Policy Optimization Through Approximated Importance Sampling
arxiv(2019)
摘要
Recent policy optimization approaches (Schulman et al., 2015a, 2017) have achieved substantial empirical successes by constructing new proxy optimization objectives. These proxy objectives allow stable and low variance policy learning, but require small policy updates to ensure that the proxy objective remains an accurate approximation of the target policy value. In this paper we derive an alternative objective that obtains the value of the target policy by applying importance sampling. This objective can be directly estimated from samples, as it takes an expectation over trajectories generated by the current policy. However, the basic importance sampled objective is not suitable for policy optimization, as it incurs unacceptable variance. We therefore introduce an approximation that allows us to directly trade-off the bias of approximation with the variance in policy updates. We show that our approximation unifies the proxy optimization approaches with the importance sampling objective and allows us to interpolate between them. We then provide a theoretical analysis of the method that directly quantifies the error term due to the approximation. Finally, we obtain a practical algorithm by optimizing the introduced objective with proximal policy optimization techniques (Schulman etal., 2017). We empirically demonstrate that the result-ing algorithm yields superior performance on continuous control benchmarks
更多查看译文
关键词
approximate importance sampling,optimization,policy
AI 理解论文
溯源树
样例
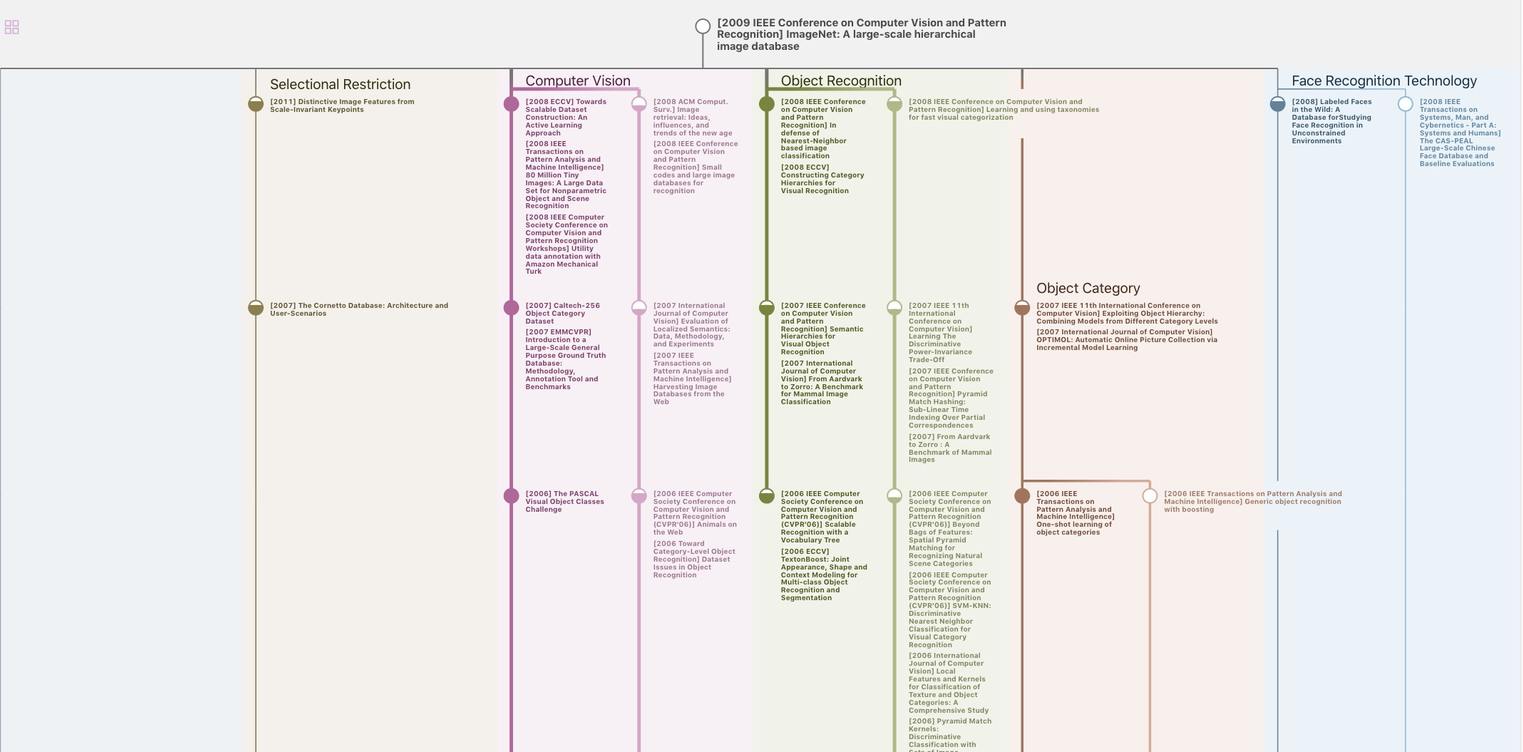
生成溯源树,研究论文发展脉络
Chat Paper
正在生成论文摘要