Cardiovascular Disease Diagnosis Using Cross-Domain Transfer Learning
2019 41ST ANNUAL INTERNATIONAL CONFERENCE OF THE IEEE ENGINEERING IN MEDICINE AND BIOLOGY SOCIETY (EMBC)(2019)
摘要
While cardiovascular diseases (CVDs) are commonly diagnosed by cardiologists via inspecting electrocardiogram (ECG) waveforms, these decisions can be supported by a data-driven approach, which may automate this process. An automatic diagnostic approach often employs hand-crafted features extracted from ECG waveforms. These features, however, do not generalise well, challenged by variation in acquisition settings such as sampling rate and mounting points. Existing deep learning (DL) approaches, on the other hand, extract features from ECG automatically but require construction of dedicated networks that require huge data and computational resource if trained from scratch. Here we propose an end-to-end trainable cross-domain transfer learning for CVD classification from ECG waveforms, by utilising existing vision-based CNN frameworks as feature extractors, followed by ECG feature learning layers. Because these frameworks are designed for image inputs, we employ a stacked spectrogram representation of multi-lead ECG waveforms as a preprocessing step. We also proposed a fusion of multiple ECG leads, using plausible stacking arrangements of the spectrograms, to encode their spatial relations. The proposed approach is validated on multiple ECG datasets and competitive performance is achieved.
更多查看译文
关键词
Transfer Learning, Cardiovascular Disease, Deep Learning, Health Informatics
AI 理解论文
溯源树
样例
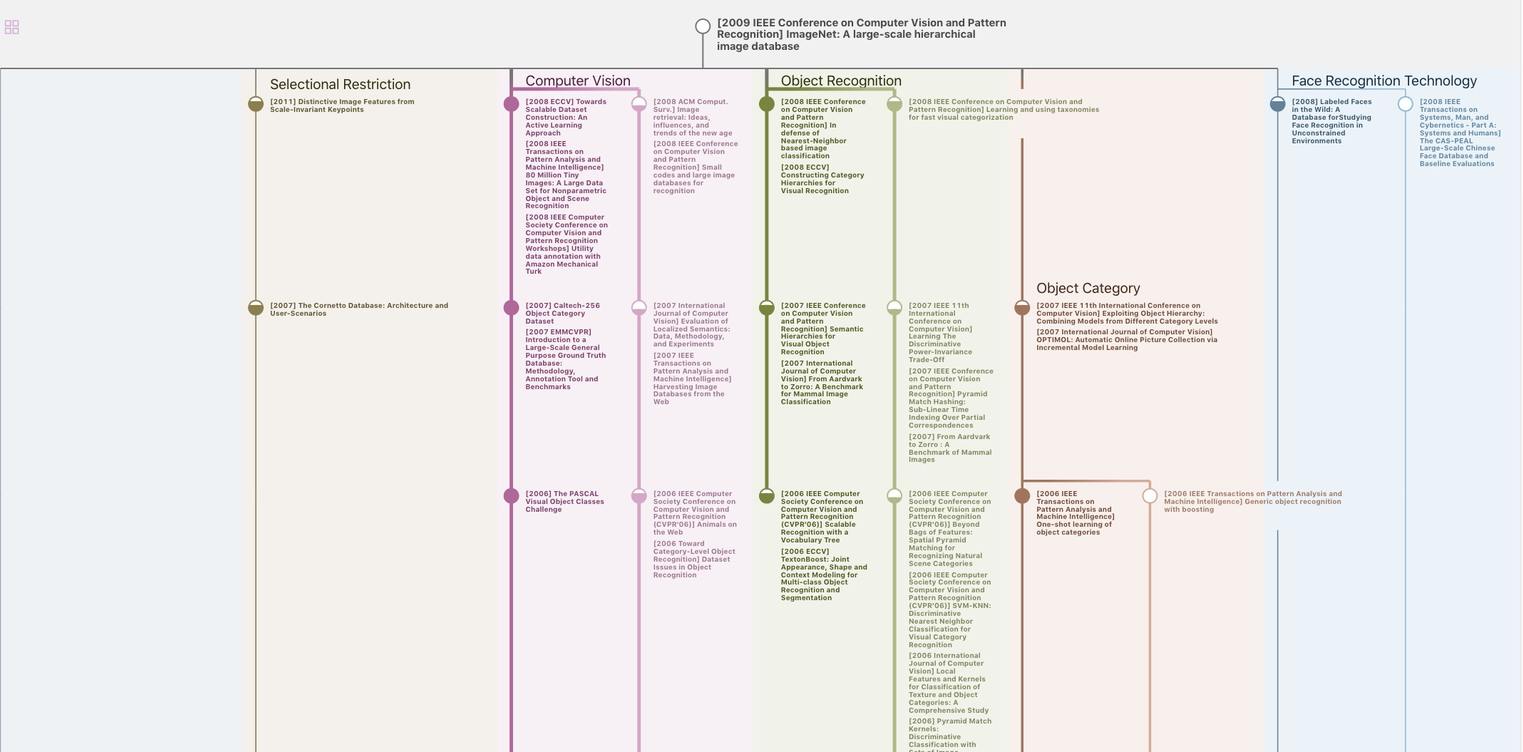
生成溯源树,研究论文发展脉络
Chat Paper
正在生成论文摘要