Learning robust features for planar object tracking
IEEE Access(2019)
摘要
Visual tracking of planar objects with multiple degrees of freedom is a core component for numerous vision-based applications. Generally, since direct methods use all raw pixels in the region of interest to estimate the motion model directly, these methods are sensitive to illumination changes, partial occlusion, and motion blur. Recently, the deep convolutional network has demonstrated remarkable ability in visual tracking via learning robust deep features. In this paper, deep features are used for improving the robustness of direct methods. To learn suitable features in an end-to-end fashion, we employ a novel network architecture, the efficient second-order minimization (ESM) layer, which performs the ESM algorithm on deep feature maps. We train and validate the convolutional features on a synthetic dataset generated from the MS-COCO dataset and evaluate the tracking performance on two challenging, real-world datasets. The experimental results show that the proposed approach outperforms most state-of-the-art methods in various tracking challenges.
更多查看译文
关键词
Visual tracking, homography estimation, convolutional neural network, efficient second-order minimization
AI 理解论文
溯源树
样例
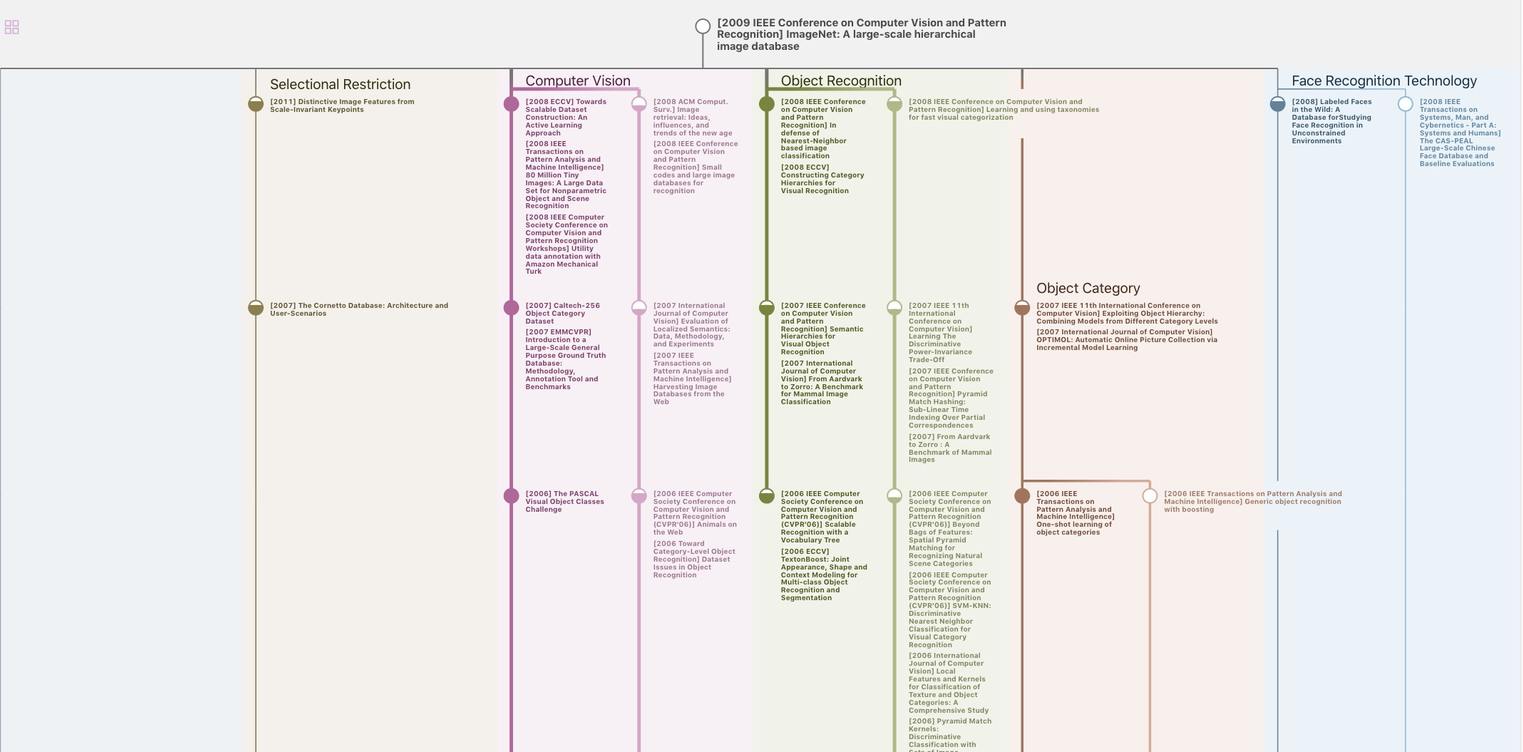
生成溯源树,研究论文发展脉络
Chat Paper
正在生成论文摘要