Sensitive-Sample Fingerprinting Of Deep Neural Networks
2019 IEEE/CVF CONFERENCE ON COMPUTER VISION AND PATTERN RECOGNITION (CVPR 2019)(2019)
摘要
Numerous cloud-based services are provided to help customers develop and deploy deep learning applications. When a customer deploys a deep learning model in the cloud and serves it to end-users, it is important to be able to verify that the deployed model has not been tampered with.In this paper; we propose a novel and practical methodology to verify the integrity of remote deep learning models, with only black-box access to the target models. Specifically, we define Sensitive-Sample fingerprints, which are a small set of human unnoticeable transformed inputs that make the model outputs sensitive to the model's parameters. Even small model changes can be clearly reflected in the model outputs. Experimental results on different types of model integrity attacks show that the proposed approach is both effective and efficient. It can detect model integrity breaches with high accuracy (>99.95%) and guaranteed zero false positives on all evaluated attacks. Meanwhile, it only requires up to 103x fewer model inferences, compared to non-sensitive samples.
更多查看译文
关键词
Deep Learning,Others
AI 理解论文
溯源树
样例
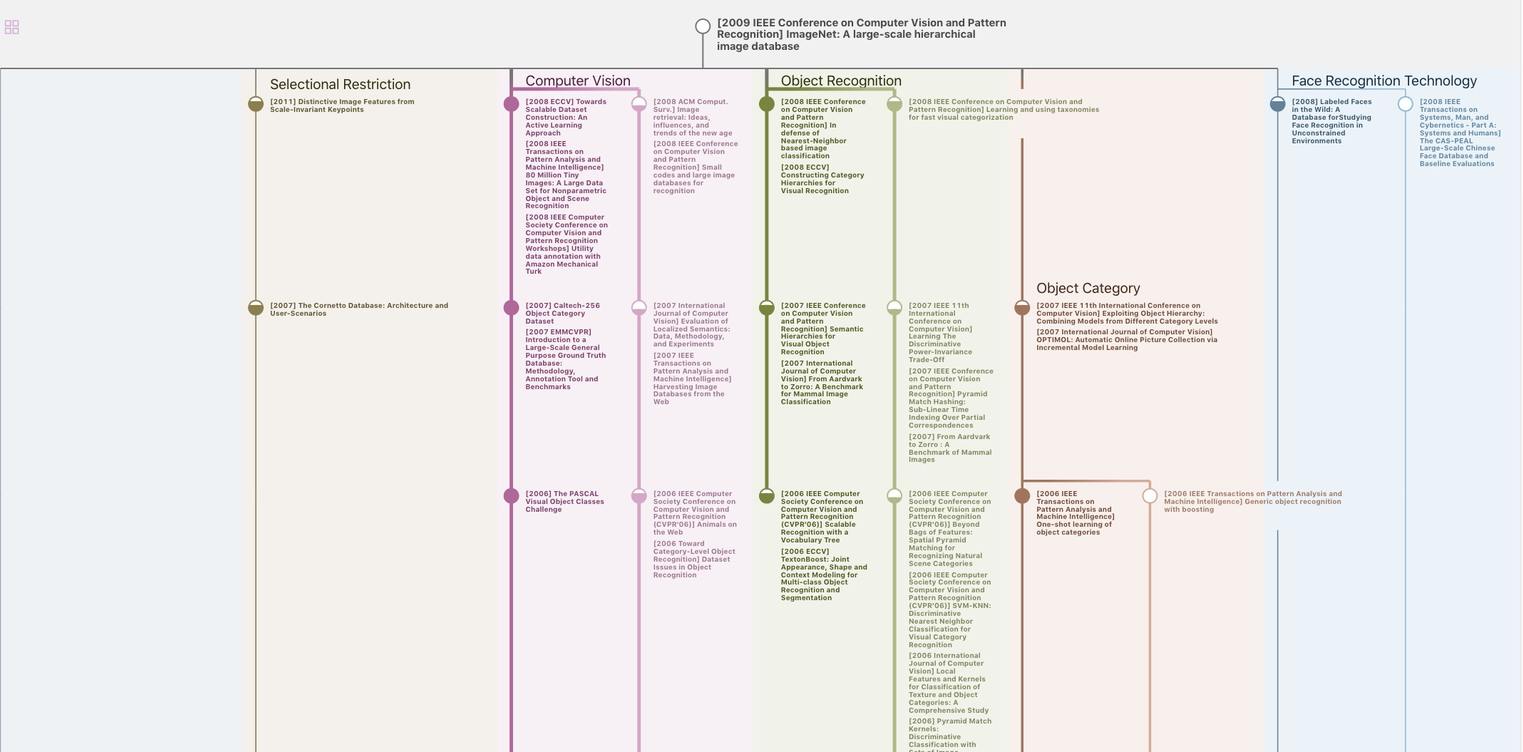
生成溯源树,研究论文发展脉络
Chat Paper
正在生成论文摘要