Patch-Based Discriminative Feature Learning for Unsupervised Person Re-Identification
2019 IEEE/CVF CONFERENCE ON COMPUTER VISION AND PATTERN RECOGNITION (CVPR 2019)(2019)
摘要
While discriminative local features have been shown effective in solving the person re-identification problem, they are limited to be trained on fully pairwise labelled data which is expensive to obtain. In this work, we overcome this problem by proposing a patch-based unsupervised learning framework in order to learn discriminative feature from patches instead of the whole images. The patch-based learning leverages similarity between patches to learn a discriminative model. Specifically, we develop a PatchNet to select patches from the feature map and learn discriminative features for these patches. To provide effective guidance for the PatchNet to learn discriminative patch feature on unlabeled datasets, we propose an unsupervised patch-based discriminative feature learning loss. In addition, we design an image-level feature learning loss to leverage all the patch features of the same image to serve as an image-level guidance for the PatchNet. Extensive experiments validate the superiority of our method for unsupervised person re-id. Our code is available at https://github.com/QizeYang/PAUL.
更多查看译文
关键词
Biometrics,Recognition: Detection,Categorization,Retrieval,Video Analytics
AI 理解论文
溯源树
样例
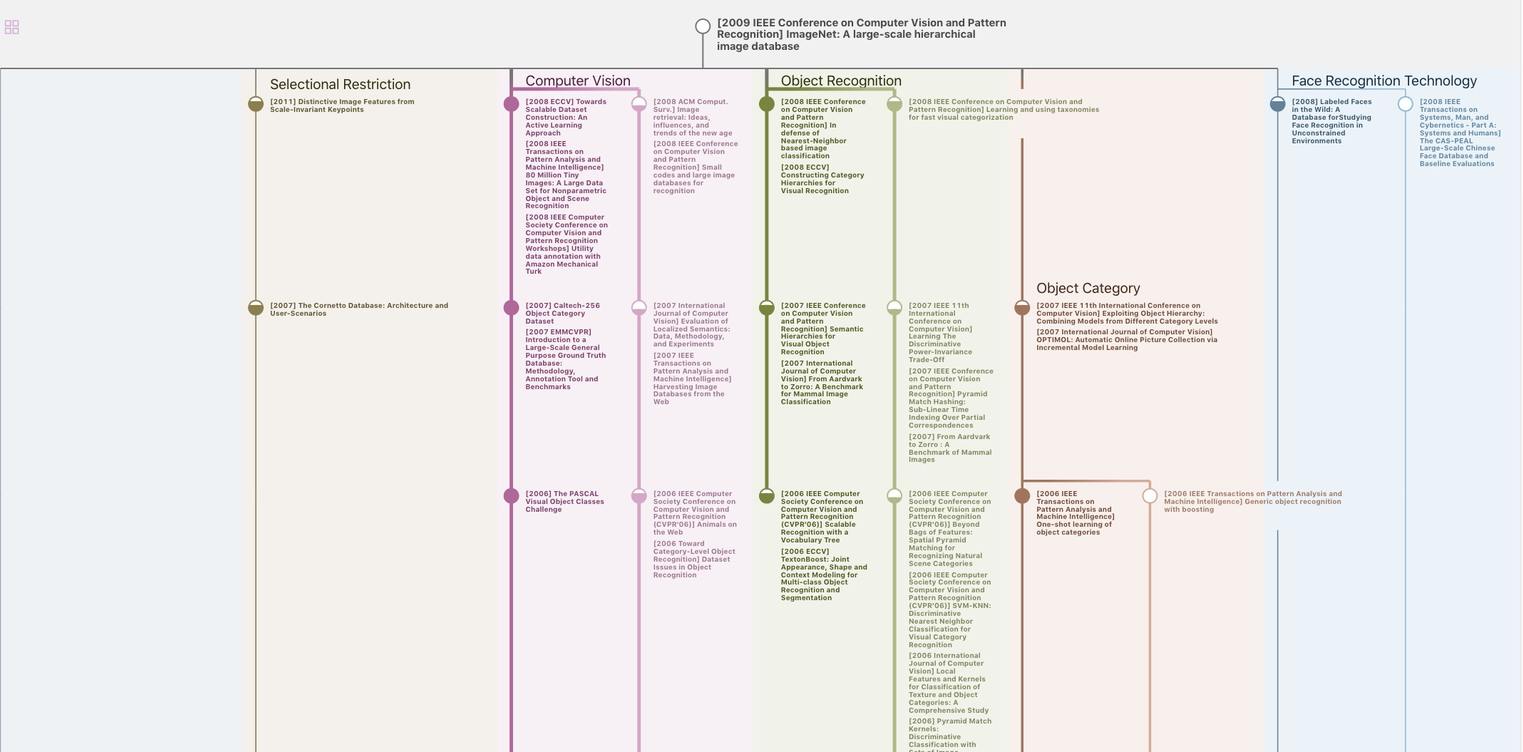
生成溯源树,研究论文发展脉络
Chat Paper
正在生成论文摘要