Low-Rank Matrix Approximations Do Not Need a Singular Value Gap
SIAM JOURNAL ON MATRIX ANALYSIS AND APPLICATIONS(2019)
摘要
Low-rank approximations to a real matrix A can be conputed from ZZ(T)A, where Z is a matrix with orthonormal columns, and the accuracy of the approximation can be estimated from some norm of A-ZZ(T)A. We show that computing A-ZZ(T)A in the two-norm, Frobenius norms, and more generally any Schatten p-norm is a well-posed mathematical problem; and, in contrast to dominant subspace computations, it does not require a singular value gap. We also show that this problem is well-conditioned (insensitive) to additive perturbations in A and Z, and to dimensionchanging or multiplicative perturbations in A-regardless of the accuracy of the approximation. For the special case when A does indeed have a singular values gap, connections are established between low-rank approximations and subspace angles.
更多查看译文
关键词
singular value decomposition,principal angles,additive perturbations,multiplicative perturbations
AI 理解论文
溯源树
样例
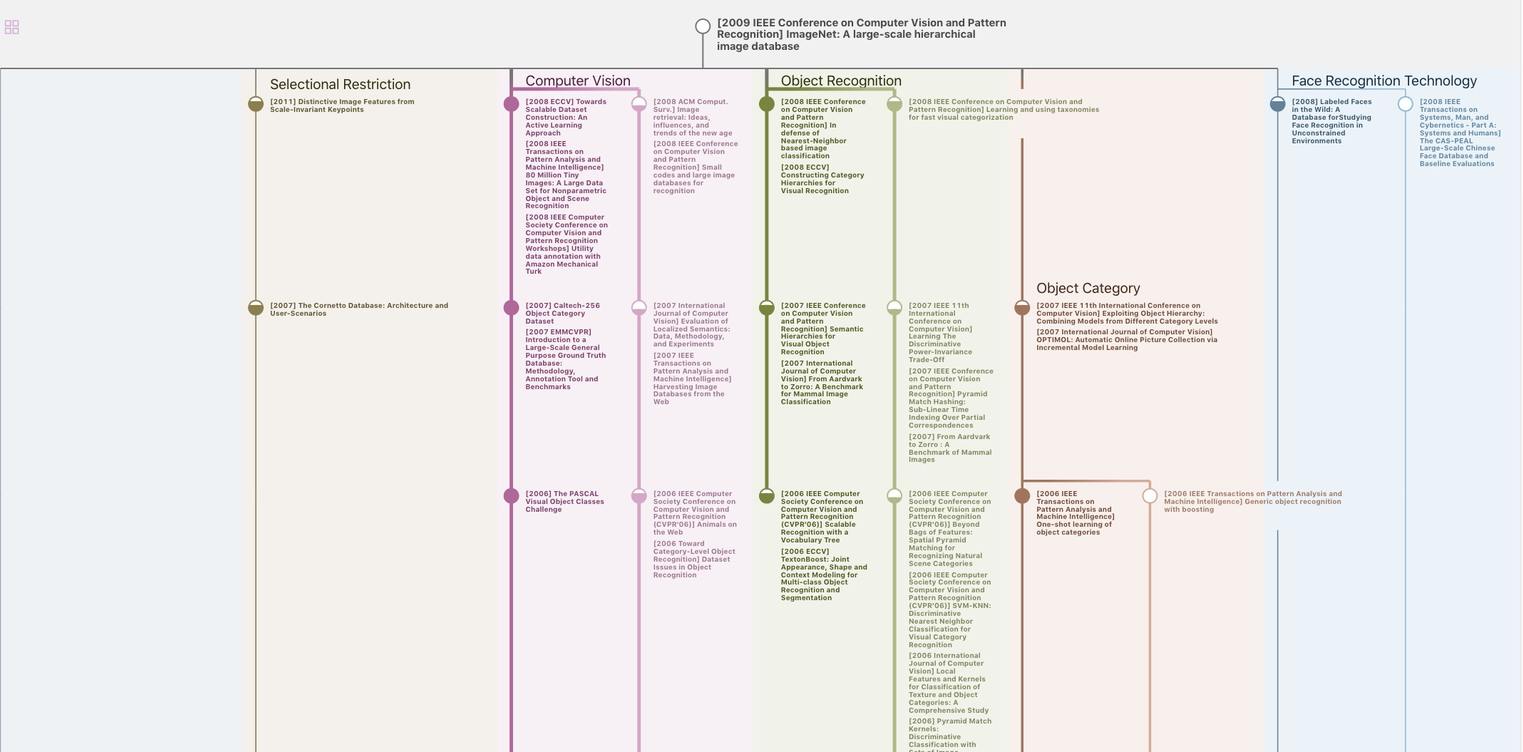
生成溯源树,研究论文发展脉络
Chat Paper
正在生成论文摘要