Saliency detection via multi-level integration and multi-scale fusion neural networks.
Neurocomputing(2019)
摘要
The recent advance on saliency models has remarkably improved performance due to the pervasive application of deep convolutional neural networks. However, for more challenging images, it is worthwhile to explore in deep convolutional neural networks how to effectively exploit features at different levels and scales for saliency detection. In this paper, we propose an end-to-end multi-level feature integration and multi-scale feature fusion network to better predict salient objects in challenging images. Specifically, our network first integrates multi-level features from high-level to low-level features in the network based on ResNet. Then, the feature combined by the multi-level feature integration network is further refined by four parallel residual connected blocks with dilated convolution, in which each block has a specific dilation rate to capture multi-scale context information. Finally, we fuse the outputs of residual connected blocks with dilated convolution and obtain the saliency map by up-sampling operation. Extensive experimental results demonstrate that the proposed model outperforms the state-of-the-art saliency models on several challenging image datasets.
更多查看译文
关键词
Saliency detection,Convolutional neural network,Multi-level integration,Multi-scale fusion
AI 理解论文
溯源树
样例
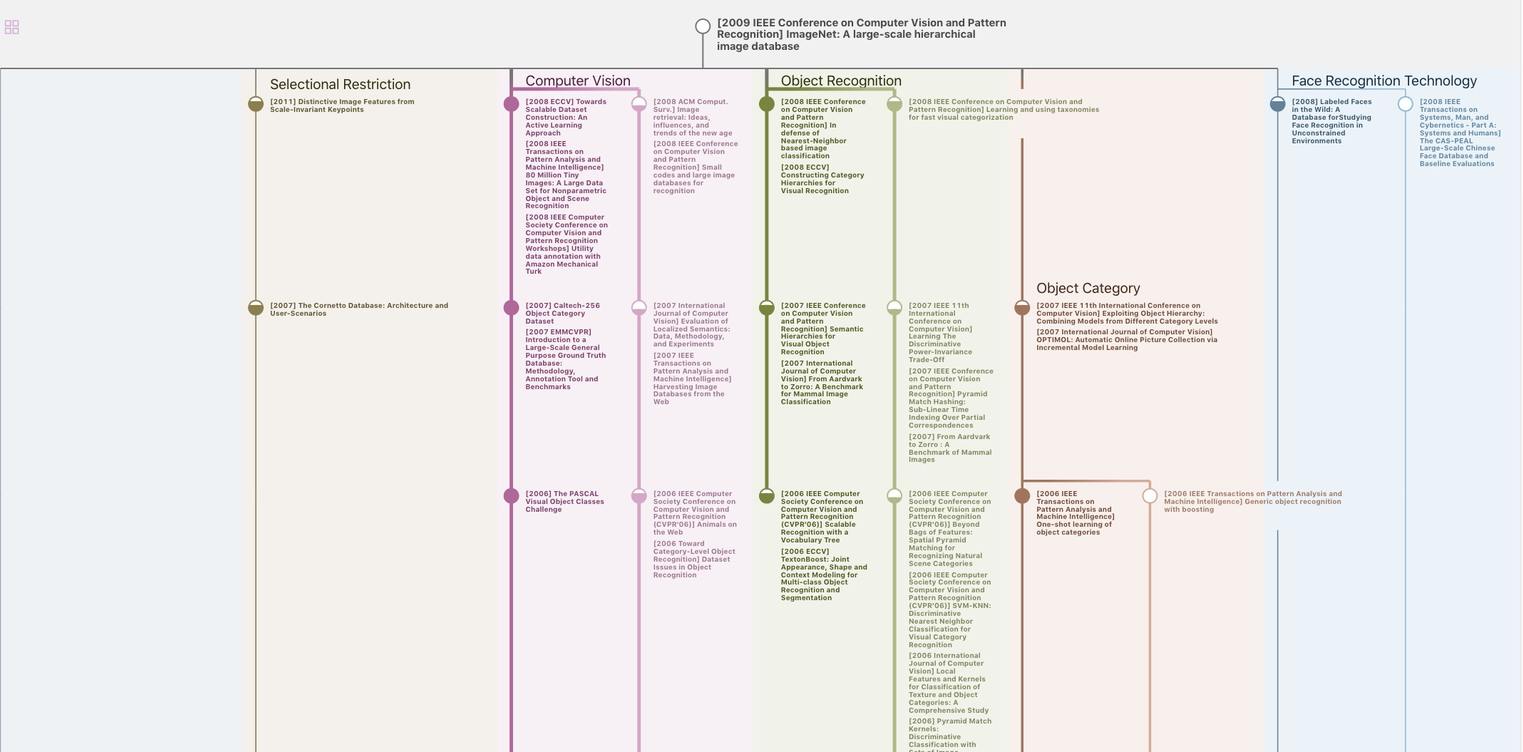
生成溯源树,研究论文发展脉络
Chat Paper
正在生成论文摘要