Bayesian Hierarchical Dynamic Model for Human Action Recognition
2019 IEEE/CVF CONFERENCE ON COMPUTER VISION AND PATTERN RECOGNITION (CVPR 2019)(2019)
摘要
Human action recognition remains as a challenging task partially due to the presence of large variations in the execution of action. To address this issue, we propose a probabilistic model called Hierarchical Dynamic Model (HDM). Leveraging on Bayesian framework, the model parameters are allowed to vary across different sequences of data, which increase the capacity of the model to adapt to intra-class variations on both spatial and temporal extent of actions. Meanwhile, the generative learning process allows the model to preserve the distinctive dynamic pattern for each action class. Through Bayesian inference, we are able to quantify the uncertainty of the classification, providing insight during the decision process. Compared to state-of-the-art methods, our method not only achieves competitive recognition performance within individual dataset but also shows better generalization capability across different datasets. Experiments conducted on data with missing values also show the robustness of the proposed method.
更多查看译文
关键词
Face,Gesture,and Body Pose,Action Recognition,Statistical Learning
AI 理解论文
溯源树
样例
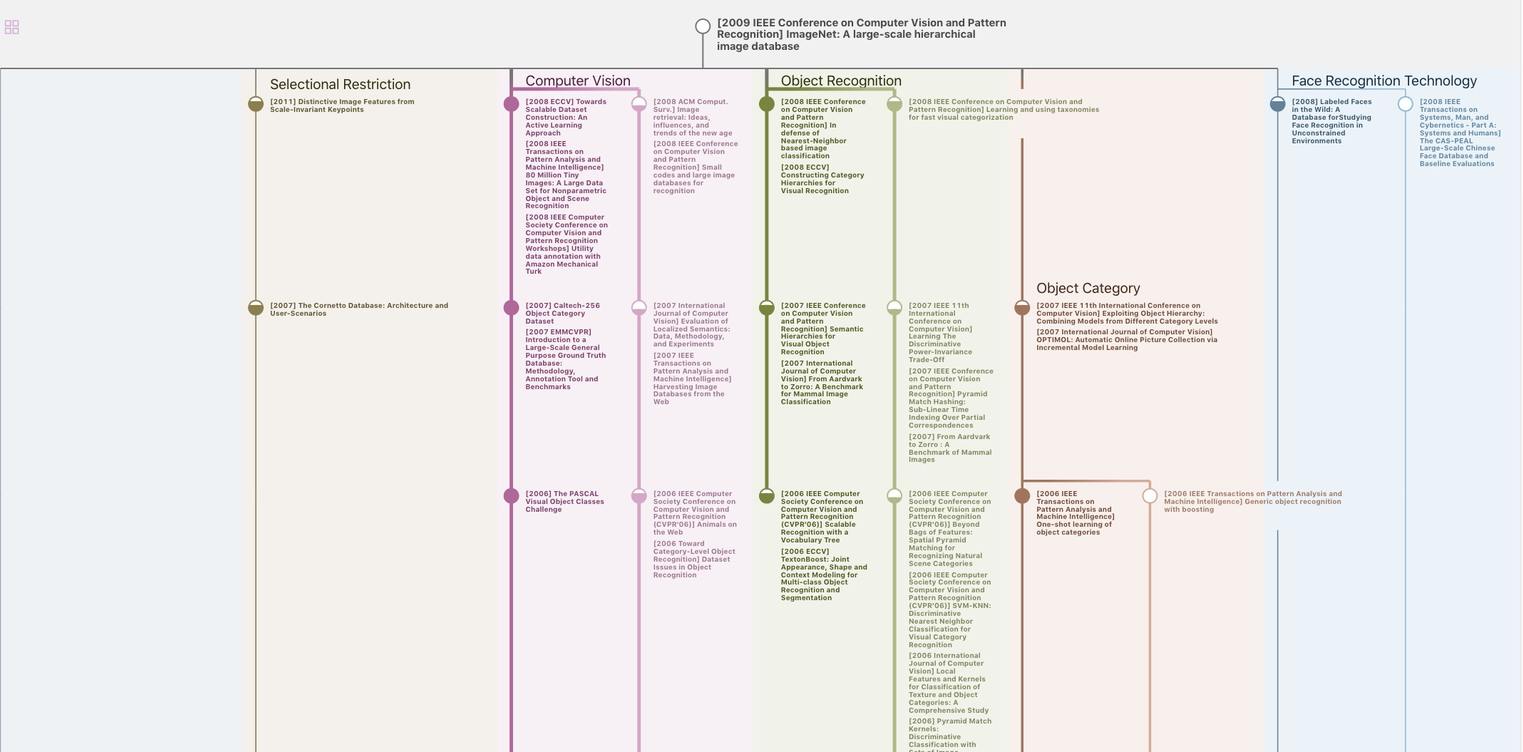
生成溯源树,研究论文发展脉络
Chat Paper
正在生成论文摘要