CASCADE: Connecting RRAMs to Extend Analog Dataflow In An End-To-End In-Memory Processing Paradigm
Proceedings of the 52nd Annual IEEE/ACM International Symposium on Microarchitecture(2019)
摘要
Processing in memory (PIM) is a concept to enable massively parallel dot products while keeping one set of operands in memory. PIM is ideal for computationally demanding deep neural networks (DNNs) and recurrent neural networks (RNNs). Processing in resistive RAM (RRAM) is particularly appealing due to RRAM's high density and low energy. A key limitation of PIM is the cost of multibit analog-to-digital (A/D) conversions that can defeat the efficiency and performance benefits of PIM. In this work, we demonstrate the CASCADE architecture that connects multiply-accumulate (MAC) RRAM arrays with buffer RRAM arrays to extend the processing in analog and in memory: dot products are followed by partial-sum buffering and accumulation to implement a complete DNN or RNN layer. Design choices are made and the interface is designed to enable a variation-tolerant, robust analog dataflow. A new memory mapping scheme named R-Mapping is devised to enable the in-RRAM accumulation of partial sums; and an analog summation scheme is used to reduce the number of A/D conversions required to obtain the final sum. CASCADE is compared with recent in-RRAM computation architectures using state-of-the-art DNN and RNN benchmarks. The results demonstrate that CASCADE improves the energy efficiency by 3.5× while maintaining a competitive throughput.
更多查看译文
关键词
Neural network accelerator, Process in memory, Resistive RAM
AI 理解论文
溯源树
样例
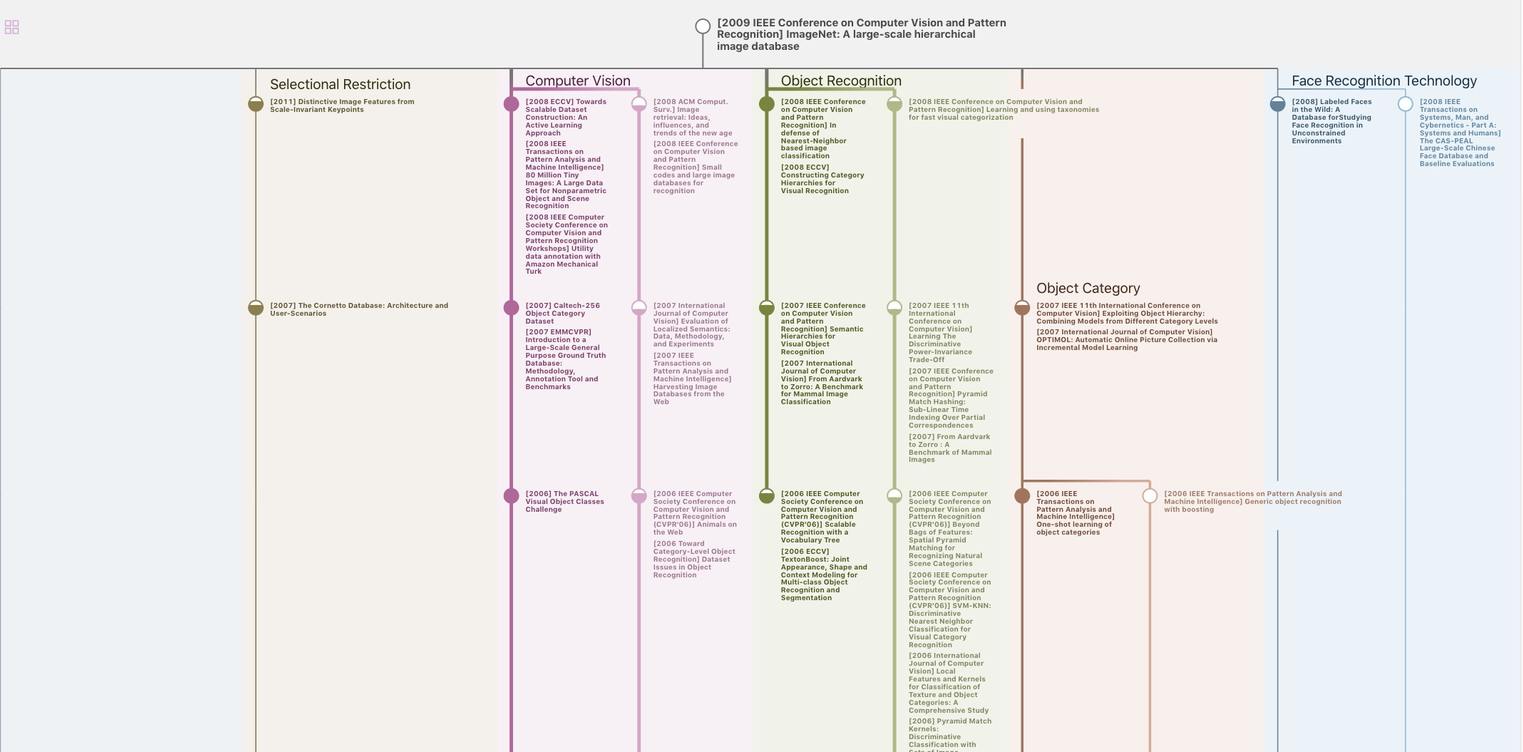
生成溯源树,研究论文发展脉络
Chat Paper
正在生成论文摘要