Deep reinforcement learning-based beam Hopping algorithm in multibeam satellite systems
IET Communications(2019)
摘要
Beam hopping (BH) is the key technology to improve the system throughput and decrease the transmission delay in multibeam satellite systems. The objective of this study is to find a policy to maximise the expected long-term resource utilisation. The BH illumination plan (BHIP) optimisation problem aimed at minimising the transmission delay is formulated and modelled as a partially observable Markov decision process. To tackle the issue of unknown dynamics and prohibitive computation, an artificial intelligence method named deep reinforcement learning (DRL) is first proposed to solve the BHIP problem in multibeam satellite systems. The proposed DRL-BHIP algorithm considers a series of realistic conditions, including the traffic demands in spatial distribution and temporal variation, ModCod constraints, antenna radiation pattern and inter-beam interference. The state reformulation concept is adopted to characterise the traffic spatial and temporal features. Simulation results show that the proposed DRL-BHIP algorithm can decrease the transmission delay and improve the system throughput compared with existing algorithms.
更多查看译文
关键词
Markov processes,learning (artificial intelligence),optimisation,antenna radiation patterns,satellite antennas,telecommunication computing,telecommunication traffic,radiofrequency interference
AI 理解论文
溯源树
样例
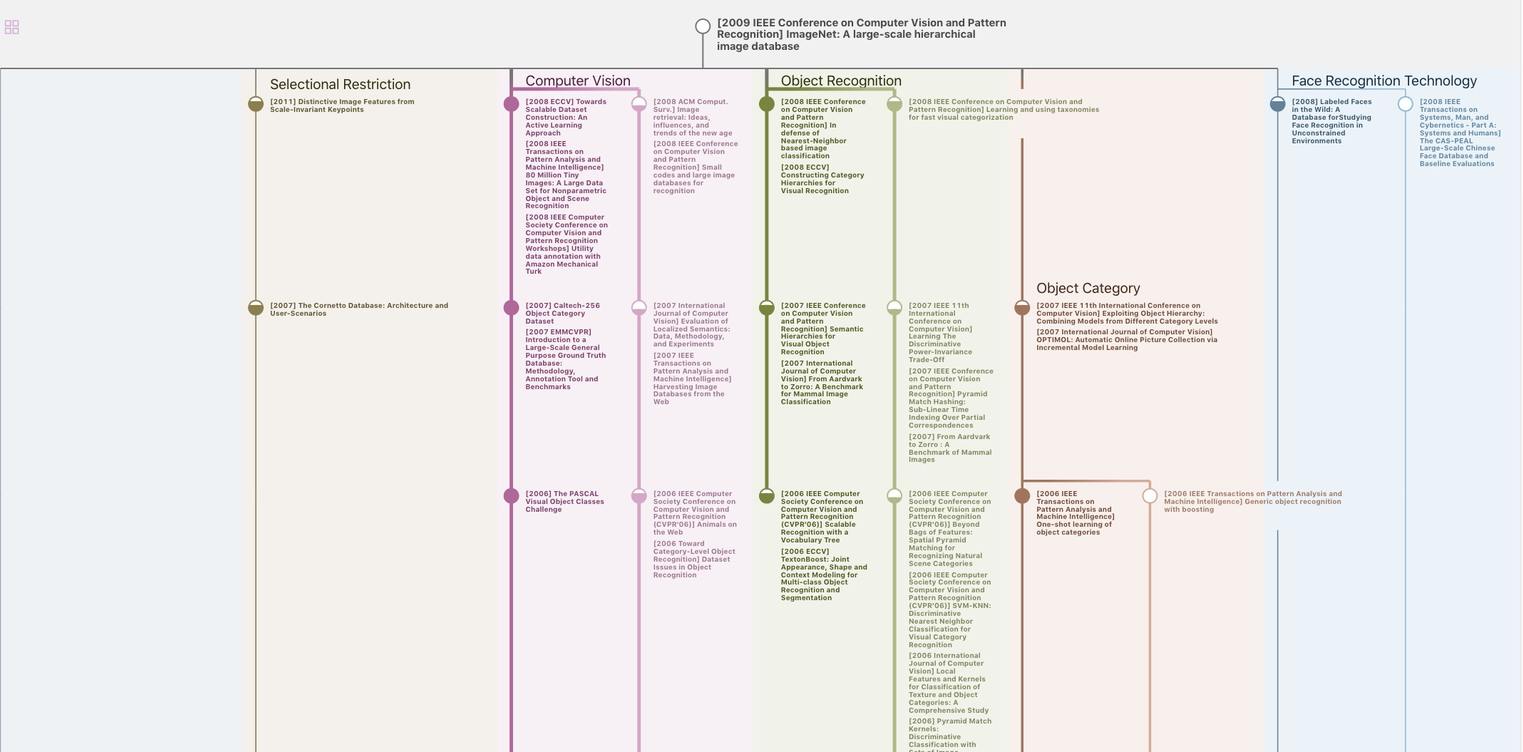
生成溯源树,研究论文发展脉络
Chat Paper
正在生成论文摘要