Semiparametric bayesian analysis of transformation spatial mixed models for large datasets
STATISTICS AND ITS INTERFACE(2019)
摘要
In spatial mixed models (SMMs), it is commonly assumed that stationary spatial process and random errors independently follow the Gassian distribution. However, in some applications, this assumption may be inappropriate. To this end, this paper proposes a transformation spatial mixed models (TSMMs) to accommodate large dataset that follows the non-Gaussian distribution. With the help of Gibbs sampler algorithm, a semiparametric Bayesian approach is developed to make inference on TSMMs by using Bayesian P-splines to approximate transformation function, and a fixed number of known but not necessarily orthogonal spatial basis functions with multi-resolution analysis method to approximate nonstationary spatial process. Instead of Wishart distribution assumption for the prior of precision matrix of random effects, we consider Cholesky decomposition of the precision matrix, and specify the priors for unknown components in low unit triangular matrix and diagonal matrix. Simulation studies and an example are used to illustrate the proposed methodologies.
更多查看译文
关键词
Gibbs sampler,Large dataset,Matrix decomposition,Semiparametric Bayesian analysis,Transformation spatial Mixed Models
AI 理解论文
溯源树
样例
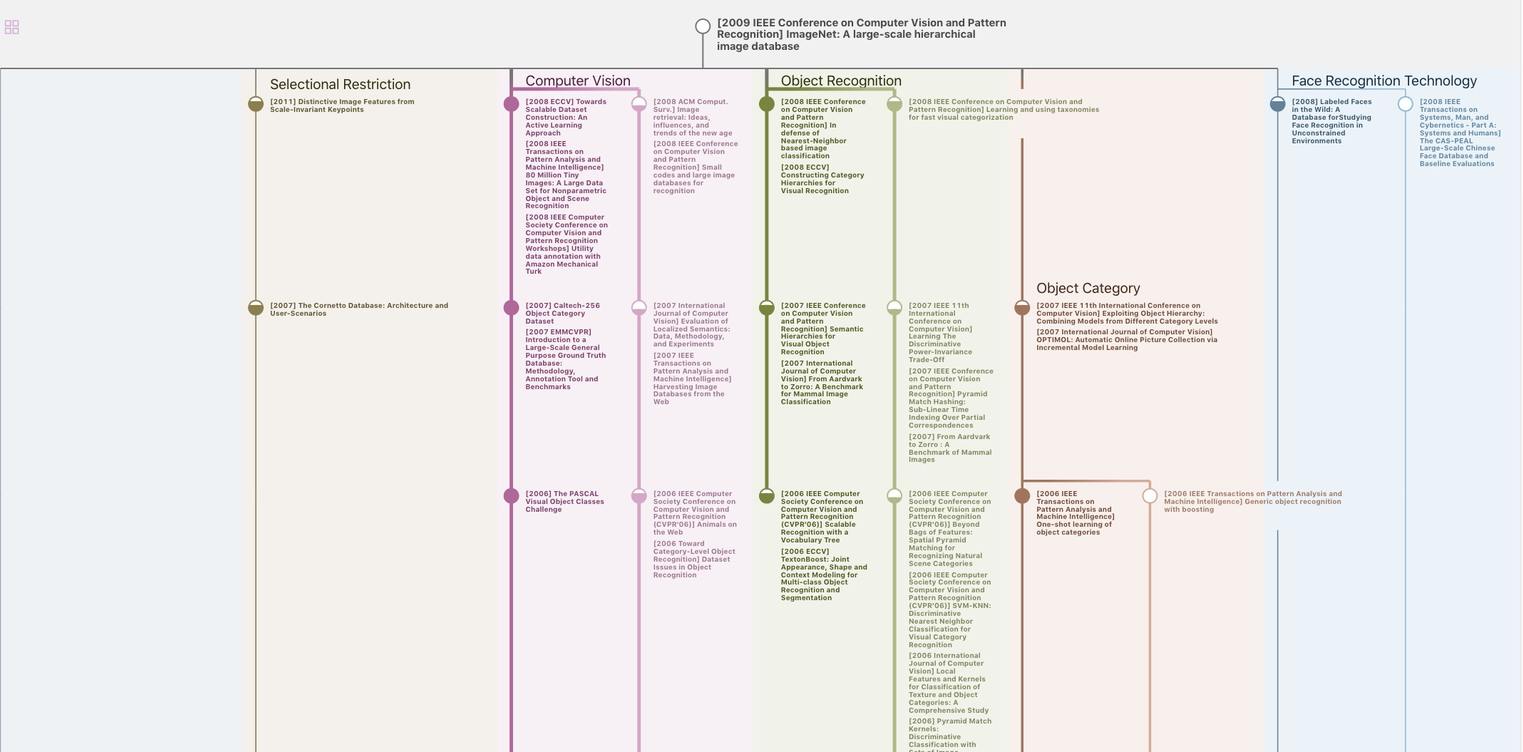
生成溯源树,研究论文发展脉络
Chat Paper
正在生成论文摘要