dimensions are highly significant markers of several cardiovascular diseases. These measurements are often used in clinical care despite suffering from large variability between observers. This variability is due to the challenging nature of accurately finding the correct temporal and spatial location of measurement endpoints in ultrasound images. These images often contain fuzzy boundaries and varying reflection patterns between frames. In this work, we present a convolutional neural network (CNN) based approach to automate 2DE LV measurements. Treating the problem as a landmark detection problem, we propose a modified U-Net CNN architecture to generate heatmaps of likely coordinate locations. To improve the network performance we use anatomically meaningful heatmaps as labels and train with a multi-component loss function. Our network achieves 13.4 (IVS), LV internal dimension (LVID), and LV posterior wall (LVPW) measurements respectively. The design outperforms other networks and matches or approaches intra-analyser expert error.
更多查看译文
关键词
Ultrasound, Echocardiography, Landmark detection, Deep learning, Convolutional neural networks
AI 理解论文
溯源树
样例
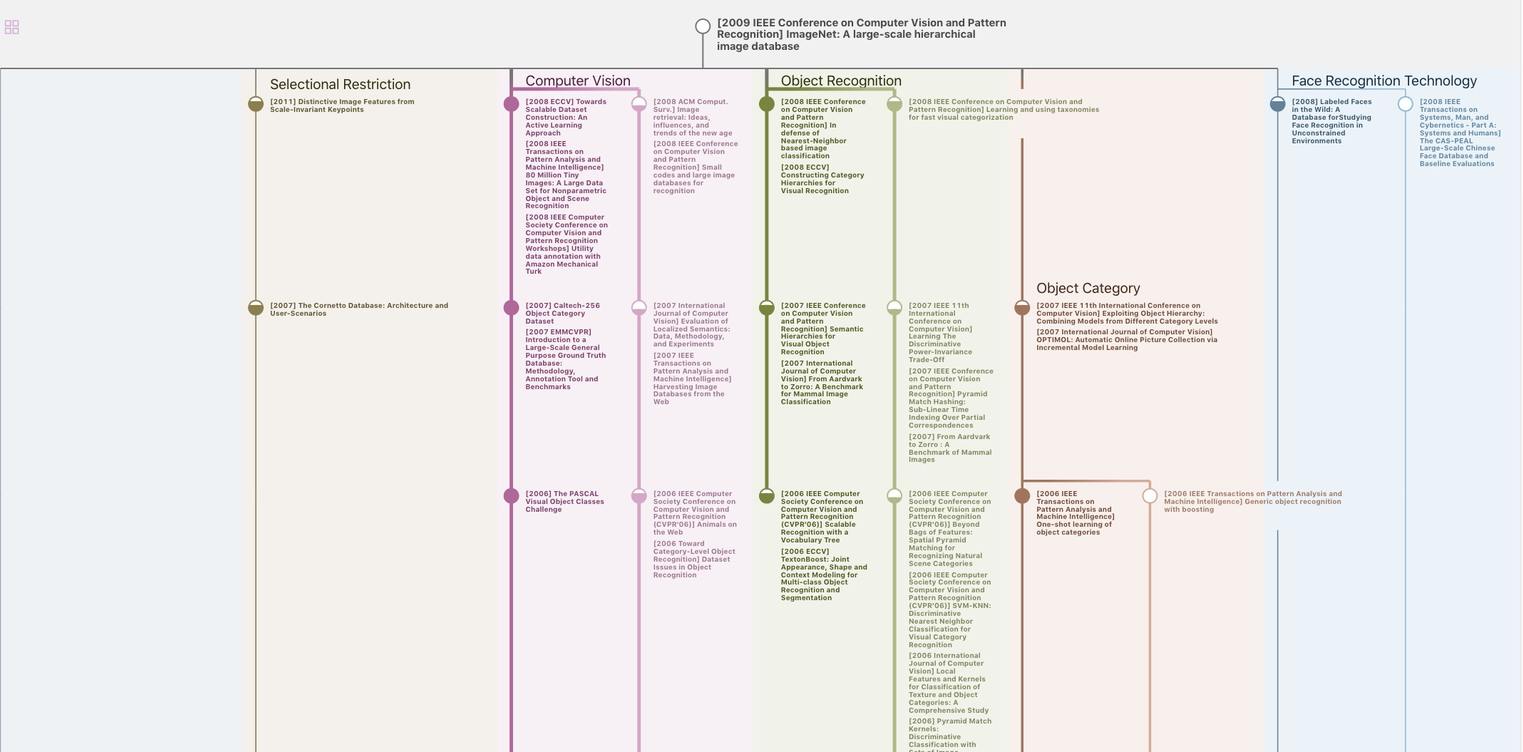
生成溯源树,研究论文发展脉络
Chat Paper
正在生成论文摘要