Variable selection under multicollinearity using modified log penalty
JOURNAL OF APPLIED STATISTICS(2020)
摘要
To handle the multicollinearity issues in the regression analysis, a class of 'strictly concave penalty function' is described in this paper. As an example, a new penalty function called 'modified log penalty' is introduced. The penalized estimator based on strictly concave penalties enjoys the oracle property under certain regularity conditions discussed in the literature. In the multicollinearity cases where such conditions are not applicable, the behaviors of the strictly concave penalties are discussed through examples involving strongly correlated covariates. Real data examples and simulation studies are provided to show the finite-sample performance of the modified log penalty in terms of prediction error under scenarios exhibiting multicollinearity.
更多查看译文
关键词
Grouping effect,modified log penalty,multicollinearity,penalized regression,strictly concave penalty function
AI 理解论文
溯源树
样例
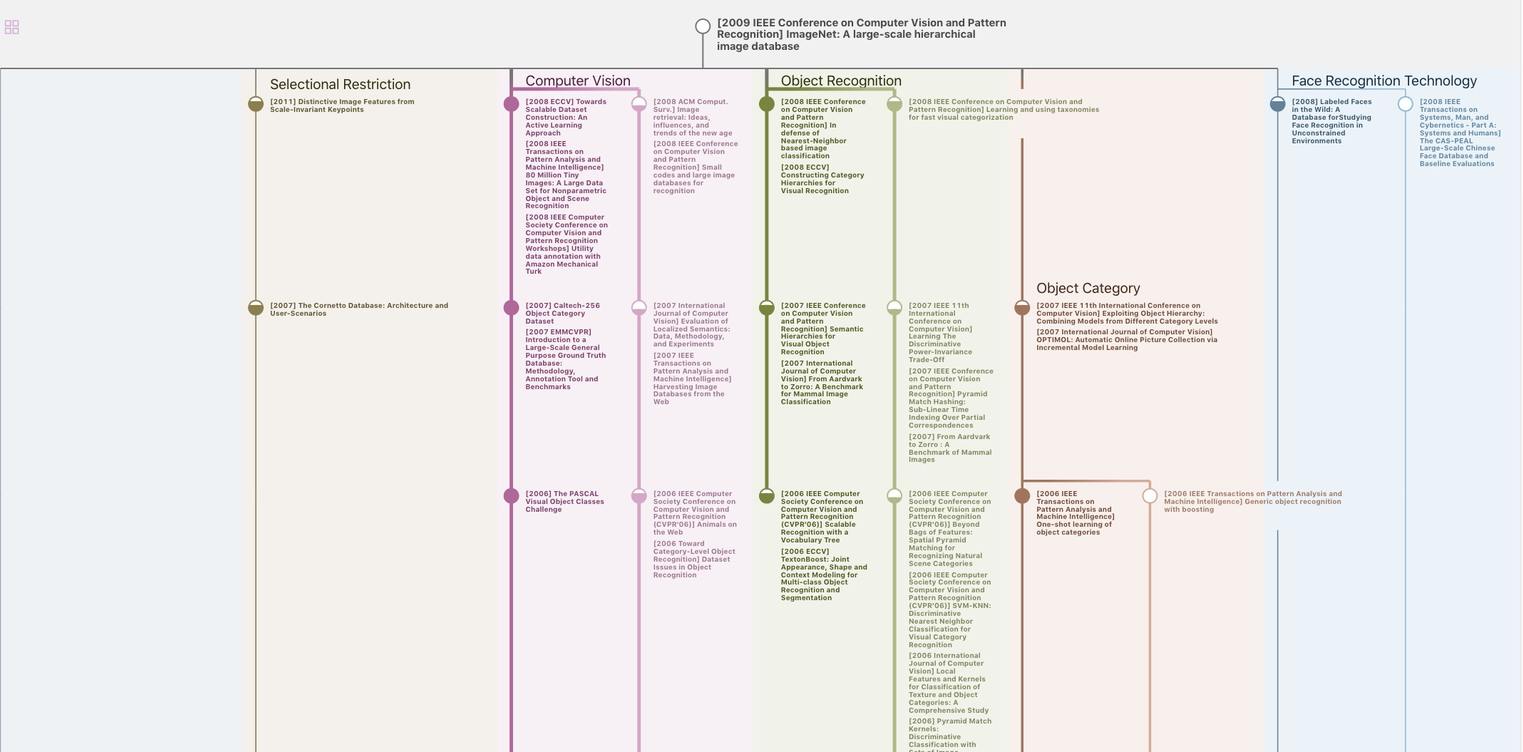
生成溯源树,研究论文发展脉络
Chat Paper
正在生成论文摘要