Facial Recognition Task For The Classification Of Mild Cognitive Impairment With Ensemble Sparse Classifier
2019 41ST ANNUAL INTERNATIONAL CONFERENCE OF THE IEEE ENGINEERING IN MEDICINE AND BIOLOGY SOCIETY (EMBC)(2019)
摘要
Conventional methods for detecting mild cognitive impairment (MCI) require cognitive exams and follow-up neuroimaging, which can be time-consuming and expensive. A great need exists for objective and cost-effective biomarkers for the early detection of MCI. This study uses a sequential imaging oddball paradigm to determine if familiar, unfamiliar, or inverted faces are effective visual stimuli for the early detection of MCI. Unlike the traditional approach where the amplitude and latency of certain deflection points of event-related potentials (ERPs) are selected as electrophysiological biomarkers (or features) of MCI, we used the entire ERPs as potential biomarkers and relied on an advanced machine-learning technique, i.e. an ensemble of sparse classifier (ESC), to choose the set of features to best discriminate MCI from healthy controls. Five MCI subjects and eight age-matched controls were given the MoCA exam before EEG recordings in a sensory-deprived room. Traditional time-domain comparisons of averaged ERPs between the two groups did not yield any statistical significance. However, ESC was able to discriminate MCI from controls with 95% classification accuracy based on the averaged ERPs elicited by familiar faces. By adopting advanced machine-learning techniques such as ESC, it may be possible to accurately diagnose MCI based on the ERPs that are specifically elicited by familiar faces.
更多查看译文
关键词
Automation,Cognitive Dysfunction,Facial Expression,Facial Recognition,Humans,Machine Learning
AI 理解论文
溯源树
样例
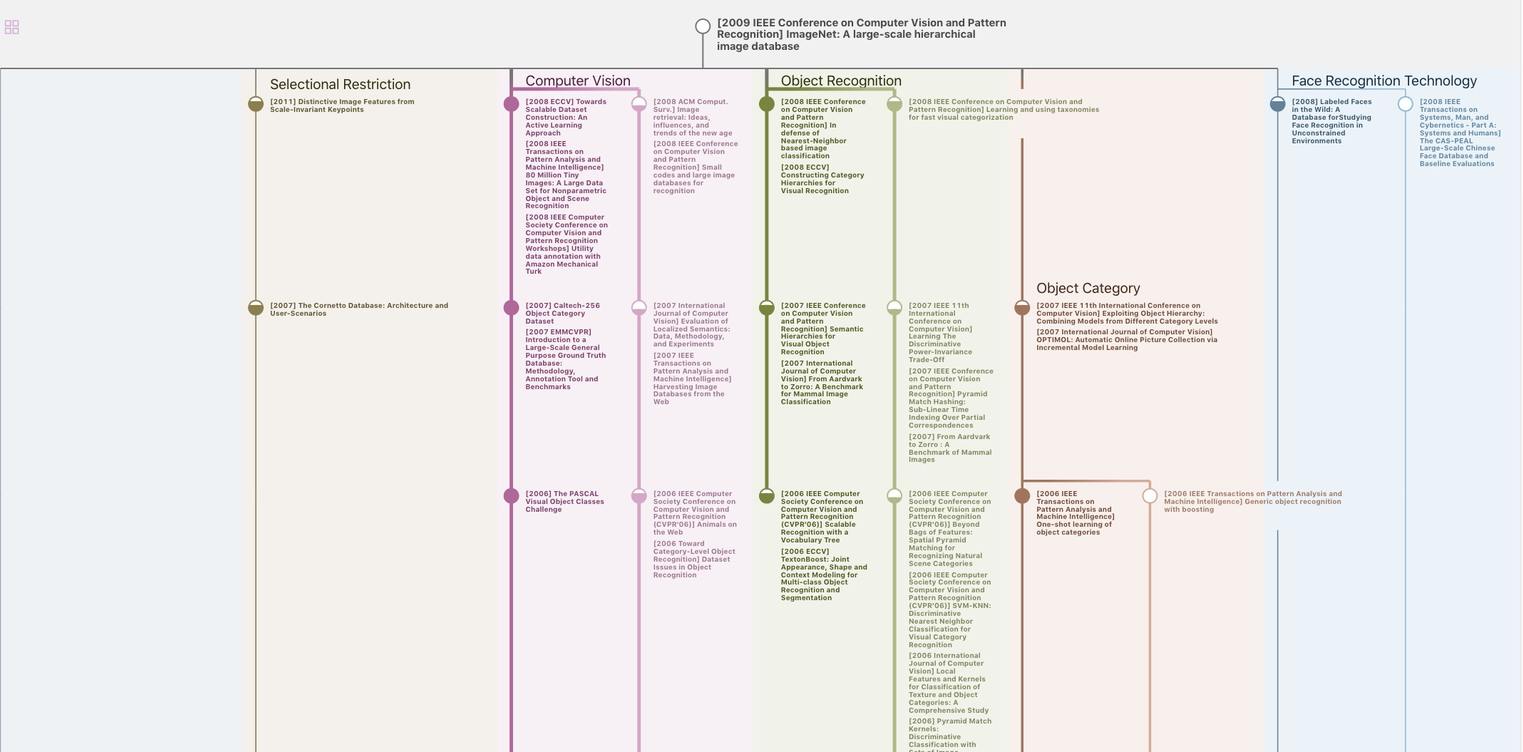
生成溯源树,研究论文发展脉络
Chat Paper
正在生成论文摘要