Deep Global Generalized Gaussian Networks
2019 IEEE/CVF CONFERENCE ON COMPUTER VISION AND PATTERN RECOGNITION (CVPR 2019)(2019)
摘要
Recently, global covariance pooling (GCP) has shown great advance in improving classification performance of deep convolutional neural networks (CNNs). However, existing deep GCP networks compute covariance pooling of convolutional activations with assumption that activations are sampled from Gaussian distributions, which may not hold in practice and fails to fully characterize the statistics of activations. To handle this issue, this paper proposes a novel deep global generalized Gaussian network (3G-Net), whose core is to estimate a global covariance of generalized Gaussian for modeling the last convolutional activations. Compared with GCP in Gaussian setting, our 3G-Net assumes the distribution of activations follows a generalized Gaussian, which can capture more precise characteristics of activations. However, there exists no analytic solution for parameter estimation of generalized Gaussian, making our 3G-Net challenging. To this end, we first present a novel regularized maximum likelihood estimator for robust estimating covariance of generalized Gaussian, which can be optimized by a modified iterative re-weighted method. Then, to efficiently estimate the covariance of generaized Gaussian under deep CNN architectures, we approximate this re-weighted method by developing an unrolling re-weighted module and a square root covariance layer. In this way, 3GNet can be flexibly trained in an end-to-end manner. The experiments are conducted on large-scale ImageNet-1K and Places365 datasets, and the results demonstrate our 3G-Net outperforms its counterparts while achieving very competitive performance to state-of-the-arts.
更多查看译文
关键词
Recognition: Detection,Categorization,Retrieval,Deep Learning , Statistical Learning
AI 理解论文
溯源树
样例
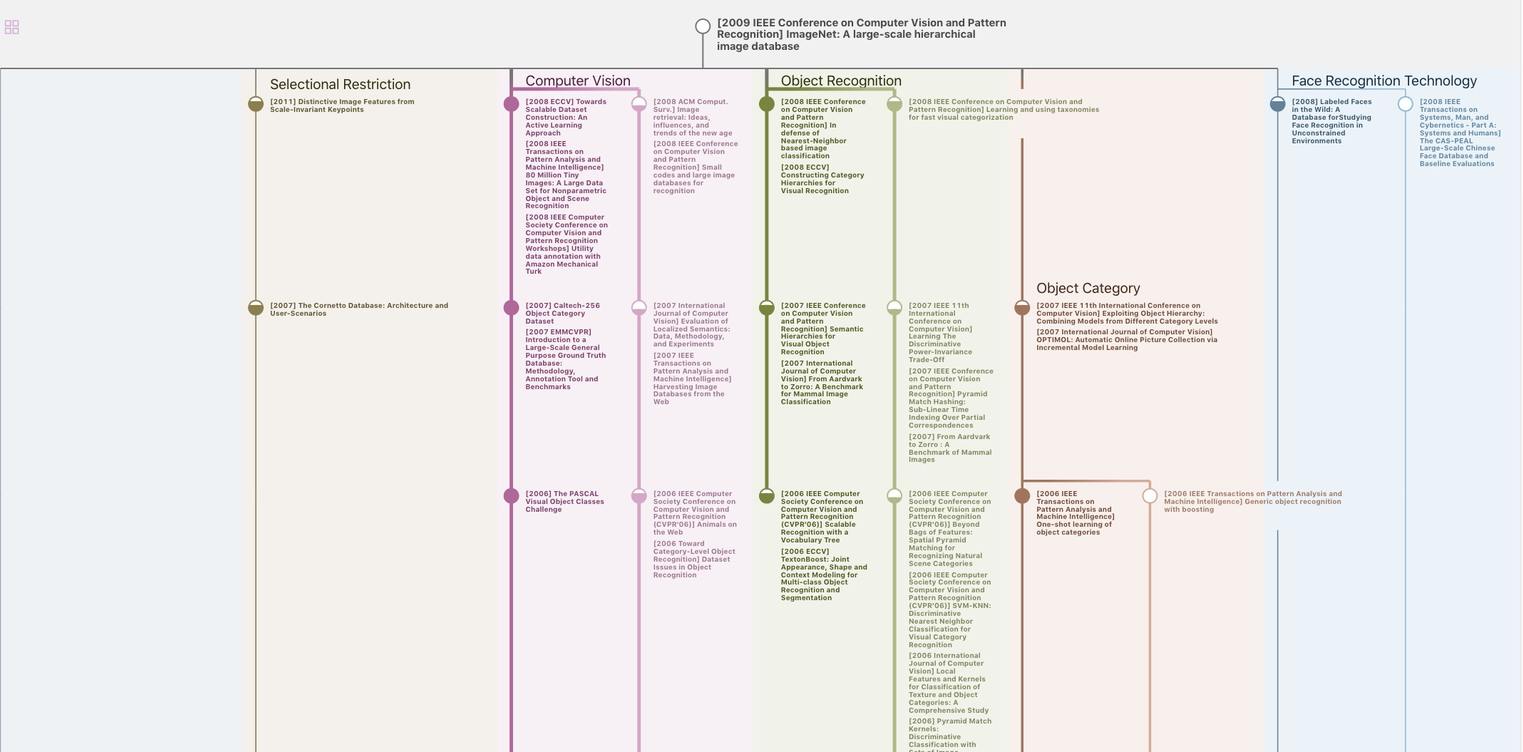
生成溯源树,研究论文发展脉络
Chat Paper
正在生成论文摘要