Evaluation of data driven models for pipe burst prediction in urban water distribution systems
URBAN WATER JOURNAL(2019)
摘要
The water distribution network is one of the most expensive parts of a water supply system. The fundamental variables of a network, material, diameter, length, age, and the hydraulic pressure of pipes are the factors that affect the pipe burst rate (PBR). Establishing a relationship among the burst rate and these factors is an important step to assess the conditions governing the network and preventing significant water leakage. Implementing the data-driven approach in PBR prediction is an effective method to find the relationship. In the present study, Grasshopper Optimization Algorithm-based Support Vector Regression (GOA-SVR), Gaussian Process Regression (GPR), and Artificial Neural Network (ANN) have been developed to predict PBR in an urban area. The results show that the GPR model outperforms other methods. Furthermore, the sensitivity analysis indicates that the pipe age has a negative effect on PBR modeling while the pipe length is the most relevant variable.
更多查看译文
关键词
Data-driven models,machine learning,pipe burst rate,water distribution systems
AI 理解论文
溯源树
样例
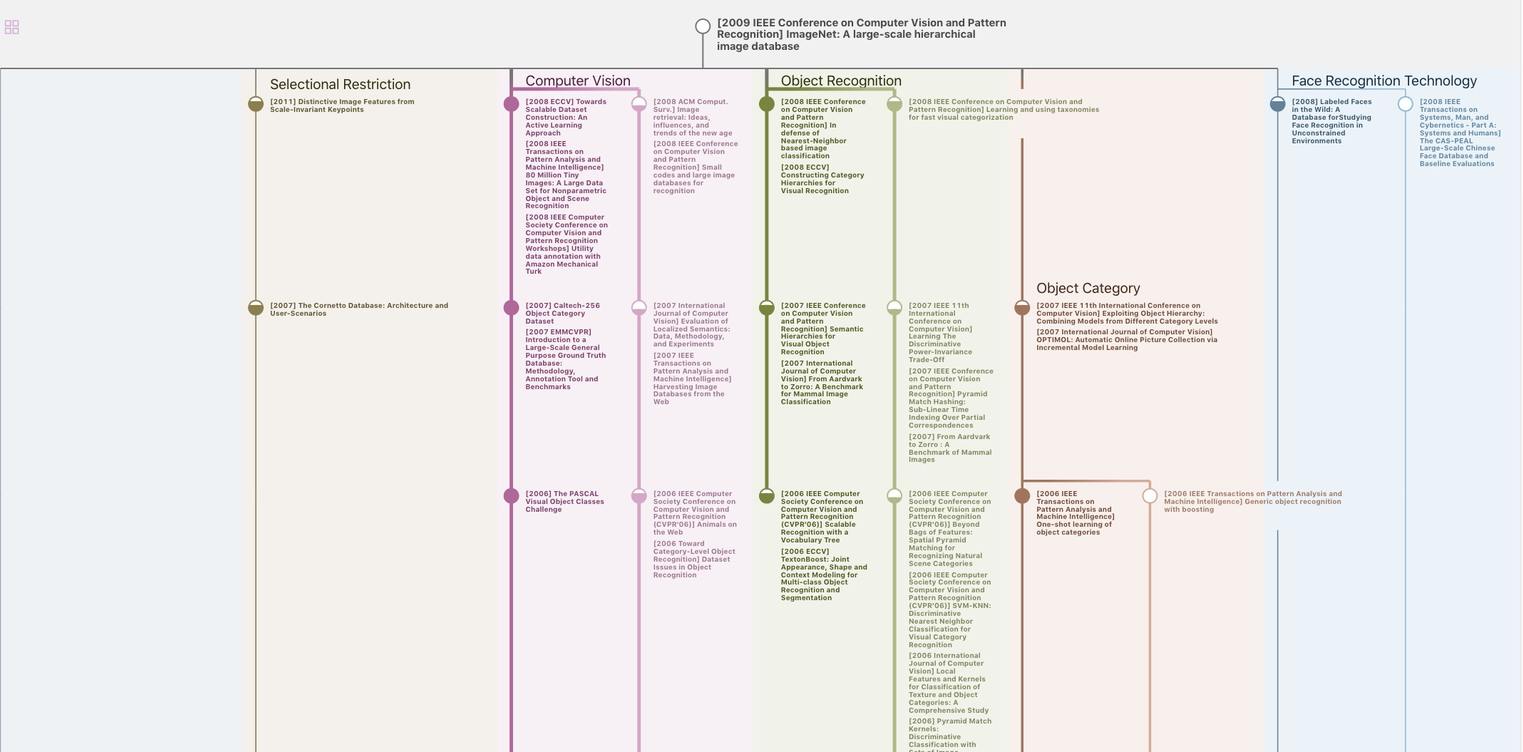
生成溯源树,研究论文发展脉络
Chat Paper
正在生成论文摘要