A CNN-BASED RETINAL IMAGE QUALITY ASSESSMENT SYSTEM FOR TELEOPHTHALMOLOGY
JOURNAL OF MECHANICS IN MEDICINE AND BIOLOGY(2019)
摘要
Oculopathy is a widespread disease among people of all ages around the world. Teleophthalmology can facilitate the ophthalmological diagnosis for less developed countries that lack medical resources. In teleophthalmology, the assessment of retinal image quality is of great importance. In this paper, we propose a no-reference retinal image assessment system based on DenseNet, a convolutional neural network architecture. This system classified fundus images into good and bad quality or five categories: adequate, just noticeable blur, inappropriate illumination, incomplete optic disc, and opacity. The proposed system was evaluated on different datasets and compared to the applications based on other two networks: VGG-16 and GoogLenet. For binary classification, the good-and-bad binary classifier achieves an AUC of 1.000, and the degradation-specified classifiers that distinguish one specified degradation versus the rest achieve AUC values of 0.972, 0.990, 0.982, 0.982 for four categories, respectively. The multi-classification based on DenseNet achieves an overall accuracy of 0.927, which is significantly higher than 0.549 and 0.757 obtained using VGG-16 and GoogLeNet, respectively. The experimental results indicate that the proposed approach produces outstanding performance in retinal image quality assessment and is worth applying in ophthalmological telemedicine applications. In addition, the proposed approach is robust to the image noise. This study fills the gap of multi-classification in retinal image quality assessment.
更多查看译文
关键词
Retinal image,image quality assessment,degradation-specified classification,multi-classification,convolutional neuron network,teleophthalmology
AI 理解论文
溯源树
样例
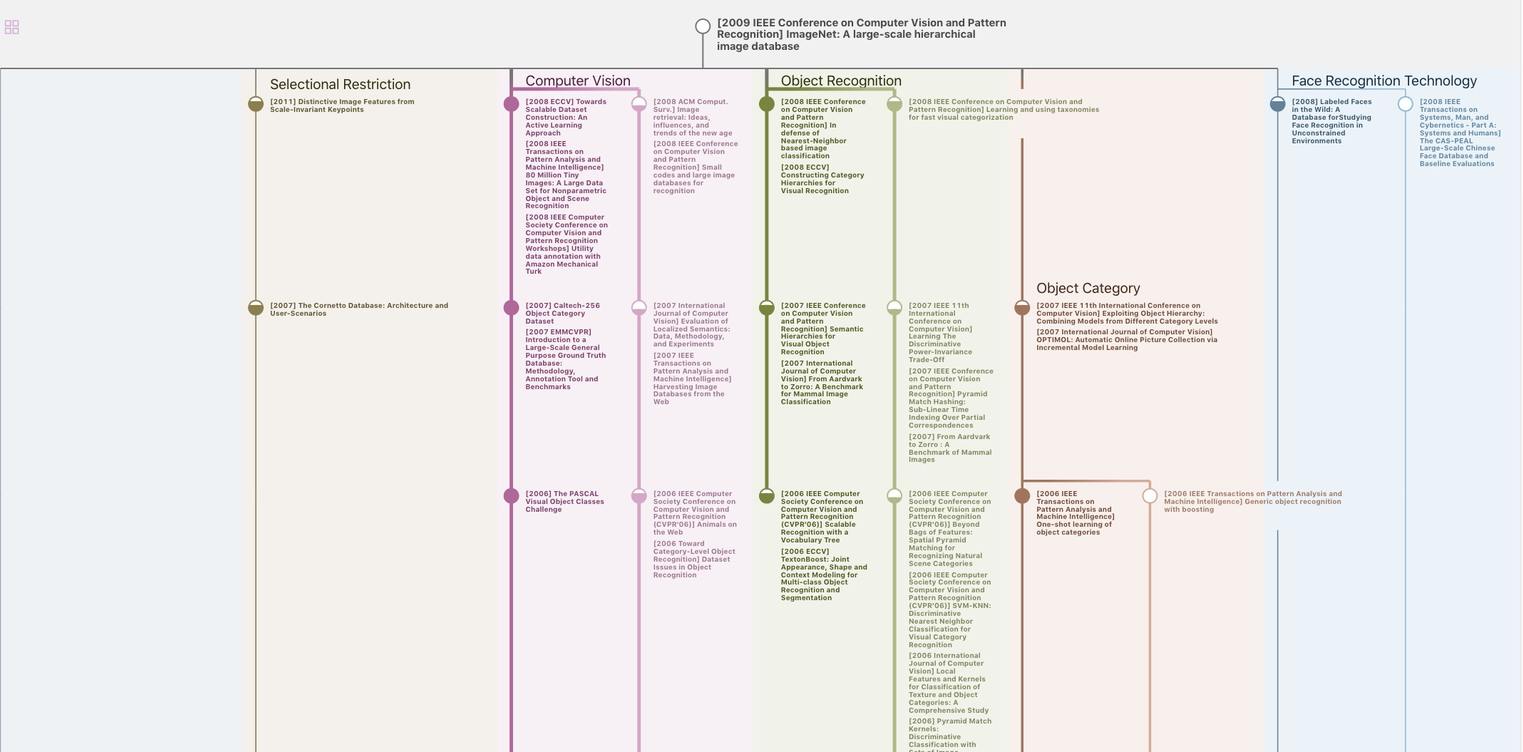
生成溯源树,研究论文发展脉络
Chat Paper
正在生成论文摘要