High- and Low-Level Feature Enhancement for Medical Image Segmentation.
Lecture Notes in Computer Science(2019)
摘要
The fully convolutional networks (FCNs) have achieved state-of-the-art performance in numerous medical image segmentation tasks. Most FCNs typically focus on fusing features in different levels to improve the learning ability to multi-scale features. In this paper, we explore an alternative direction to improve network performance by enhancing the encoding quality of high- and low-level features, so as to introduce two feature enhancement modules: (i) high-level feature enhancement module (HFE); (ii) low-level feature enhancement module (LFE). HFE utilizes attention mechanism to selectively aggregate the optimal feature information in high- and low-levels, enhancing the ability of high-level features to reconstruct accurate details. LFE aims to use global semantic information of high-level features to adaptively guide feature learning of bottom networks, so as to enhance the semantic consistency of high- and low-level features. We integrate HFE and LFE into a typical encoder-decoder network, and propose a novel medical image segmentation framework (HLF-Net). On two challenging datasets of skin lesion segmentation and spleen segmentation, we prove that the proposed modules and network can improve the performance considerably.
更多查看译文
关键词
Medical image,Segmentation,Feature enhancement,Convolutional networks
AI 理解论文
溯源树
样例
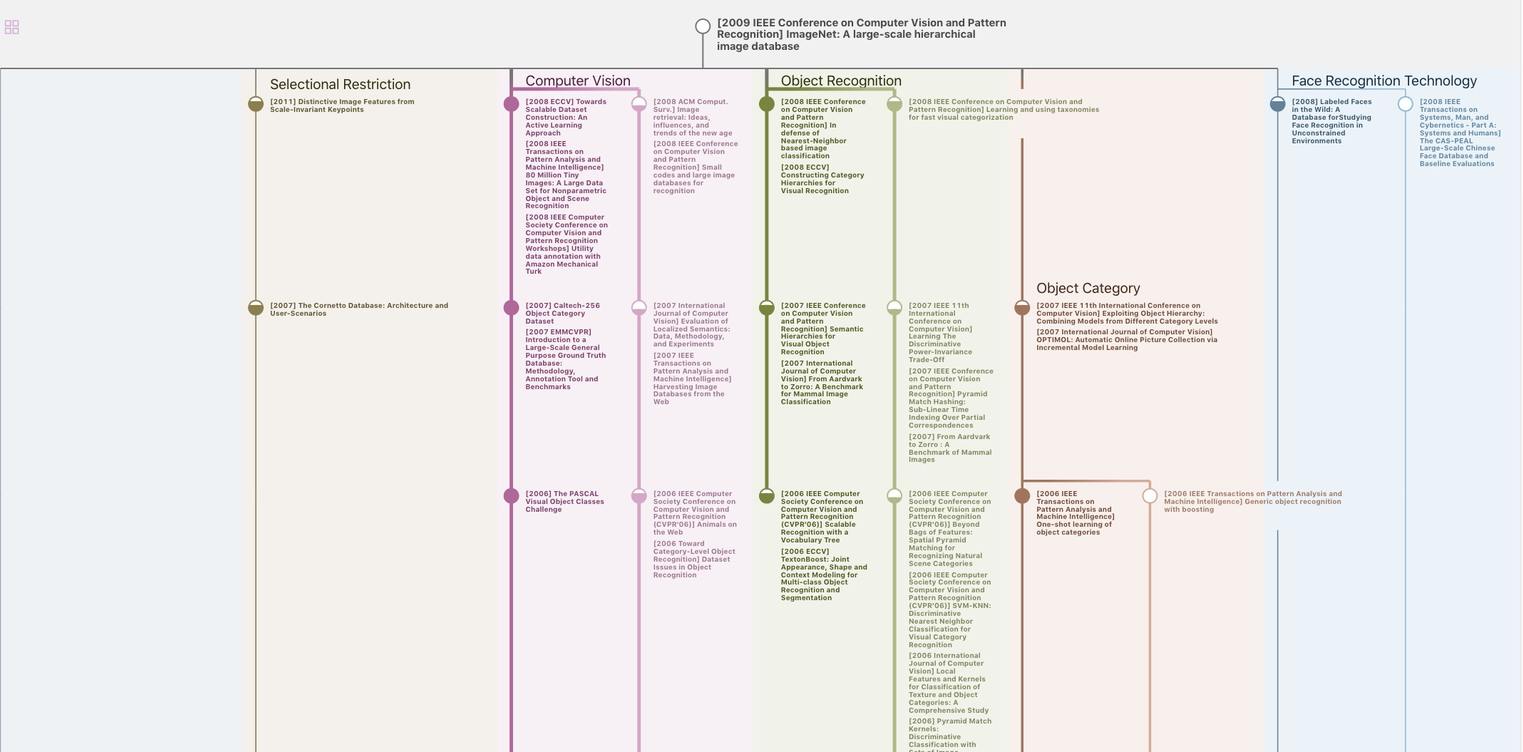
生成溯源树,研究论文发展脉络
Chat Paper
正在生成论文摘要