On Post-selection Inference in A/B Testing
Knowledge Discovery and Data Mining(2021)
摘要
ABSTRACTWhen interpreting A/B tests, we typically focus only on the statistically significant results and take them by face value. This practice, termed post-selection inference in the statistical literature, may negatively affect both point estimation and uncertainty quantification, and therefore hinder trustworthy decision making in A/B testing. To address this issue, in this paper we explore two seemingly unrelated paths, one based on supervised machine learning and the other on empirical Bayes, and propose post-selection inferential approaches that combine the strengths of both. Through large-scale simulated and empirical examples, we demonstrate that our proposed methodologies stand out among other existing ones in both reducing post-selection biases and improving confidence interval coverage rates, and discuss how they can be conveniently adjusted to real-life scenarios.
更多查看译文
关键词
A/B testing, big data, machine learning, regression, empirical Bayes, online metrics, randomization, post-selection inference, bias correction, winner's curse
AI 理解论文
溯源树
样例
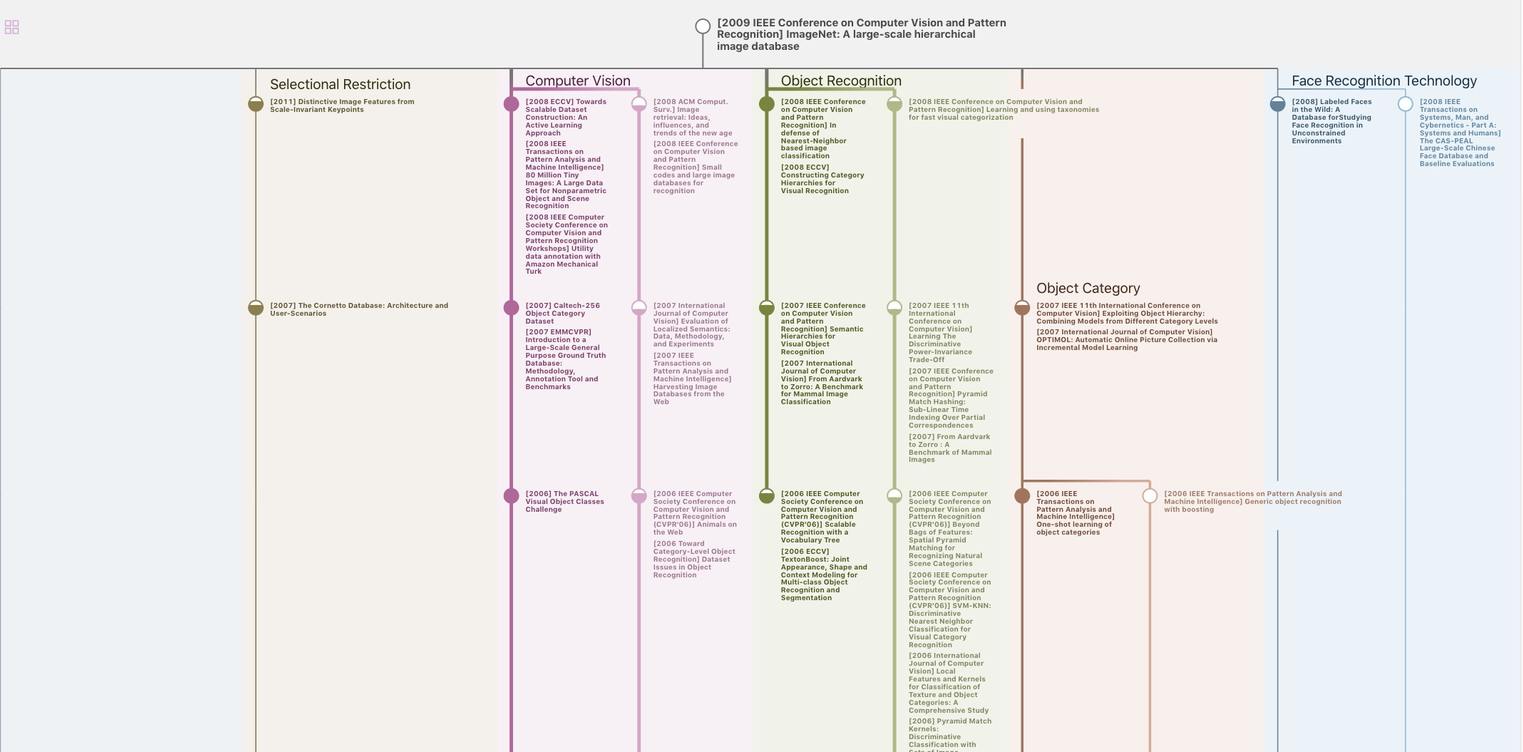
生成溯源树,研究论文发展脉络
Chat Paper
正在生成论文摘要