Global Convergence Rate Analysis Of A Generic Line Search Algorithm With Noise
SIAM JOURNAL ON OPTIMIZATION(2021)
摘要
In this paper, we develop convergence analysis of a modified line search method for objective functions whose value is computed with noise and whose gradient estimates are inexact and possibly random. The noise is assumed to be bounded in absolute value without any additional assumptions. We extend the framework based on stochastic methods from [C. Cartis and K. Scheinberg, Math. Program., 169 (2018), pp. 337-375] which was developed to provide analysis of a standard line search method with exact function values and random gradients to the case of noisy functions. We introduce two alternative conditions on the gradient which, when satisfied with some sufficiently large probability at each iteration, guarantees convergence properties of the line search method. We derive expected complexity bounds to reach a near optimal neighborhood for convex, strongly convex and nonconvex functions. The exact dependence of the convergence neighborhood on the noise is specified.
更多查看译文
关键词
nonlinear optimization, line search, convergence rates, derivative-free optimization
AI 理解论文
溯源树
样例
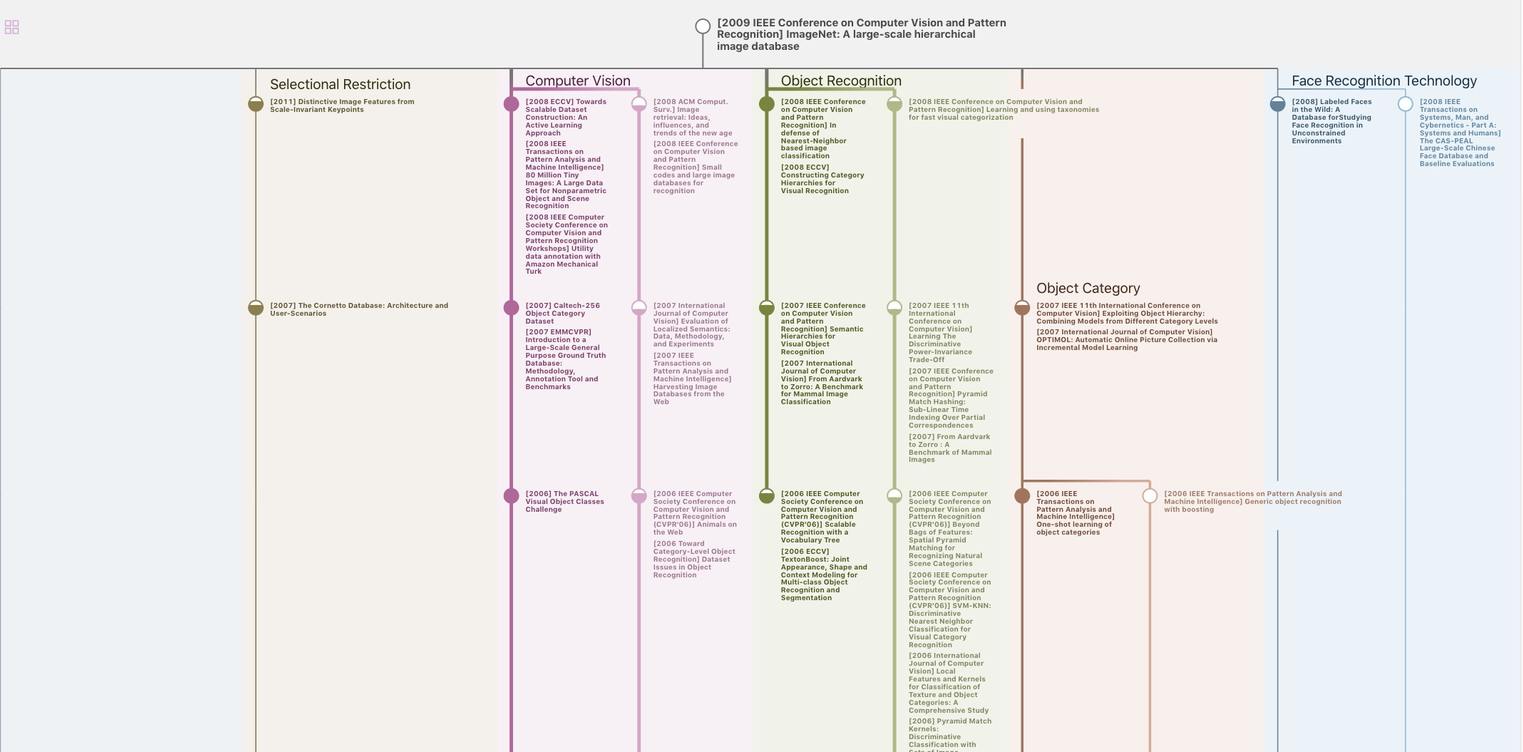
生成溯源树,研究论文发展脉络
Chat Paper
正在生成论文摘要