Chaotic Time Series Prediction Using A Novel Echo State Network Model With Input Reconstruction, Bayesian Ridge Regression And Independent Component Analysis
INTERNATIONAL JOURNAL OF PATTERN RECOGNITION AND ARTIFICIAL INTELLIGENCE(2020)
摘要
This paper presents a novel Echo State Network (ESN) model for chaotic time series prediction, which consists of three steps including input reconstruction, dimensionality reduction and regression. First, phase-space reconstruction is used to reconstruct the original 'attractor' of the input time series. Then, Independent Component Analysis (ICA) is used to identify independent components, reduce dimensionality and overcome multicollinearity problem of the reconstructed input matrix. Finally, Bayesian Ridge Regression provides accurate predictions thanks to its regularization effect to avoid over-fitting and its robustness to noise owing to its probabilistic strategy. Our experimental results show that our model significantly outperforms other ESN models in predicting both artificial and real-world chaotic time series.
更多查看译文
关键词
Echo State Network, phase-space reconstruction, Bayesian Ridge Regression, independent component analysis, time series prediction
AI 理解论文
溯源树
样例
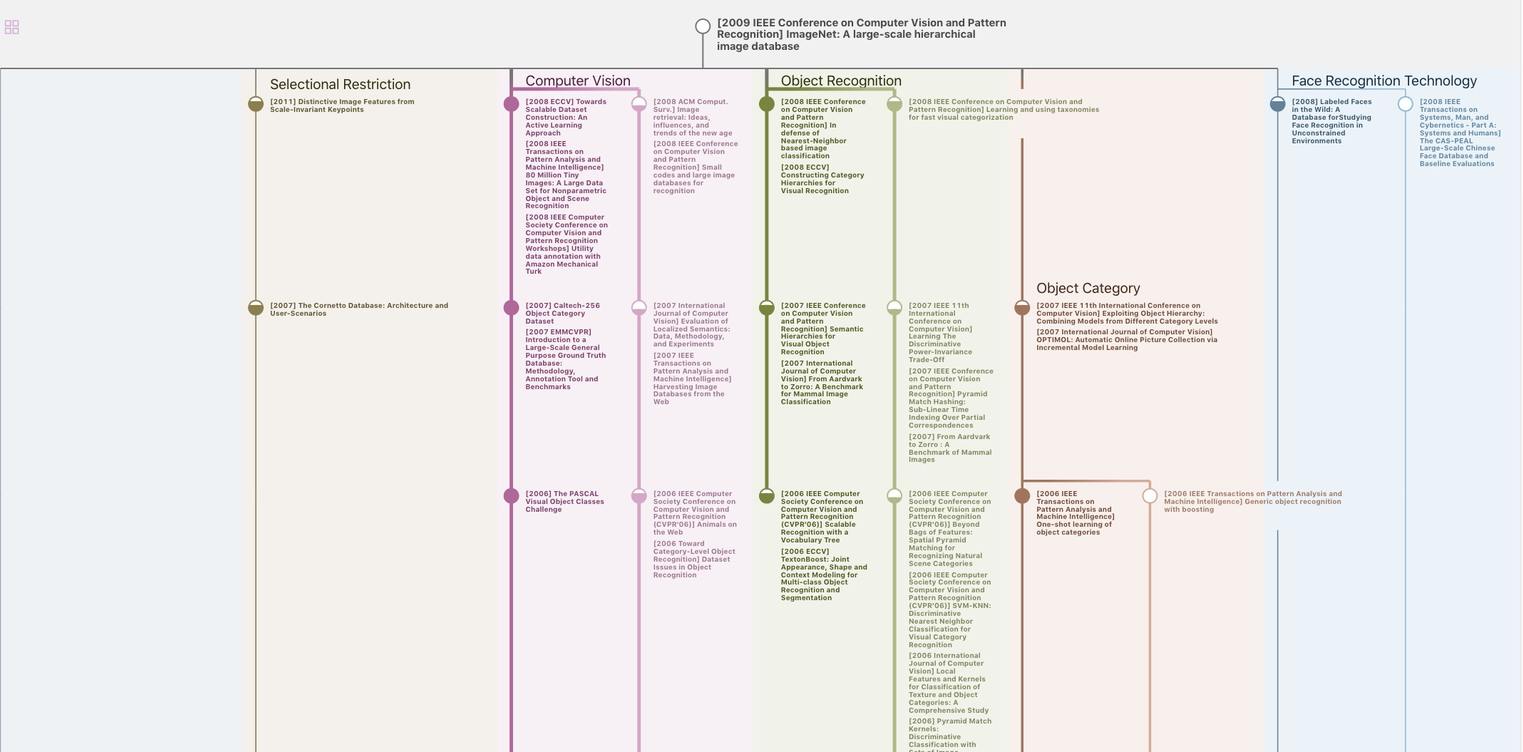
生成溯源树,研究论文发展脉络
Chat Paper
正在生成论文摘要