Second-Order Attention Network For Single Image Super-Resolution
2019 IEEE/CVF CONFERENCE ON COMPUTER VISION AND PATTERN RECOGNITION (CVPR 2019)(2019)
摘要
Recently, deep convolutional neural networks (CNNs) have been widely explored in single image super-resolution (SISR) and obtained remarkable performance. However, most of the existing CNN-based SISR methods mainly focus on wider or deeper architecture design, neglecting to explore the feature correlations of intermediate layers, hence hindering the representational power of CNNs. To address this issue, in this paper, we propose a second-order attention network (SAN) for more powerful feature expression and feature correlation learning. Specifically, a novel trainable second-order channel attention (SOCA) module is developed to adaptively rescale the channel-wise features by using second-order feature statistics for more discriminative representations. Furthermore, we present a non-locally enhanced residual group (NLRG) structure, which not only incorporates non-local operations to capture long-distance spatial contextual information, but also contains repeated local-source residual attention groups (LSRAG) to learn increasingly abstract feature representations. Experimental results demonstrate the superiority of our SAN network over state-of-the-art SISR methods in terms of both quantitative metrics and visual quality.
更多查看译文
关键词
Low-level Vision,Deep Learning
AI 理解论文
溯源树
样例
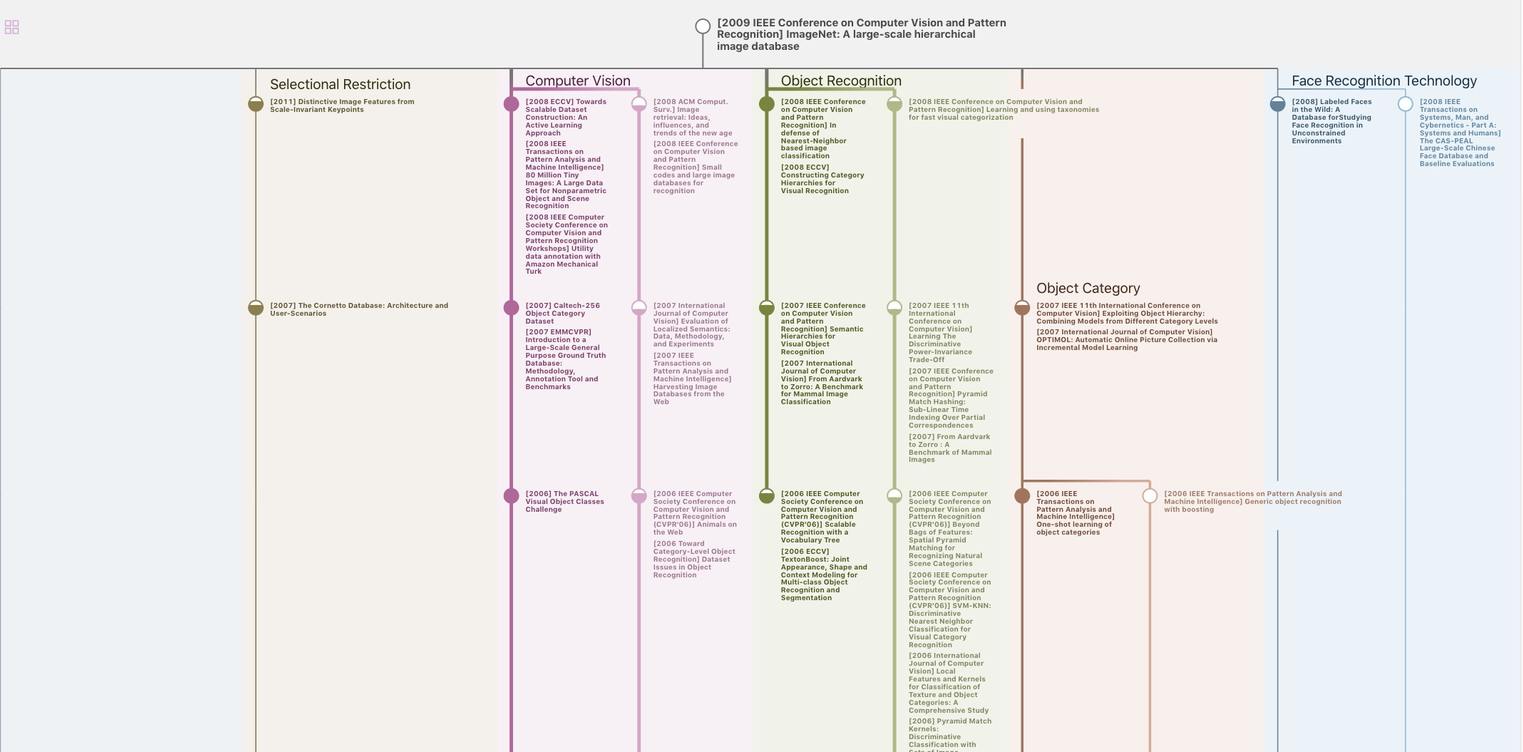
生成溯源树,研究论文发展脉络
Chat Paper
正在生成论文摘要