CosFace: Large Margin Cosine Loss for Deep Face Recognition
IEEE Conference on Computer Vision and Pattern Recognition(2018)
摘要
Face recognition has made extraordinary progress owing to the advancement of deep convolutional neural networks (CNNs). The central task of face recognition, including face verification and identification, involves face feature discrimination. However, the traditional softmax loss of deep CNNs usually lacks the power of discrimination. To address this problem, recently several loss functions such as center loss, large margin softmax loss, and angular softmax loss have been proposed. All these improved losses share the same idea: maximizing inter-class variance and minimizing intra-class variance. In this paper, we propose a novel loss function, namely large margin cosine loss (LMCL), to realize this idea from a different perspective. More specifically, we reformulate the softmax loss as a cosine loss by $L_2$ normalizing both features and weight vectors to remove radial variations, based on which a cosine margin term is introduced to further maximize the decision margin in the angular space. As a result, minimum intra-class variance and maximum inter-class variance are achieved by virtue of normalization and cosine decision margin maximization. We refer to our model trained with LMCL as CosFace. Extensive experimental evaluations are conducted on the most popular public-domain face recognition datasets such as MegaFace Challenge, Youtube Faces (YTF) and Labeled Face in the Wild (LFW). We achieve the state-of-the-art performance on these benchmarks, which confirms the effectiveness of our proposed approach.
更多查看译文
关键词
deep convolutional neural networks,face verification,face feature discrimination,deep CNNs,center loss,angular softmax loss,loss function,normalization,cosine decision margin maximization,face identification,loss functions,intra-class variance,inter-class variance,deep face recognition,large margin cosine loss,CosFace,large margin softmax loss,LMCL,weight vectors,MegaFace Challenge,Youtube Faces,Labeled Face in the Wild,YTF,LFW
AI 理解论文
溯源树
样例
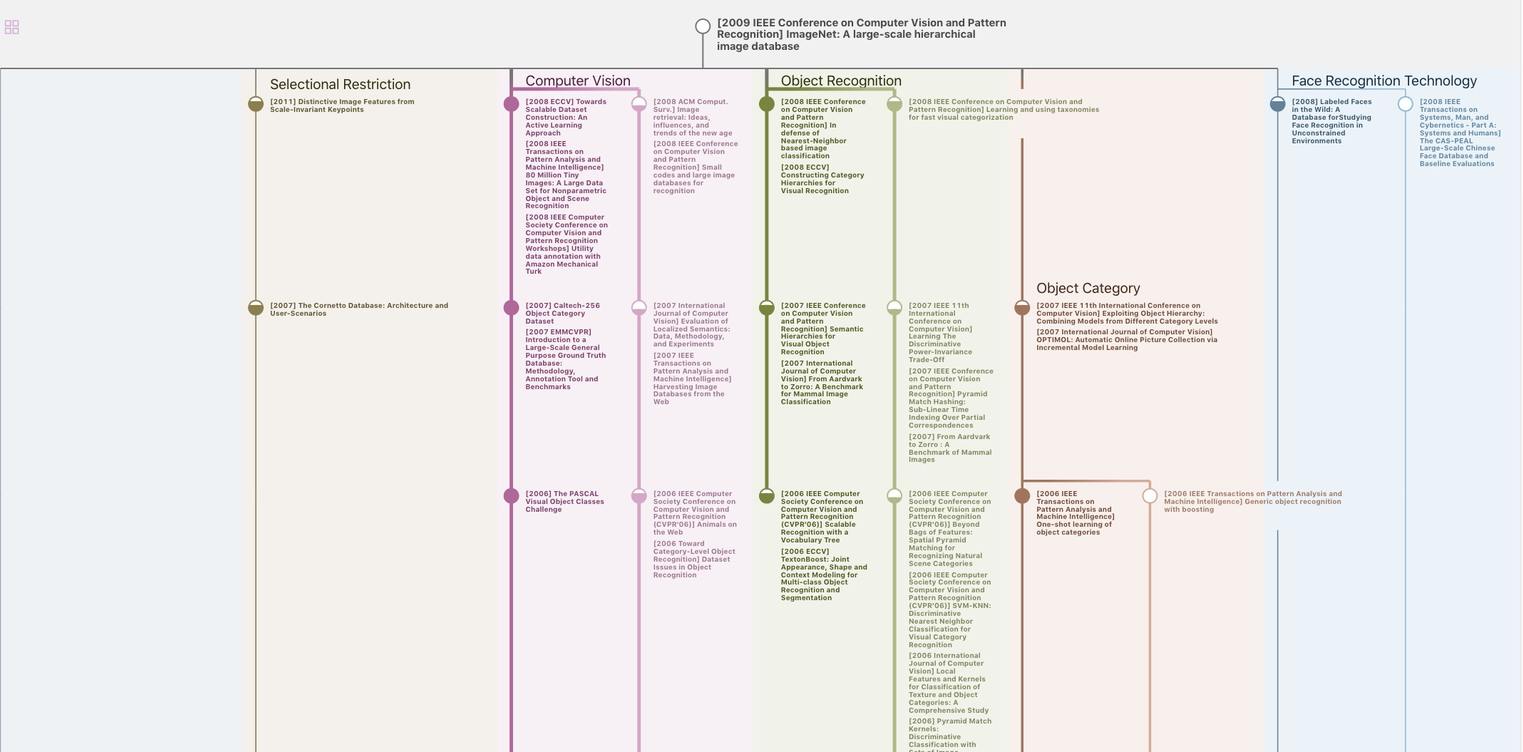
生成溯源树,研究论文发展脉络
Chat Paper
正在生成论文摘要