Every Smile is Unique: Landmark-Guided Diverse Smile Generation
IEEE Conference on Computer Vision and Pattern Recognition(2018)
摘要
Each smile is unique: one person surely smiles in different ways (e.g., closing/opening the eyes or mouth). Given one input image of a neutral face, can we generate multiple smile videos with distinctive characteristics? To tackle this one-to-many video generation problem, we propose a novel deep learning architecture named Conditional Multi-Mode Network (CMM-Net). To better encode the dynamics of facial expressions, CMM-Net explicitly exploits facial landmarks for generating smile sequences. Specifically, a variational auto-encoder is used to learn a facial landmark embedding. This single embedding is then exploited by a conditional recurrent network which generates a landmark embedding sequence conditioned on a specific expression (e.g., spontaneous smile). Next, the generated landmark embeddings are fed into a multi-mode recurrent landmark generator, producing a set of landmark sequences still associated to the given smile class but clearly distinct from each other. Finally, these landmark sequences are translated into face videos. Our experimental results demonstrate the effectiveness of our CMM-Net in generating realistic videos of multiple smile expressions.
更多查看译文
关键词
smile class,input image,multiple smile expressions,realistic videos,face videos,landmark sequences,multimode recurrent landmark generator,generated landmark embeddings,specific expression,conditional recurrent network,single embedding,facial landmark embedding,variational auto-encoder,smile sequences,facial landmarks,facial expressions,CMM-Net,Conditional MultiMode Network,deep learning architecture,video generation problem,distinctive characteristics,multiple smile videos,neutral face,landmark-guided diverse smile generation
AI 理解论文
溯源树
样例
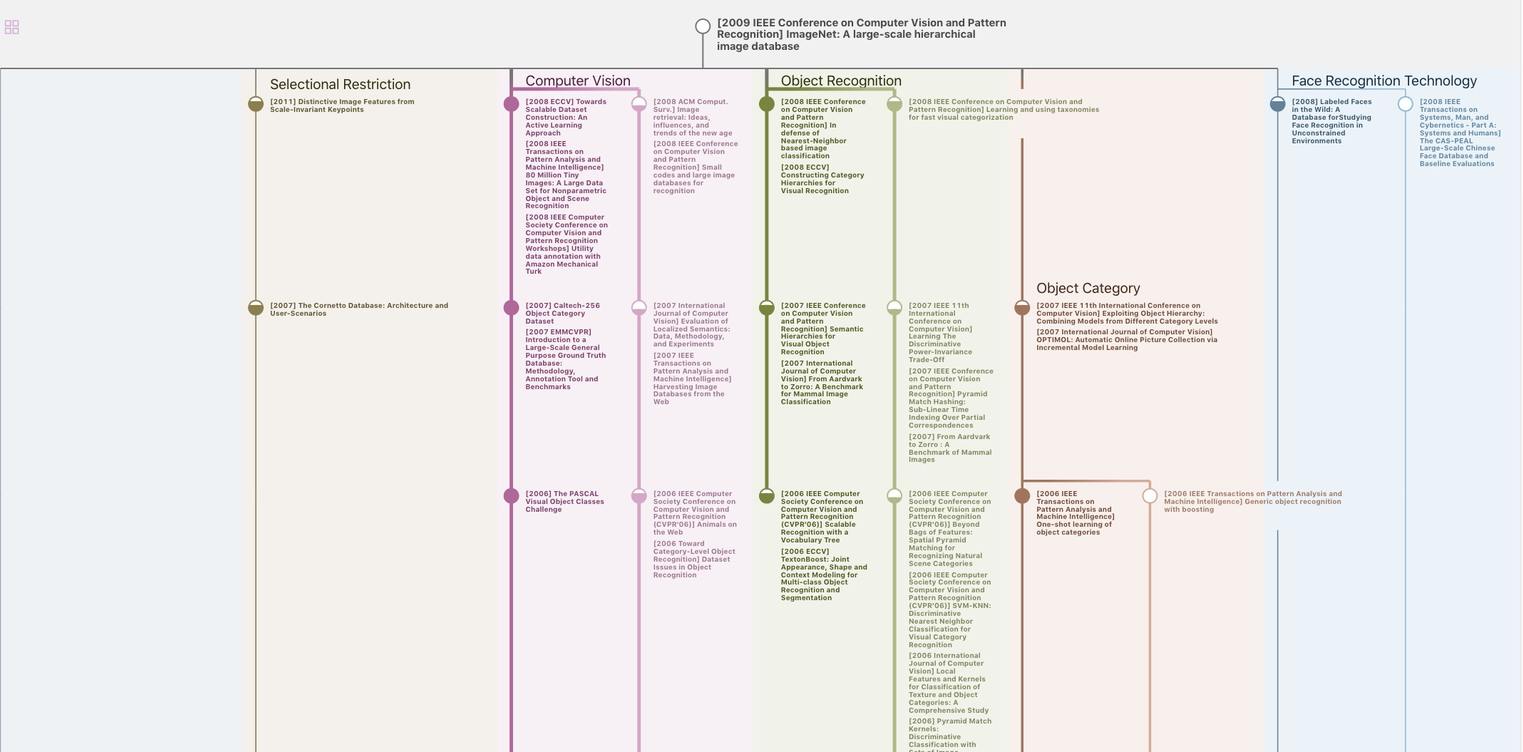
生成溯源树,研究论文发展脉络
Chat Paper
正在生成论文摘要