First Order Methods Beyond Convexity and Lipschitz Gradient Continuity with Applications to Quadratic Inverse Problems
SIAM JOURNAL ON OPTIMIZATION(2018)
摘要
We focus on nonconvex and nonsmooth minimization problems with a composite objective, where the differentiable part of the objective is freed from the usual and restrictive global Lipschitz gradient continuity assumption. This longstanding smoothness restriction is pervasive in first order methods, and recently was circumvented for convex composite optimization by Bauschke, Bolte, and Teboulle, through a simple framework which captures, all at once, the geometry of the function and of the feasible set. Building on this work, we tackle genuine nonconvex problems. We first complement and extend their approach to derive an extended descent lemma by introducing the notion of smooth adaptable functions. We then consider a Bregman-based proximal gradient method for the nonconvex composite model with smooth adaptable functions, which is proven to globally converge to a critical point under natural assumptions on the problem's data, and in particular for semialgebraic problems. To illustrate the potential of our general framework and results, we consider a broad class of quadratic inverse problems with sparsity constraints which arises in many fundamental applications, and we apply our approach to derive new globally convergent schemes for this class.
更多查看译文
关键词
composite nonconvex nonsmooth minimization,proximal gradient algorithms,extended descent lemma,non-Euclidean distances,Bregman distance,global convergence,Kurdyka-Lojasiewicz property,semialgebraic functions,quadratic inverse problems,phase retrieval
AI 理解论文
溯源树
样例
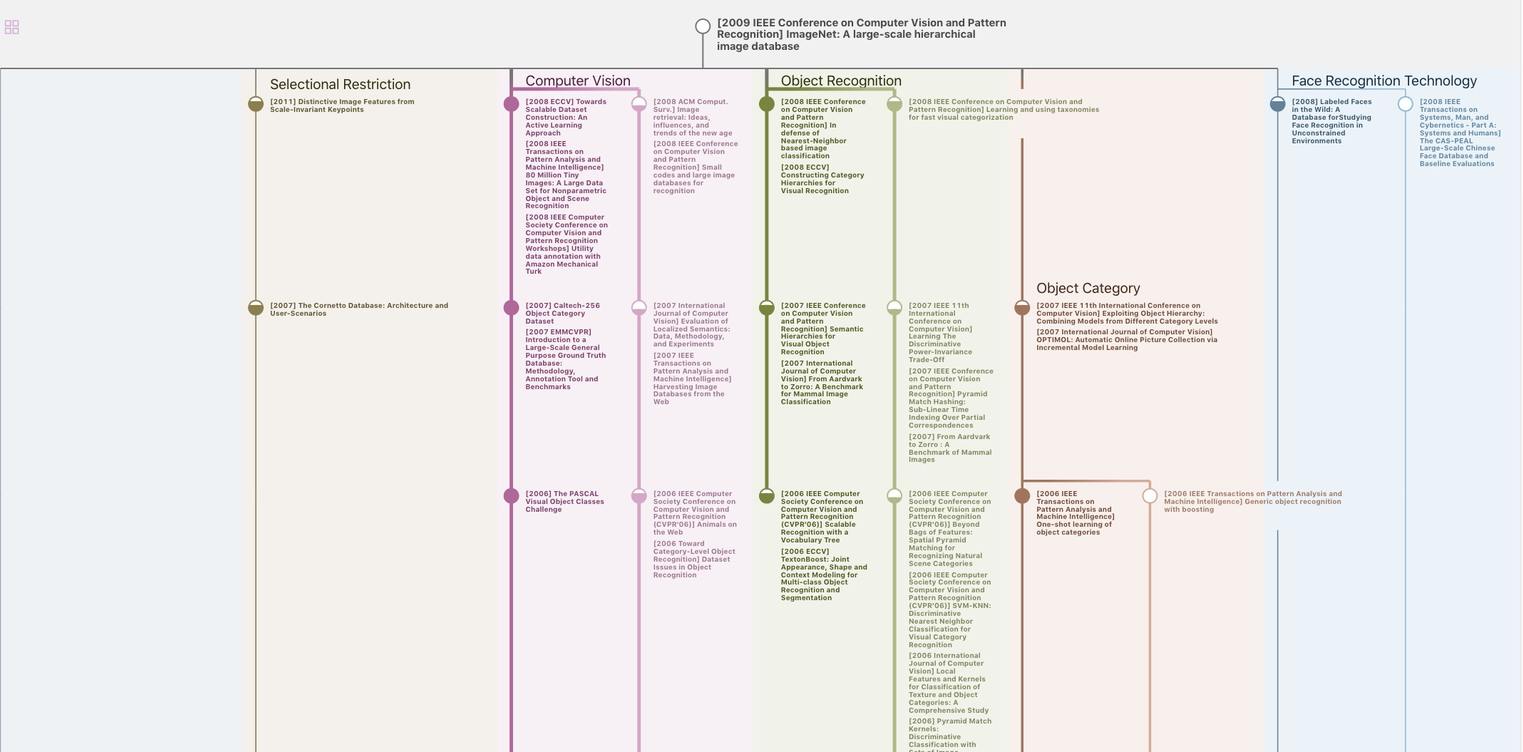
生成溯源树,研究论文发展脉络
Chat Paper
正在生成论文摘要