Hybrid descriptors and Weighted PCA-EFMNet for Face Verification in the Wild
International Journal of Multimedia Information Retrieval(2019)
摘要
Unconstrained face recognition is a complex task because of the numerous intra-class changes caused by lighting, occlusion, facial expressions and poses changes. Such variations affect considerably the performance of facial recognition systems, particularly those based on 2D information. In this research work, an efficient feature extraction method, called Gabor Local Binarized Statistical Image Features, is introduced. A new deep learning network is also developed to extract features relying on data processing components: (1) Cascaded Weighted Principal Component Analysis (WPCA) with Enhanced Fisher Model (WPCA-EFM); (2) binary hashing; and (3) Block-wise Histograms. The suggested architecture (Weighted PCA-EFM) is employed to learn multistage filter banks. Simple block histogram and binary hashing are applied for indexing and pooling, which facilitate the design and learning of our architecture for Face Verification in the Wild. Classification is finally performed using distance measure Cosine and support vector machine. Our experiments are carried out in real-world dataset: Labeled Faces in the Wild. Experimental findings demonstrate that the developed technique achieved high accuracy of 95%.
更多查看译文
关键词
Descriptor,Deep learning,Classification,Fusion,Pooling
AI 理解论文
溯源树
样例
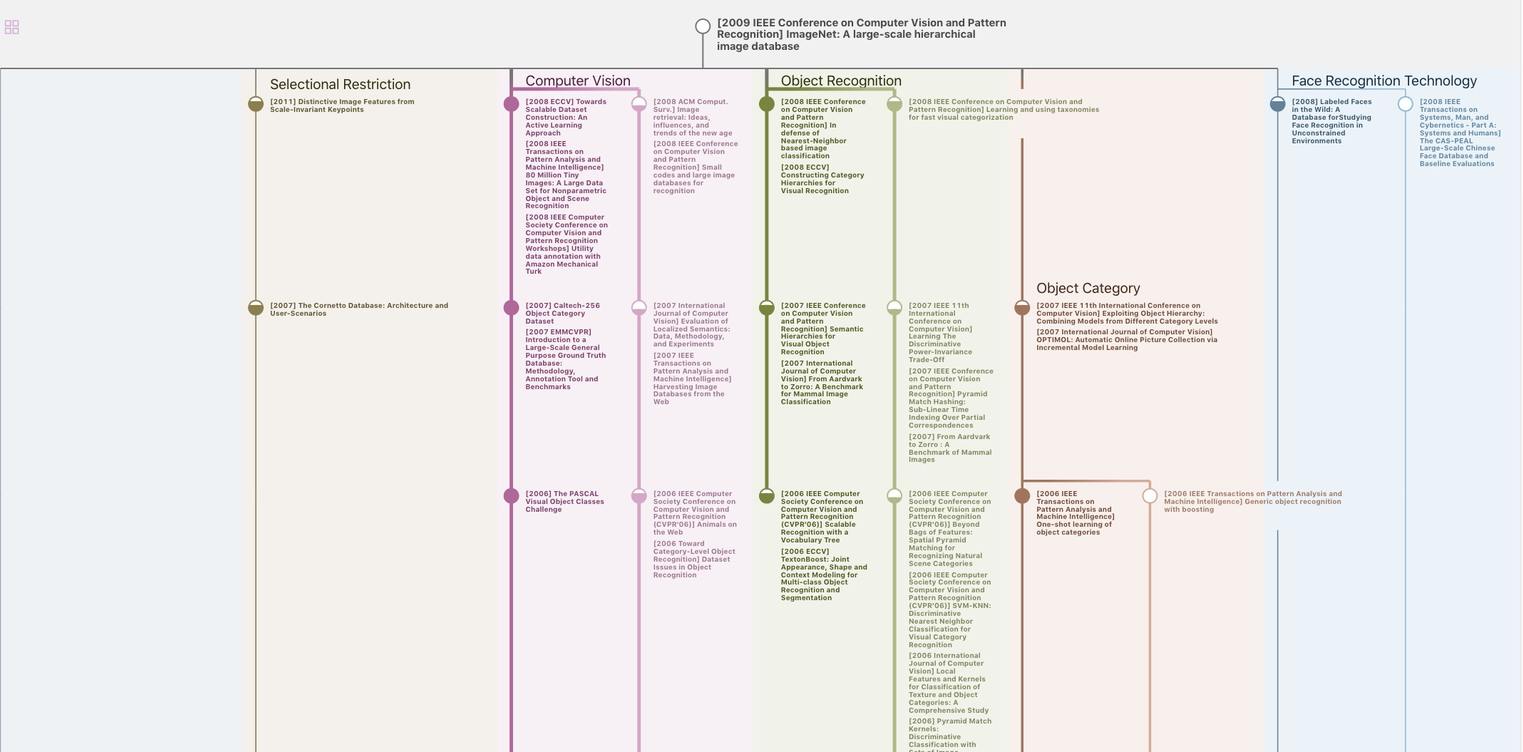
生成溯源树,研究论文发展脉络
Chat Paper
正在生成论文摘要