Repmet: Representative-Based Metric Learning For Classification And Few-Shot Object Detection
computer vision and pattern recognition(2019)
摘要
Distance metric learning (DML) has been successfully applied to object classification,both in the standard regime of rich training data and in the few-shot scenario, where each category is represented by only a few examples. In this work, we propose a new method for DML that simultaneously learns the backbone network parameters,the embedding space, and the multi-modal distribution of each of the training categories in that space, in a single end-to-end training process. Our approach outperforms state-of-the-art methods for DML-based object classification on a variety of standard fine-grained datasets. Furthermore, we demonstrate the effectiveness of our approach on the problem of few-shot object detection, by incorporating the proposed DML architecture as a classification head into a standard object detection model. We achieve the best results on the ImageNet-LOC dataset compared to strong baselines, when only a few training examples are available. We also offer the community a new episodic benchmark based on the ImageNet dataset for the few-shot object detection task.
更多查看译文
关键词
Recognition: Detection,Categorization,Retrieval,Deep Learning , Representation Learning
AI 理解论文
溯源树
样例
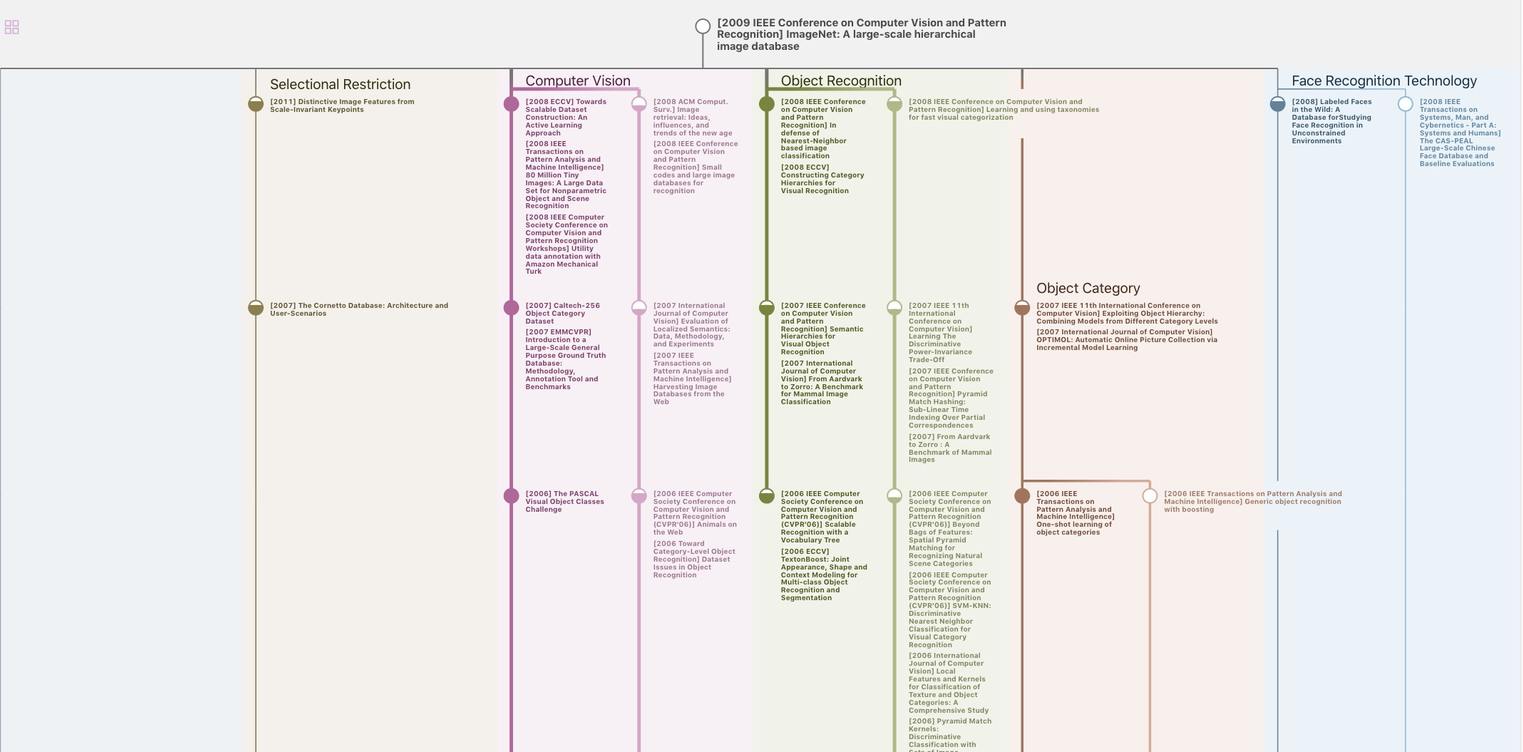
生成溯源树,研究论文发展脉络
Chat Paper
正在生成论文摘要