Help me find a job: A graph-based approach for job recommendation at scale
2017 IEEE INTERNATIONAL CONFERENCE ON BIG DATA (BIG DATA)(2017)
摘要
Online job boards are one of the central components of modern recruitment industry. With millions of candidates browsing through job postings everyday, the need for accurate, effective, meaningful, and transparent job recommendations is apparent more than ever. While recommendation systems are successfully advancing in variety of online domains by creating social and commercial value, the job recommendation domain is less explored. Existing systems are mostly focused on content analysis of resumes and job descriptions, relying heavily on the accuracy and coverage of the semantic analysis and modeling of the content in which case, they end up usually suffering from rigidity and the lack of implicit semantic relations that are uncovered from users' behavior and could be captured by Collaborative Filtering (CF) methods. Few works which utilize CF do not address the scalability challenges of real-world systems and the problem of cold-start. In this paper, we propose a scalable item-based recommendation system for online job recommendations. Our approach overcomes the major challenges of sparsity and scalability by leveraging a directed graph of jobs connected by multi-edges representing various behavioral and contextual similarity signals. The short lived nature of the items (jobs) in the system and the rapid rate in which new users and jobs enter the system make the cold-start a serious problem hindering CF methods. We address this problem by harnessing the power of deep learning in addition to user behavior to serve hybrid recommendations. Our technique has been leveraged by CareerBuilder.com which is one of the largest job boards in the world to generate high-quality recommendations for millions of users.
更多查看译文
关键词
Large-scale Recommendations, Graph-based Recommendations, Deep learning for Recommendations, Job Recommendations, Hybrid Recommendations
AI 理解论文
溯源树
样例
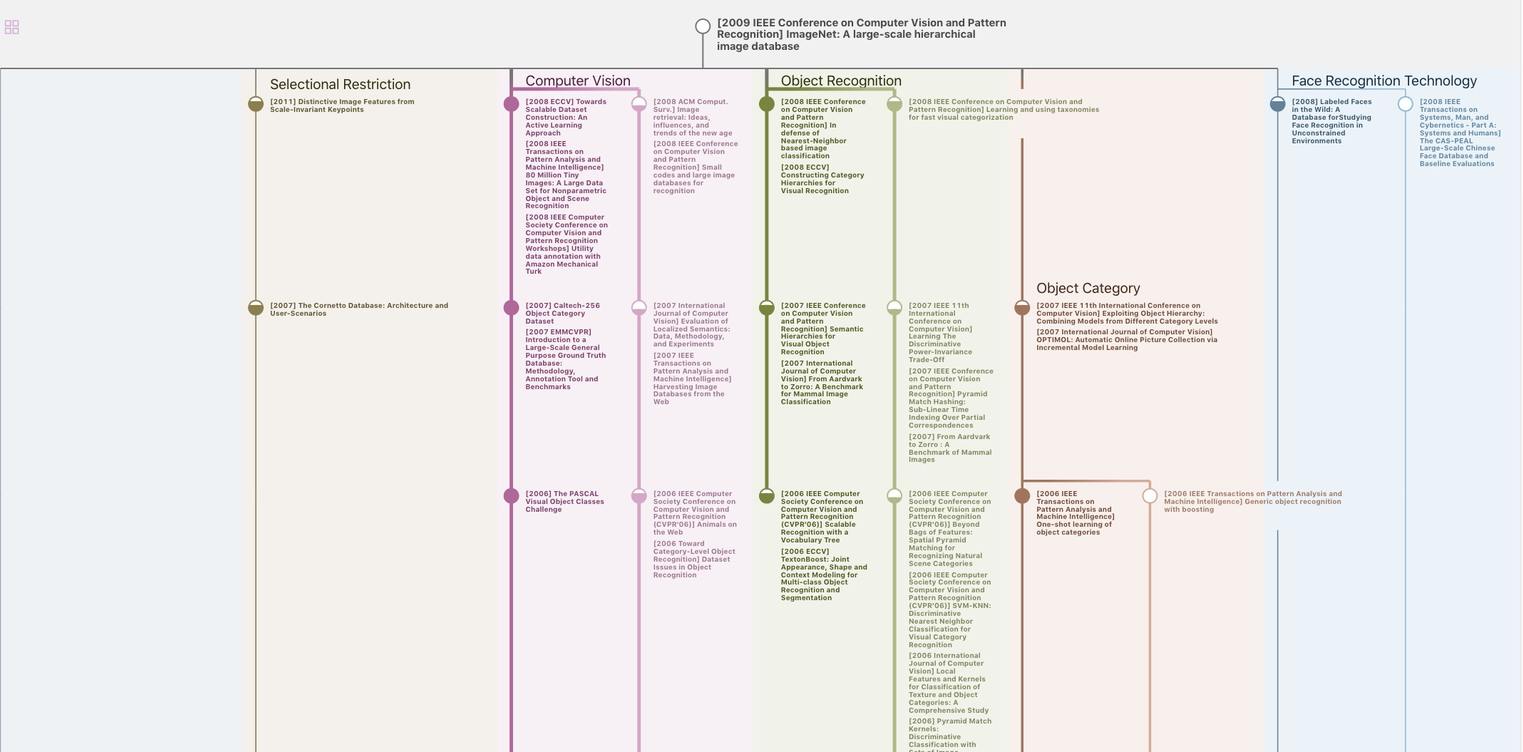
生成溯源树,研究论文发展脉络
Chat Paper
正在生成论文摘要