Hierarchical Deep Stereo Matching On High-Resolution Images
2019 IEEE/CVF CONFERENCE ON COMPUTER VISION AND PATTERN RECOGNITION (CVPR 2019)(2019)
摘要
We explore the problem of real-time stereo matching on high-res imagery. Many state-of-the-art (SOTA) methods struggle to process high-res imagery because of memory constraints or speed limitations. To address this issue, we propose an end-to-end framework that searches for correspondences incrementally over a coarse-to-fine hierarchy. Because high-res stereo datasets are relatively rare, we introduce a dataset with high-res stereo pairs for both training and evaluation. Our approach achieved SOTA performance on Middlebury-v3 and KITTI-15 while running significantly faster than its competitors. The hierarchical design also naturally allows for anytime on-demand reports of disparity by capping intermediate coarse results, allowing us to accurately predict disparity for near-range structures with low latency (30ms). We demonstrate that the performance-vs-speed tradeoff afforded by on-demand hierarchies may address sensing needs for time-critical applications such as autonomous driving.
更多查看译文
关键词
3D from Multiview and Sensors,Big Data,Large Scale Methods,Datasets and Evaluation,Deep Learning,Low-level Vision,Robotics
AI 理解论文
溯源树
样例
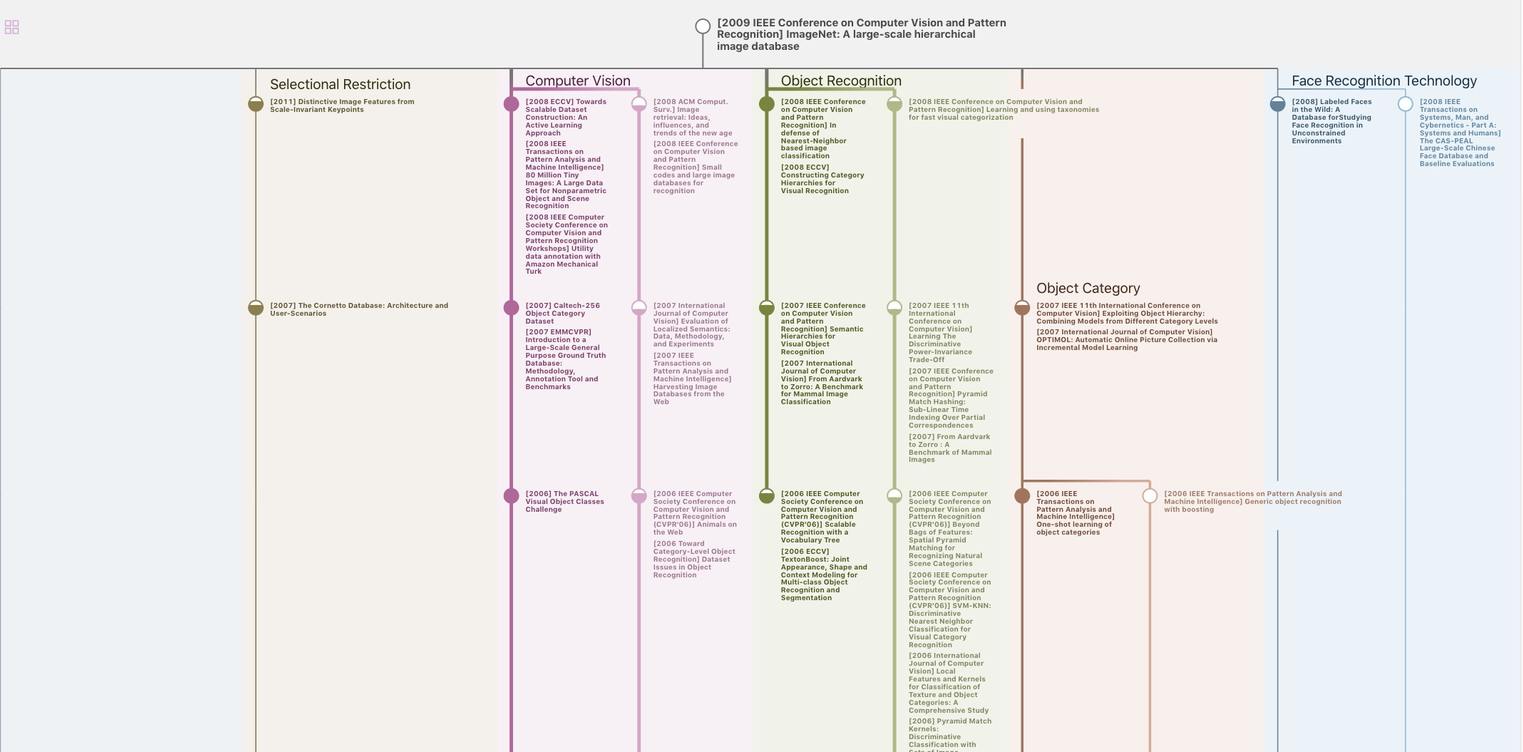
生成溯源树,研究论文发展脉络
Chat Paper
正在生成论文摘要