Feature Space Reduction for Single Trial EEG Classification based on Wavelet Decomposition
2019 41ST ANNUAL INTERNATIONAL CONFERENCE OF THE IEEE ENGINEERING IN MEDICINE AND BIOLOGY SOCIETY (EMBC)(2019)
摘要
In contrary to recent signal/information processing advancements, human brain remains the most intriguing signal processing unit in existence with inconceivable capabilities to fuse various multi-modal signals, adaptively and in real-time fashion. To connect brain with the outer world, brain computer interfacing (BCI) via Electroencephalography (EEG) signals has received extensive attention. Extracting informative and discriminating features from EEG signals and decomposing the recorded signals into their underlying components is believed to yield compromising results. Different algorithms, therefore, are recently proposed combining signal decomposition techniques (e.g., spectral filterbanks, and Wavelet decomposition) with feature extracting methodologies (e.g., common spatial patterns (CSP), and Riemannian manifold learning). Although coupling filterbanks and Wavelet with the CSP has been investigated, to best of our knowledge, the potentials of coupling Wavelet with Riemannian manifold learning are not yet studied. The paper addresses this gap. In particular, we propose a level-based classification approach that couples the Wavelet decomposition with Riemannian manifold spatial learning (WvRiem). In the proposed WvRiem framework, the EEG signals are decomposed into several components (levels) and then spatial filtering via Riemannian manifold learning is performed on the best level which yields the most discriminating features. The proposed WvRiem is evaluated on the BCI Competition IV
2a
dataset and noticeably outperforms its counterparts.
更多查看译文
关键词
Brain-computer Interface (BCI), Riemannian Manifold Learning, Wavelet Transforms
AI 理解论文
溯源树
样例
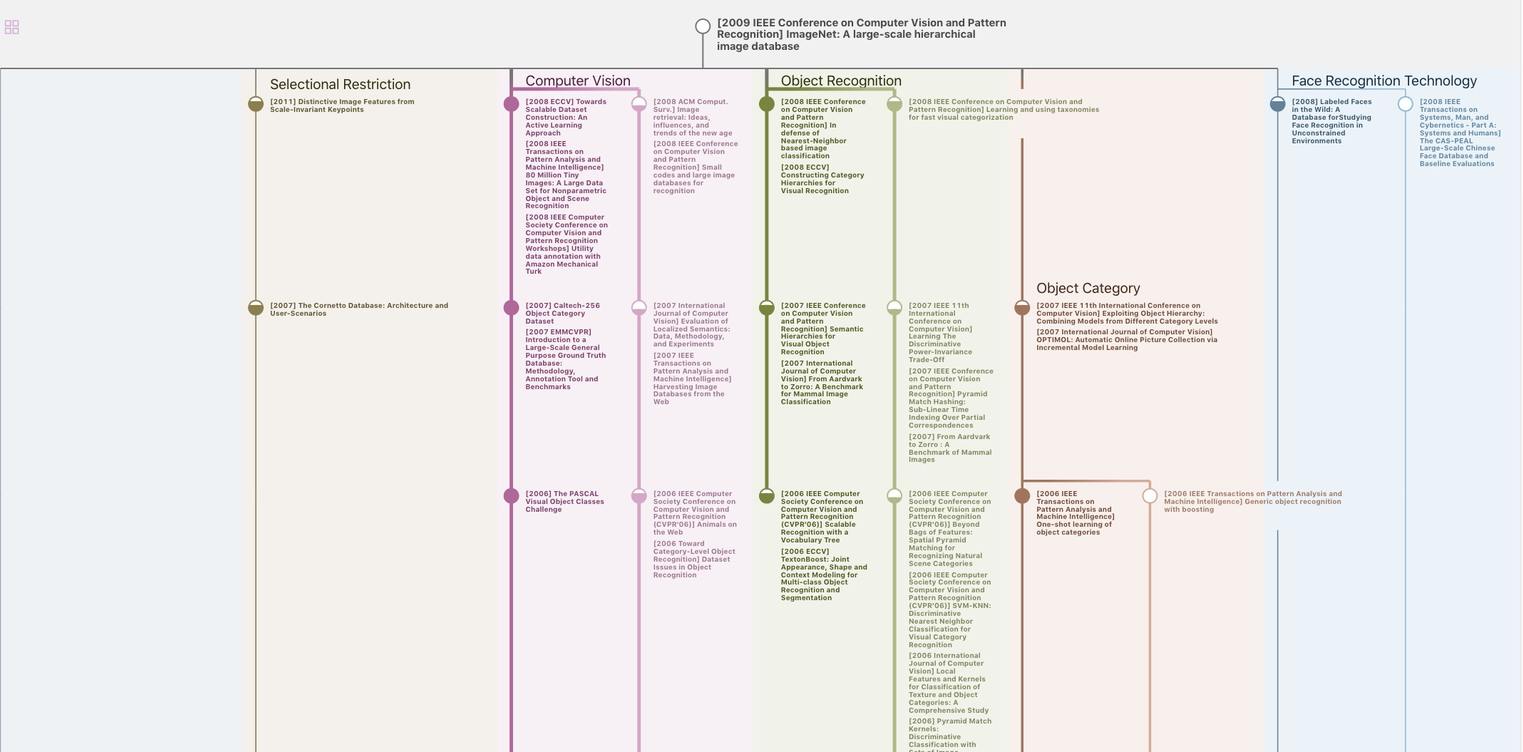
生成溯源树,研究论文发展脉络
Chat Paper
正在生成论文摘要