Linear-Quadratic Mean-Field Reinforcement Learning: Convergence of Policy Gradient Methods
arxiv(2019)
摘要
We investigate reinforcement learning for mean field control problems in discrete time, which can be viewed as Markov decision processes for a large number of exchangeable agents interacting in a mean field manner. Such problems arise, for instance when a large number of robots communicate through a central unit dispatching the optimal policy computed by minimizing the overall social cost. An approximate solution is obtained by learning the optimal policy of a generic agent interacting with the statistical distribution of the states of the other agents. We prove rigorously the convergence of exact and model-free policy gradient methods in a mean-field linear-quadratic setting. We also provide graphical evidence of the convergence based on implementations of our algorithms.
更多查看译文
关键词
reinforcement learning,linear-quadratic,mean-field
AI 理解论文
溯源树
样例
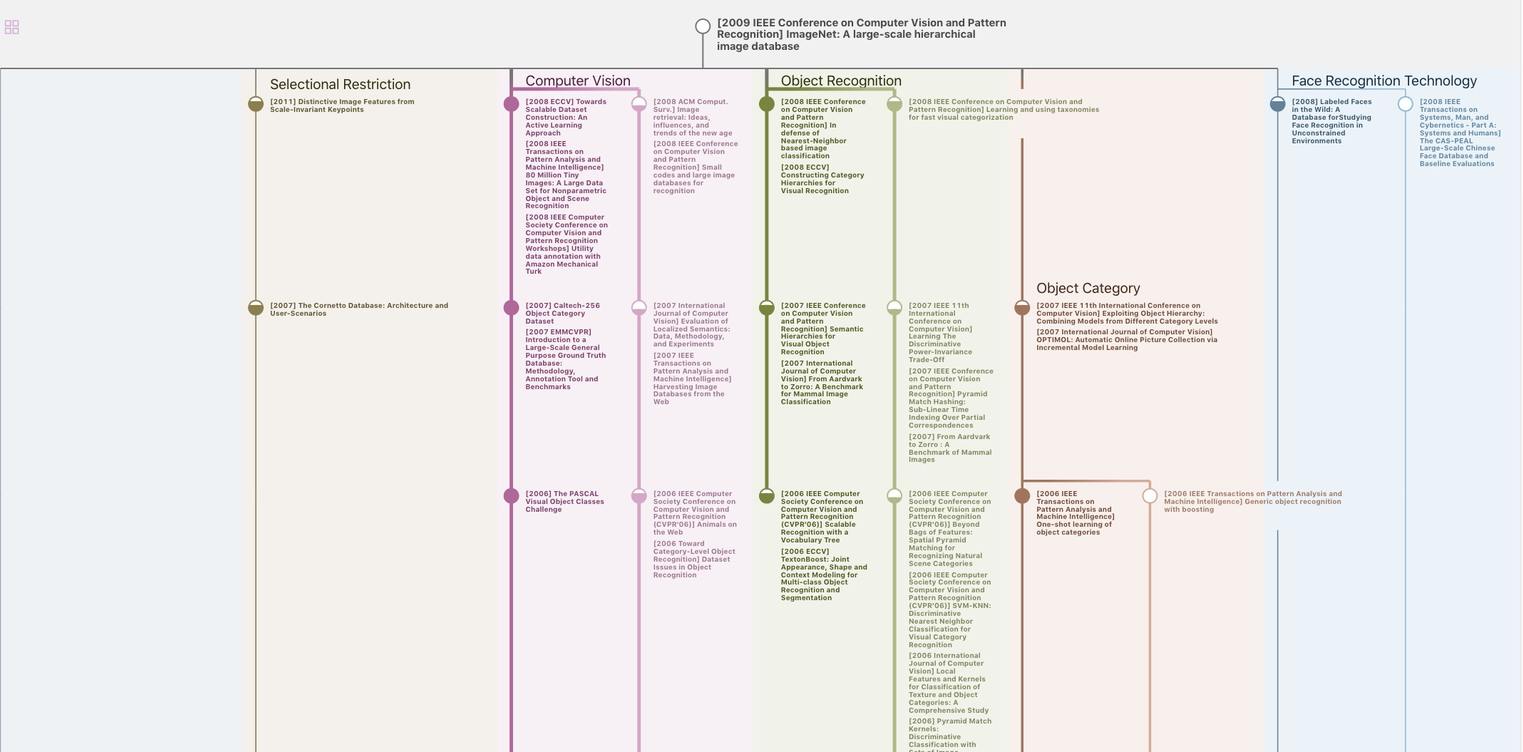
生成溯源树,研究论文发展脉络
Chat Paper
正在生成论文摘要