Large-Scale Few-Shot Learning: Knowledge Transfer With Class Hierarchy
2019 IEEE/CVF CONFERENCE ON COMPUTER VISION AND PATTERN RECOGNITION (CVPR 2019)(2019)
摘要
Recently, large-scale few-shot learning (FSL) becomes topical. It is discovered that, for a large-scale FSL problem with 1,000 classes in the source domain, a strong baseline emerges, that is, simply training a deep feature embedding model using the aggregated source classes and performing nearest neighbor (NN) search using the learned features on the target classes. The state-of-the-art large-scale FSL methods struggle to beat this baseline, indicating intrinsic limitations on scalability. To overcome the challenge, we propose a novel large-scale FSL model by learning transferable visual features with the class hierarchy which encodes the semantic relations between source and target classes. Extensive experiments show that the proposed model significantly outperforms not only the NN baseline but also the state-of-the-art alternatives. Furthermore, we show that the proposed model can be easily extended to the large-scale zero-shot learning (ZSL) problem and also achieves the state-of-the-art results.
更多查看译文
关键词
Recognition: Detection,Categorization,Retrieval,Big Data,Large Scale Methods
AI 理解论文
溯源树
样例
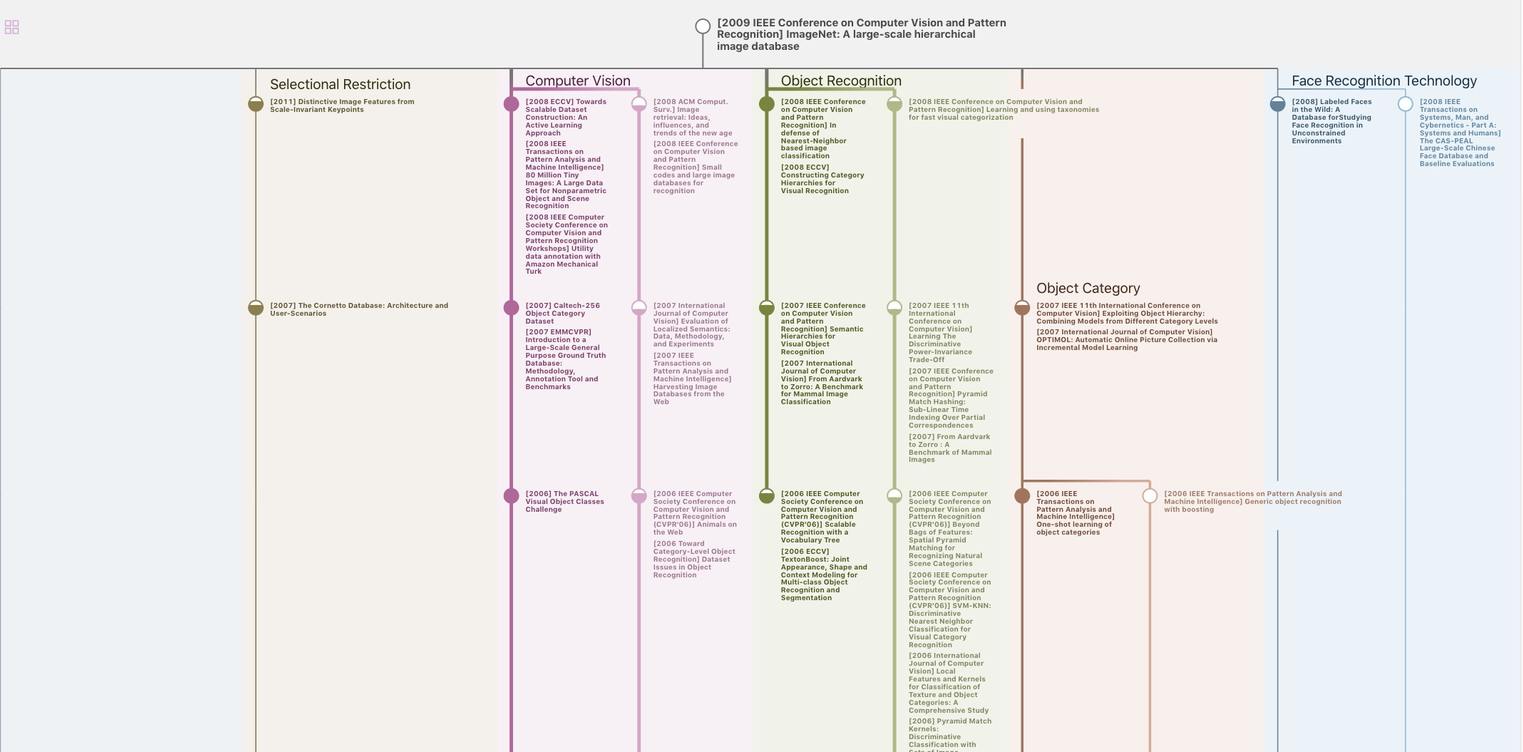
生成溯源树,研究论文发展脉络
Chat Paper
正在生成论文摘要