Abstract 5104: Pan-cancer classification on gene expression data by neural network
Cancer Research(2019)
摘要
Cancer classification based on molecular signatures such as gene expression profiles has been provided insights on causes of the disease or provided guidance for selecting patient’s treatment regimen. We developed a cancer classifier that can identify 13 different types of cancers or a normal tissue based on bulk RNA-seq data as well as single cell RNA-seq data. Training was performed with 6,483 samples from 13 cancer tissues and 640 samples from tumor-adjacent normal tissues in The Cancer Genome Atlas based on 300 most significant genes for the binary classification of each cancer. Then, we compared Neural network, Support vector machine, Naive Bayes and Random Forest methods. Neural network performed consistently better than other methods, even it took shorter time to train. We further applied our approach to bulked single cell data that is generated from single cell RNA-seq and found our model successfully classified cancer types and normal samples. Gene set enrichment analysis comparing cancer types found genes in cell cycle-related pathways, which highlights the differences between cancer types might be in their abilities to grow in different conditions. We also found genes that expressed in multiple cancers that can be novel biomarkers and therapeutic targets. Citation Format: Kijin Yu, Bong-Hyun Kim, Peter Chang Whan Lee. Pan-cancer classification on gene expression data by neural network [abstract]. In: Proceedings of the American Association for Cancer Research Annual Meeting 2019; 2019 Mar 29-Apr 3; Atlanta, GA. Philadelphia (PA): AACR; Cancer Res 2019;79(13 Suppl):Abstract nr 5104.
更多查看译文
AI 理解论文
溯源树
样例
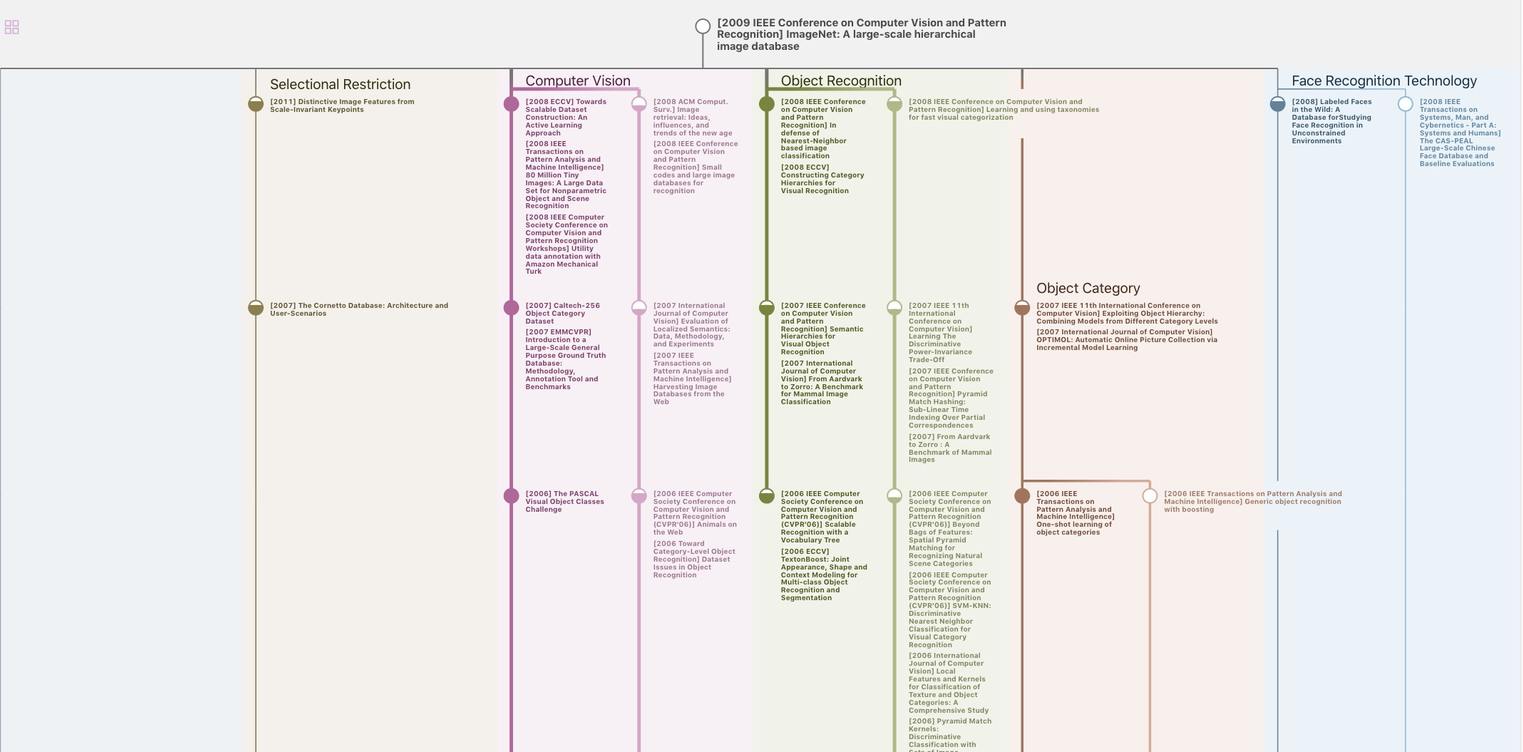
生成溯源树,研究论文发展脉络
Chat Paper
正在生成论文摘要