Evaluating Parameterization Methods for Convolutional Neural Network (CNN)-Based Image Operators
IEEE Computer Society Conference on Computer Vision and Pattern Recognition Workshops(2019)
摘要
Recently, deep neural networks have been widely used to approximate or improve image operators. In general, an image operator has some hyper-parameters that change its operating configurations, e.g., the strength of smoothing, up-scale factors in super-resolution, or a type of image operator. To address varying parameter settings, an image operator taking such parameters as its input, namely a parameterized image operator, is an essential cue in image processing. Since many types of parameterization techniques exist, a comparative analysis is required in the context of image processing. In this paper, we therefore analytically explore the operation principles of these parameterization techniques and study their differences. In addition, performance comparisons between image operators parameterized by using these methods are assessed experimentally on common image processing tasks including image smoothing, denoising, deblocking, and super-resolution.
更多查看译文
关键词
image super-resolution,image deblocking,image denoising,image smoothing,operating configurations,convolutional neural network-based image operators,common image processing tasks,operation principles,parameterized image operator
AI 理解论文
溯源树
样例
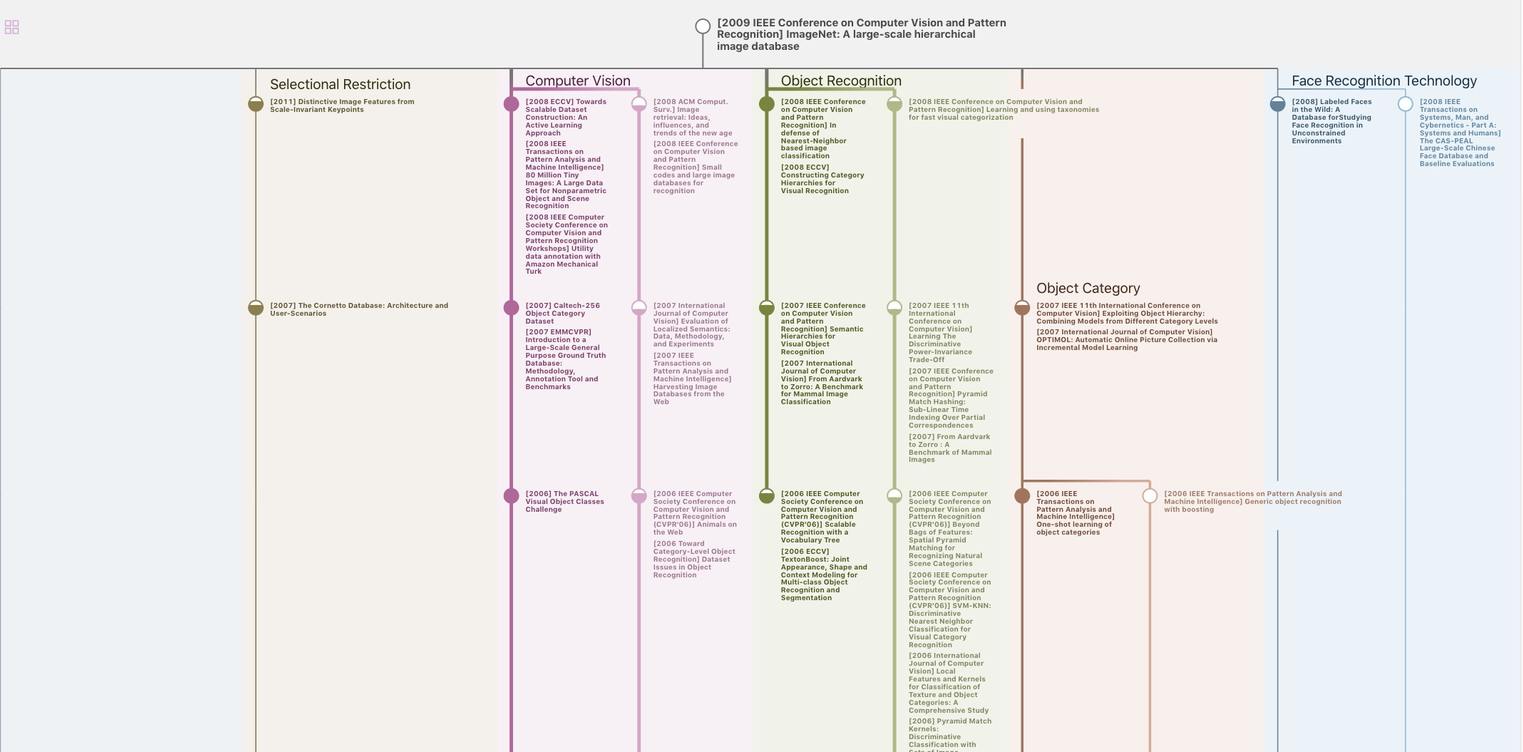
生成溯源树,研究论文发展脉络
Chat Paper
正在生成论文摘要