Robust Visual Tracking based on Adversarial Unlabeled Instance Generation with Label Smoothing Loss Regularization.
Pattern Recognition(2020)
摘要
•We propose two types of generative adversarial networks (GANs) to augment training data in the sample space and feature space respectively, which can capture a variety of appearance changes and bridge the gap between data hunger deep neural networks and visual tracking task.•We propose a label smoothing loss regularization to integrate unlabeled GAN-generated data with real labeled training data for classifier training, which introduces more color, lighting and pose variances to regularize the model and avoid model overfitting.•We conservatively learn a reliable re-detection correlation filter, which is not only combined with classification score metric to evaluate tracking results, but also used to recover tracking failures.•We extensively validate our method on five large-scale benchmark datasets: OTB-2013, OTB-100, UAV123, UAV20L, and VOT2016. Extensive experimental results demonstrate that the proposed tracking algorithm performs favorably against the state-of-the-art trackers.
更多查看译文
关键词
Visual tracking,Sample-level generative adversarial network,Feature-level generative adversarial network,Label smoothing loss regularization,Re-detection correlation filter
AI 理解论文
溯源树
样例
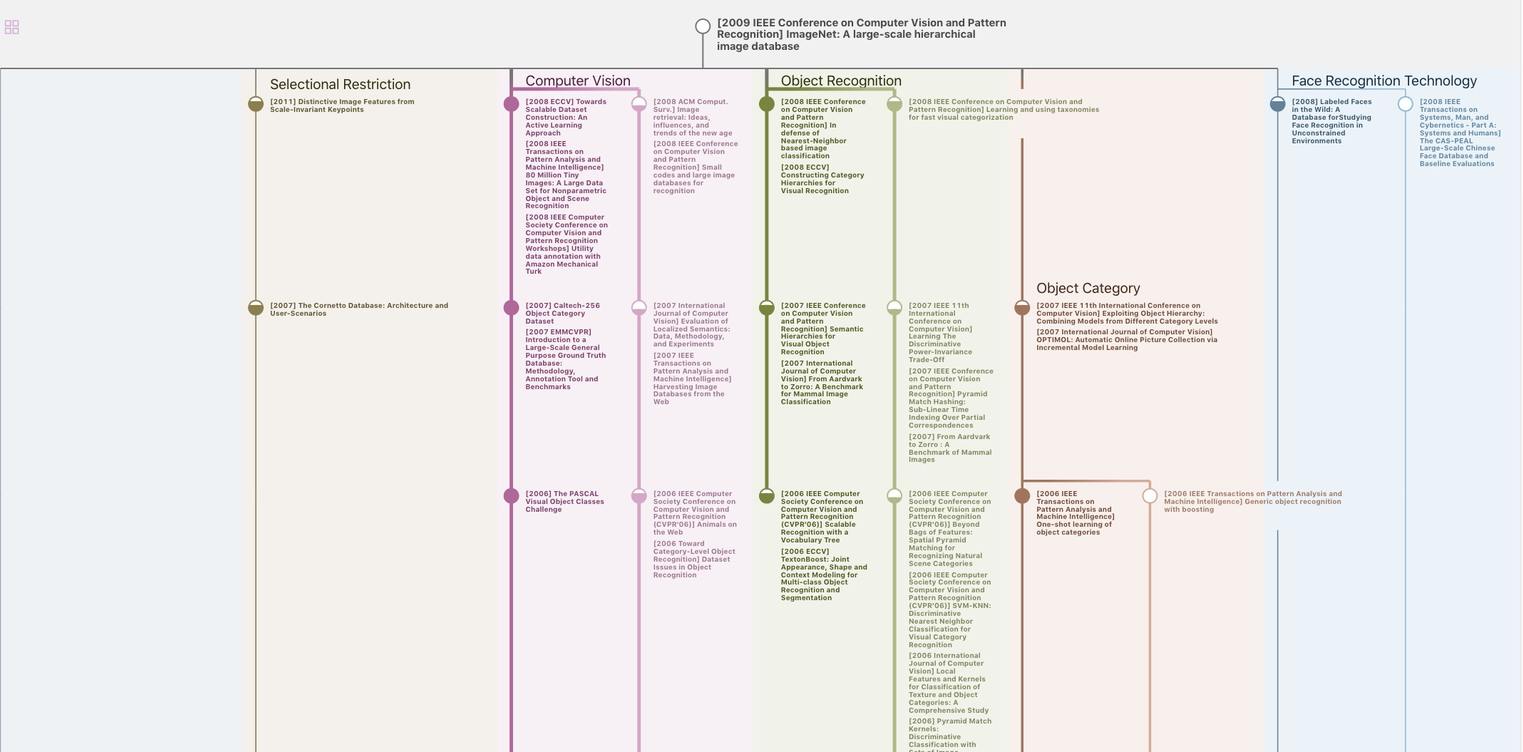
生成溯源树,研究论文发展脉络
Chat Paper
正在生成论文摘要