Multi-Armed Bandits for Autonomous Timing-driven Design Optimization
2019 29th International Symposium on Power and Timing Modeling, Optimization and Simulation (PATMOS)(2019)
摘要
Timing closure is a complex process that involves many iterative optimization steps applied in various phases of the physical design flow. Cell sizing and transistor threshold selection, as well as datapath and clock buffering, are some of the tools available for design optimization. At the moment, design optimization methods are integrated into EDA tools and applied incrementally in various parts of the flow, while the optimal order of their application is yet to be determined. In this work, we rely on reinforcement learning - through the use of the Multi-Armed Bandit model for decision making under uncertainty - to automatically suggest online which optimization heuristic should be applied to the design. The goal is to improve the performance metrics based on the rewards learned from the previous applications of each heuristic. Experimental results show that automating the process of design optimization with machine learning not only results in designs that are close to the best-published results derived from deterministic approaches, but it also allows for the execution of the optimization flow without any human in the loop, and without any need for offline training of the heuristic-orchestration algorithm.
更多查看译文
关键词
autonomous timing-driven design optimization,timing closure,complex process,optimization steps,physical design flow,transistor threshold selection,datapath,clock buffering,design optimization methods,EDA tools,optimal order,multiarmed bandit model,optimization heuristic,optimization flow
AI 理解论文
溯源树
样例
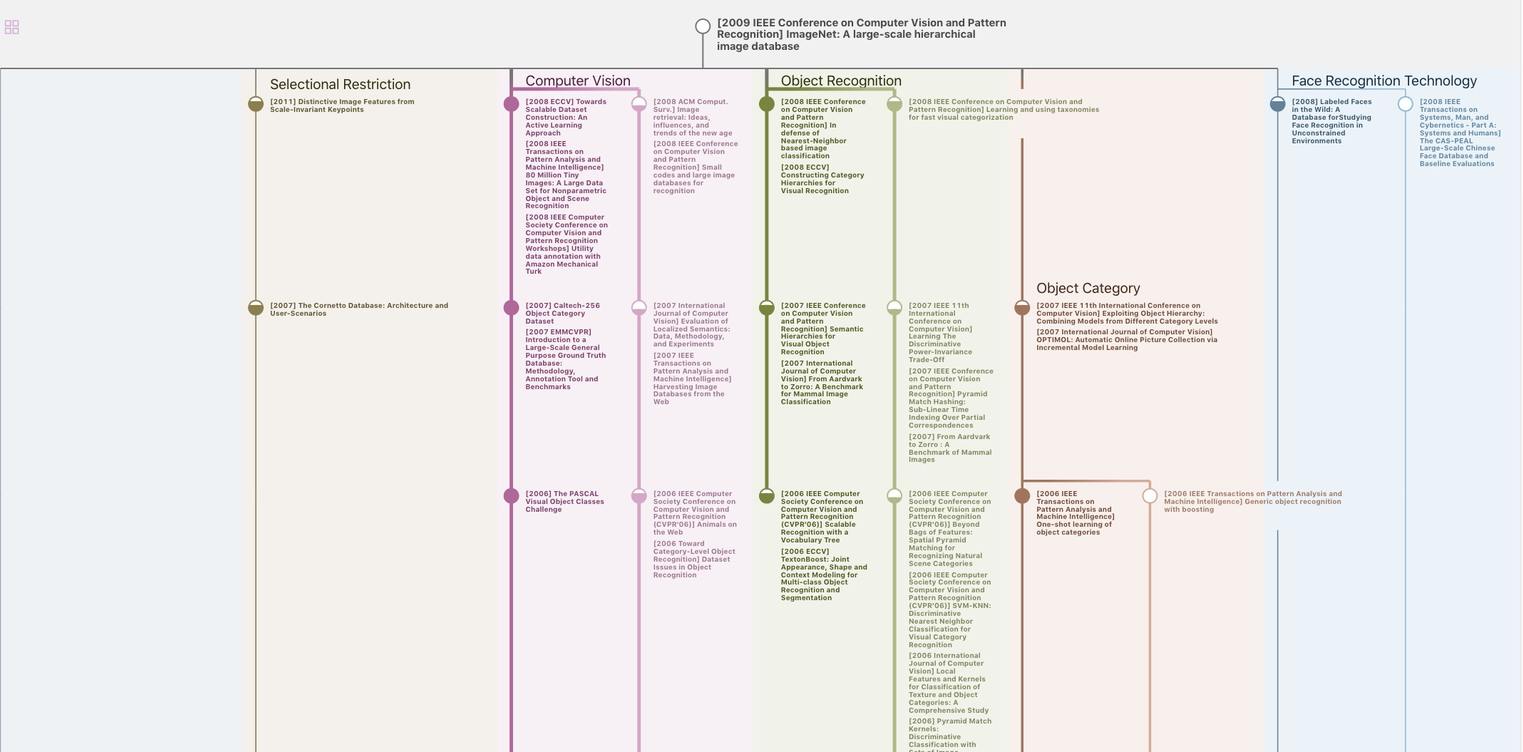
生成溯源树,研究论文发展脉络
Chat Paper
正在生成论文摘要