Extracting Activity Patterns From Taxi Trajectory Data: A Two-Layer Framework Using Spatio-Temporal Clustering, Bayesian Probability And Monte Carlo Simulation
INTERNATIONAL JOURNAL OF GEOGRAPHICAL INFORMATION SCIENCE(2020)
摘要
Global positioning system (GPS) data generated from taxi trips is a valuable source of information that offers an insight into travel behaviours of urban populations with high spatio-temporal resolution. However, in its raw form, GPS taxi data does not offer information on the purpose (or intended activity) of travel. In this context, to enhance the utility of taxi GPS data sets, we propose a two-layer framework to identify the related activities of each taxi trip automatically and estimate the return trips and successive activities after the trip, by using geographic point-of-interest (POI) data and a combination of spatio-temporal clustering, Bayesian inference and Monte Carlo simulation. Two million taxi trips in New York, the United States of America, and ten million taxi trips in Shenzhen, China, are used as inputs for the two-layer framework. To validate each layer of the framework, we collect 6,003 trip diaries in New York and 712 questionnaire surveys in Shenzhen. The results show that the first layer of the framework performs better than comparable methods published in the literature, while the second layer has high accuracy when inferring return trips.
更多查看译文
关键词
Spatio-temporal clustering, Bayesian probabilities, Monte Carlo simulation, travel behaviours
AI 理解论文
溯源树
样例
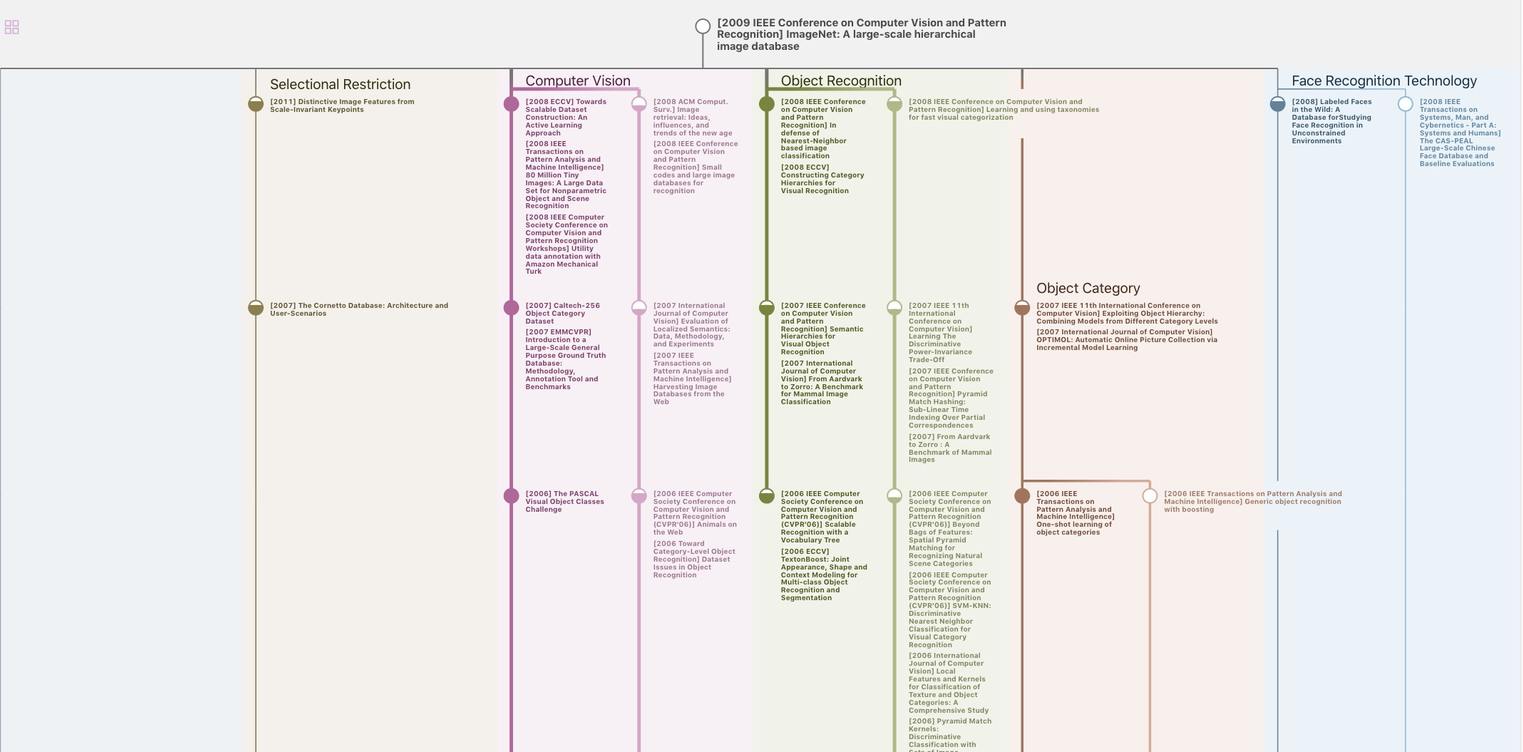
生成溯源树,研究论文发展脉络
Chat Paper
正在生成论文摘要