Improving documentation of presenting problems in the emergency department using a domain-specific ontology and machine learning-driven user interfaces.
International journal of medical informatics(2019)
摘要
OBJECTIVES:To determine the effect of a domain-specific ontology and machine learning-driven user interfaces on the efficiency and quality of documentation of presenting problems (chief complaints) in the emergency department (ED).
METHODS:As part of a quality improvement project, we simultaneously implemented three interventions: a domain-specific ontology, contextual autocomplete, and top five suggestions. Contextual autocomplete is a user interface that ranks concepts by their predicted probability which helps nurses enter data about a patient's presenting problems. Nurses were also given a list of top five suggestions to choose from. These presenting problems were represented using a consensus ontology mapped to SNOMED CT. Predicted probabilities were calculated using a previously derived model based on triage vital signs and a brief free text note. We evaluated the percentage and quality of structured data captured using a mixed methods retrospective before-and-after study design.
RESULTS:A total of 279,231 consecutive patient encounters were analyzed. Structured data capture improved from 26.2% to 97.2% (p < 0.0001). During the post-implementation period, presenting problems were more complete (3.35 vs 3.66; p = 0.0004) and higher in overall quality (3.38 vs. 3.72; p = 0.0002), but showed no difference in precision (3.59 vs. 3.74; p = 0.1). Our system reduced the mean number of keystrokes required to document a presenting problem from 11.6 to 0.6 (p < 0.0001), a 95% improvement.
DISCUSSION:We demonstrated a technique that captures structured data on nearly all patients. We estimate that our system reduces the number of man-hours required annually to type presenting problems at our institution from 92.5 h to 4.8 h.
CONCLUSION:Implementation of a domain-specific ontology and machine learning-driven user interfaces resulted in improved structured data capture, ontology usage compliance, and data quality.
更多查看译文
AI 理解论文
溯源树
样例
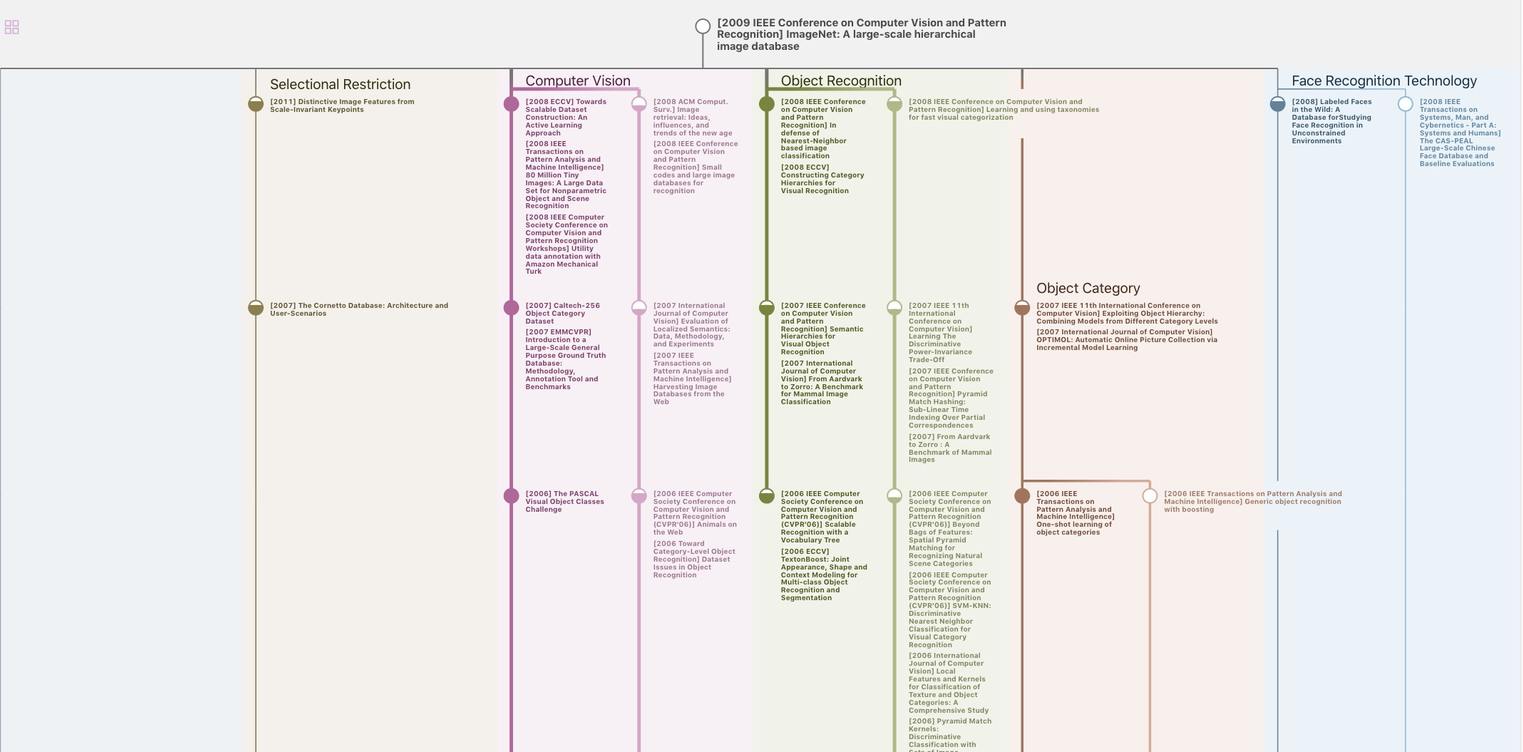
生成溯源树,研究论文发展脉络
Chat Paper
正在生成论文摘要