Extracting Sparse High-Dimensional Dynamics from Limited Data
SIAM JOURNAL ON APPLIED MATHEMATICS(2018)
摘要
Extracting governing equations from dynamic data is an essential task in model selection and parameter estimation. The form of the governing equation is rarely known a priori; however, based on the sparsity-of-effect principle one may assume that the number of candidate functions needed to represent the dynamics is very small. In this work, we leverage the sparse structure of the governing equations along with recent results from random sampling theory to develop methods for selecting dynamical systems from undersampled data. In particular, we detail three sampling strategies that lead to the exact recovery of first-order dynamical systems when we are given fewer samples than unknowns. The first method makes no assumptions on the behavior of the data, and requires a certain number of random initial samples. The second method utilizes the structure of the governing equation to limit the number of random initializations needed. The third method leverages chaotic behavior in the data to construct a nearly deterministic sampling strategy. Using results from compressive sensing, we show that the strategies lead to exact recovery, which is stable to the sparse structure of the governing equations and robust to noise in the estimation of the velocity. Computational results validate each of the sampling strategies and highlight potential applications.
更多查看译文
关键词
high-dimensional dynamical systems,sparse optimization,model selection,exact recovery,undersampled data,chaos
AI 理解论文
溯源树
样例
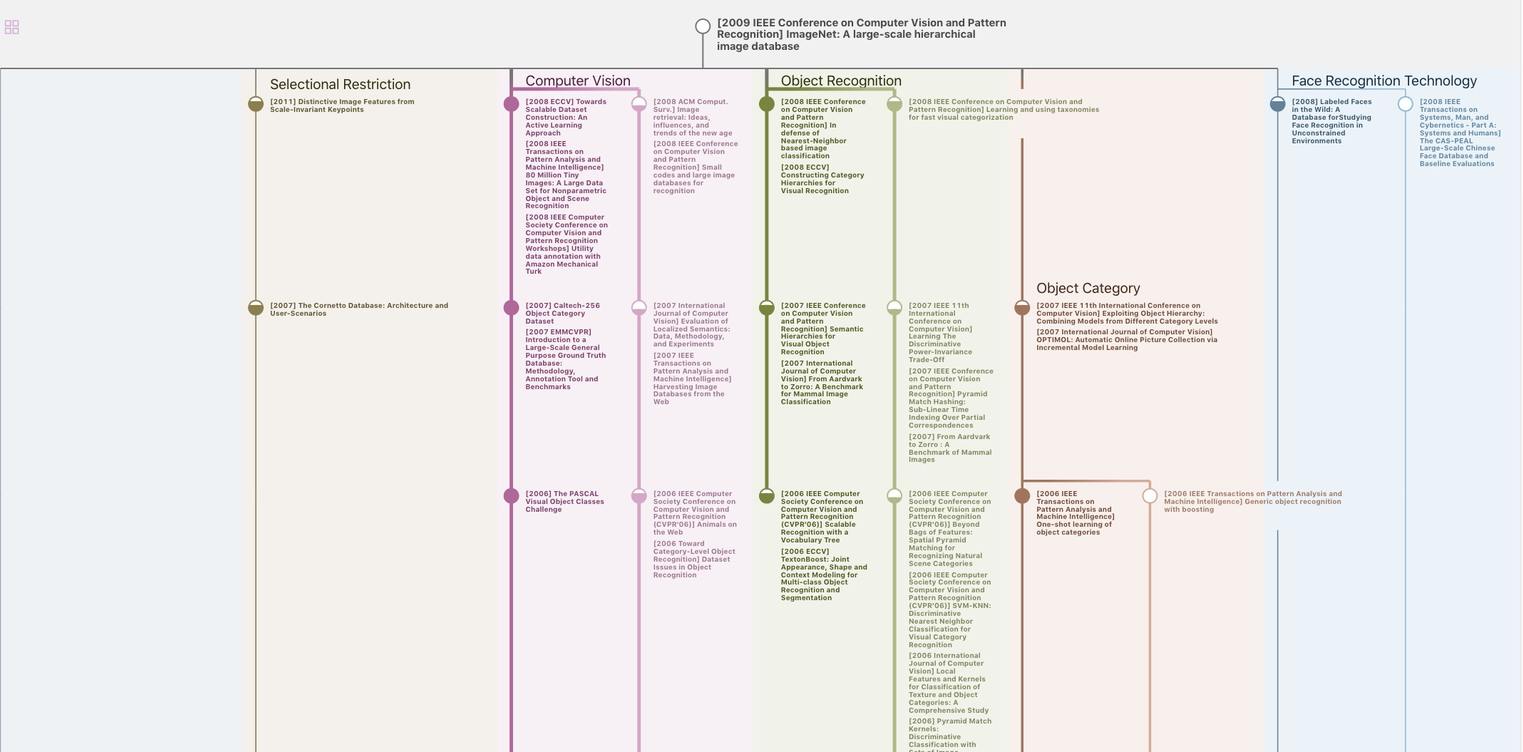
生成溯源树,研究论文发展脉络
Chat Paper
正在生成论文摘要