Proximally Guided Stochastic Subgradient Method for Nonsmooth, Nonconvex Problems
SIAM JOURNAL ON OPTIMIZATION(2019)
摘要
In this paper, we introduce a stochastic projected subgradient method for weakly convex (i.e., uniformly prox-regular) nonsmooth, nonconvex functions -a wide class of functions which includes the additive and convex composite classes. At a high level, the method is an inexact proximal-point iteration in which the strongly convex proximal subproblems are quickly solved with a specialized stochastic projected subgradient method. The primary contribution of this paper is a simple proof that the proposed algorithm converges at the same rate as the stochastic gradient method for smooth nonconvex problems. This result appears to be the first convergence rate analysis of a stochastic (or even deterministic) subgradient method for the class of weakly convex functions. In addition, a two-phase variant is proposed that significantly reduces the variance of the solutions returned by the algorithm. Finally, preliminary numerical experiments are also provided.
更多查看译文
关键词
nonsmooth,nonconvex,subgradient,stochastic,proximal
AI 理解论文
溯源树
样例
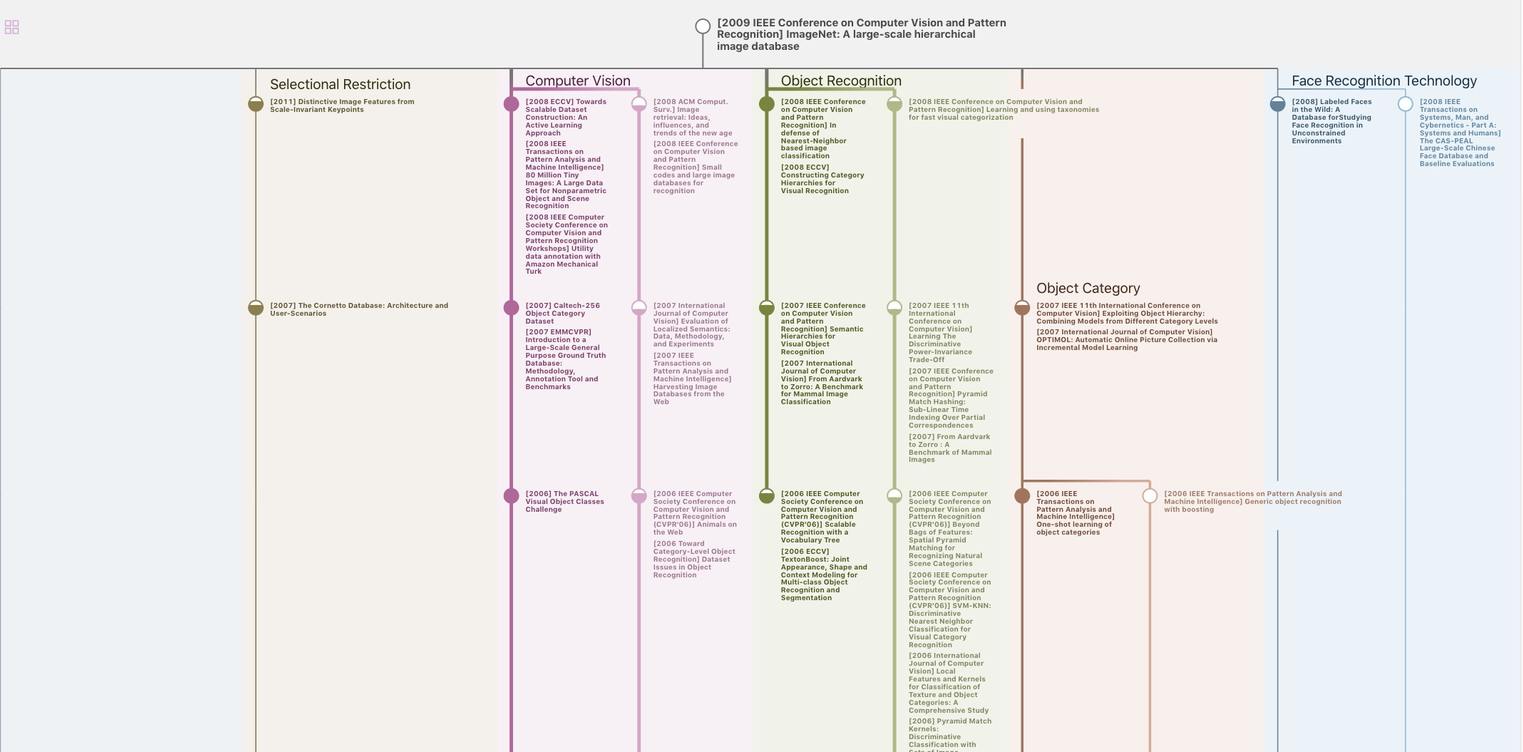
生成溯源树,研究论文发展脉络
Chat Paper
正在生成论文摘要