Recurrent Segmentation for Variable Computational Budgets
IEEE Conference on Computer Vision and Pattern Recognition(2018)
摘要
State-of-the-art systems for semantic image segmentation use feed-forward pipelines with fixed computational costs. Building an image segmentation system that works across a range of computational budgets is challenging and time-intensive as new architectures must be designed and trained for every computational setting. To address this problem we develop a recurrent neural network that successively improves prediction quality with each iteration. Importantly, the RNN may be deployed across a range of computational budgets by merely running the model for a variable number of iterations. We find that this architecture is uniquely suited for efficiently segmenting videos. By exploiting the segmentation of past frames, the RNN can perform video segmentation at similar quality but reduced computational cost compared to state-of-the-art image segmentation methods. When applied to static images in the PASCAL VOC 2012 and Cityscapes segmentation datasets, the RNN traces out a speed-accuracy curve that saturates near the performance of state-of-the-art segmentation methods.
更多查看译文
关键词
recurrent segmentation,variable computational budgets,semantic image segmentation,feed-forward pipelines,fixed computational costs,image segmentation system,computational setting,recurrent neural network,RNN,video segmentation,computational cost,static images,Cityscapes segmentation datasets,prediction quality,image segmentation methods
AI 理解论文
溯源树
样例
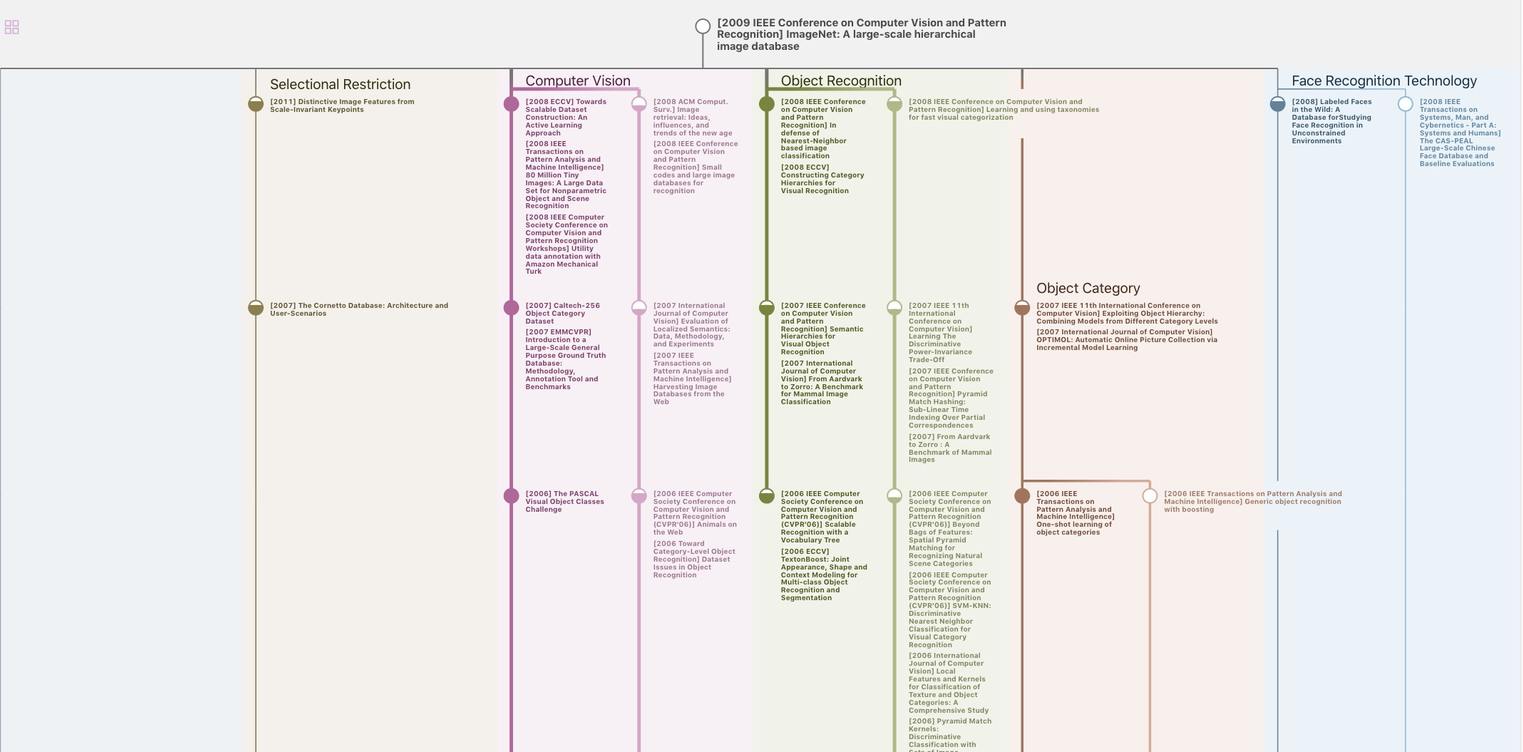
生成溯源树,研究论文发展脉络
Chat Paper
正在生成论文摘要