Challenges in Bayesian inference via Markov chain Monte Carlo for neural networks
arxiv(2021)
摘要
Markov chain Monte Carlo (MCMC) methods and neural networks are instrumental in tackling inferential and prediction problems. However, Bayesian inference based on joint use of MCMC methods and of neural networks is limited. This paper reviews the main challenges posed by neural networks to MCMC developments, including lack of parameter identifiability due to weight symmetries, prior specification effects, and consequently high computational cost and convergence failure. Population and manifold MCMC algorithms are combined to demonstrate these challenges via multilayer perceptron (MLP) examples and to develop case studies for assessing the capacity of approximate inference methods to uncover the posterior covariance of neural network parameters. Some of these challenges, such as high computational cost arising from the application of neural networks to big data and parameter identifiability arising from weight symmetries, stimulate research towards more scalable approximate MCMC methods or towards MCMC methods in reduced parameter spaces.
更多查看译文
关键词
Bayesian inference, Bayesian neural networks, convergence diagnostics, Markov chain Monte Carlo, posterior predictive distribution
AI 理解论文
溯源树
样例
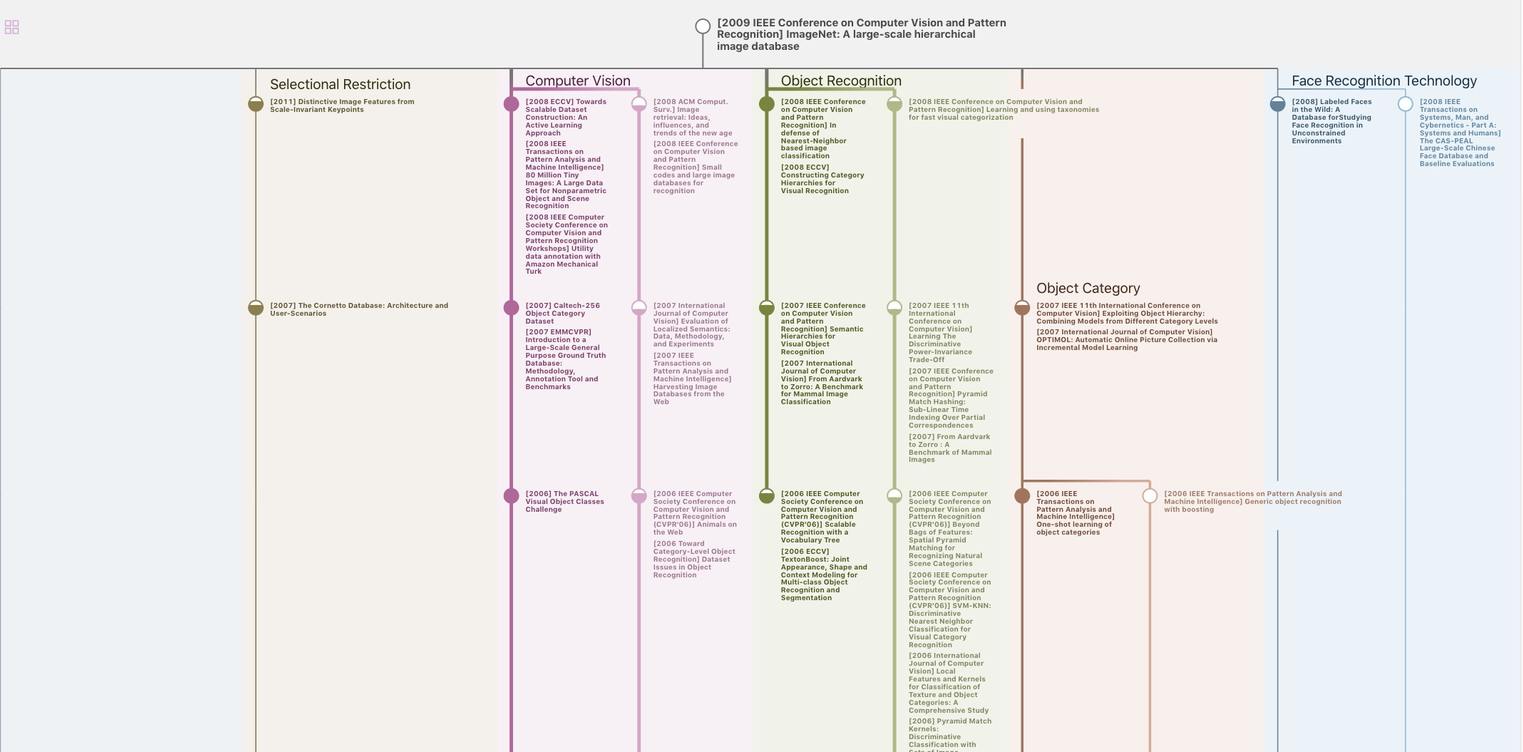
生成溯源树,研究论文发展脉络
Chat Paper
正在生成论文摘要