Open-Domain Why-Question Answering With Adversarial Learning To Encode Answer Texts
57TH ANNUAL MEETING OF THE ASSOCIATION FOR COMPUTATIONAL LINGUISTICS (ACL 2019)(2019)
摘要
In this paper, we propose a method for why-question answering (why-QA) that uses an adversarial learning framework. Existing why-QA methods retrieve answer passages that usually consist of several sentences. These multi-sentence passages contain not only the reason sought by a why-question and its connection to the why-question, but also redundant and/or unrelated parts. We use our proposed Adversarial networks for Generating compact-answer Representation (AGR) to generate from a passage a vector representation of the non-redundant reason sought by a why-question and exploit the representation for judging whether the passage actually answers the why-question. Through a series of experiments using Japanese why-QA datasets, we show that these representations improve the performance of our why-QA neural model as well as that of a BERT-based why-QA model. We show that they also improve a state-of-the-art distantly supervised open-domain QA (DS-QA) method on publicly available English datasets, even though the target task is not a why-QA.
更多查看译文
AI 理解论文
溯源树
样例
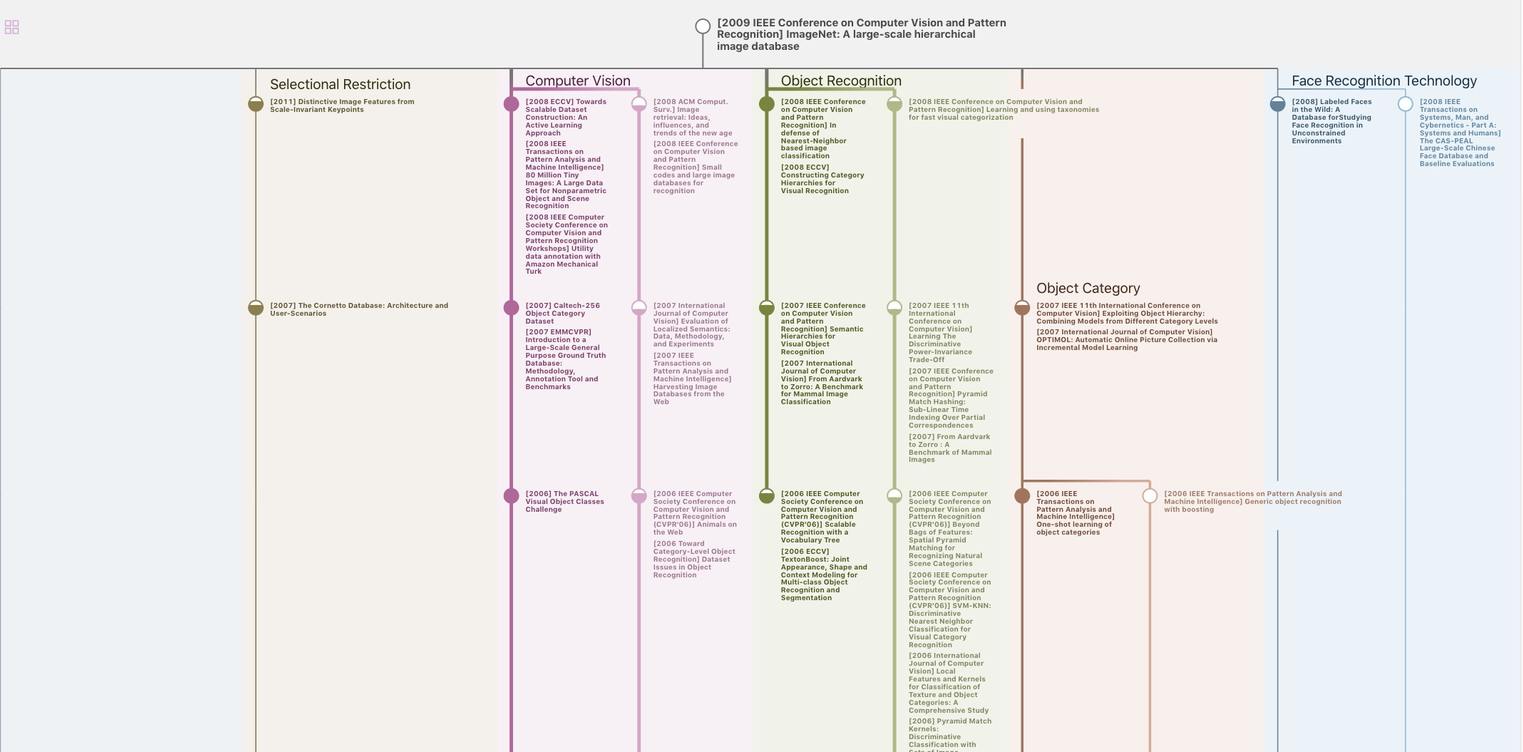
生成溯源树,研究论文发展脉络
Chat Paper
正在生成论文摘要