AB0234 AN INTEGRATED PROTEOMICS AND ANTIBODY ANALYSIS OF THE U-ACT-EARLY TRIAL TO IDENTIFY MARKERS OF TREATMENT RESPONSE AND DISEASE PROGRESSION IN EARLY RHEUMATOID ARTHRITIS
ANNALS OF THE RHEUMATIC DISEASES(2019)
摘要
Background Predictive markers of treatment response in rheumatoid arthritis (RA) are necessary to stratify patients to devise personalised treatment strategies. The U-Act-Early trial [1], in which disease-modifying antirheumatic drugs (DMARD) naive early RA patients initiated either methotrexate (MTX) or tocilizumab (TCZ) in monotherapy or in combination, offers a unique opportunity to assess the capacity of many baseline –omics markers (i.e. genomics, transcriptomics, proteomics, metabolomics, auto-antibodies) to predict clinical outcomes. Objectives To identify predictive markers of treatment response by developing a statistical machine learning methodology for correlating baseline clinical and –omics variables with clinical response data over time. Methods We developed a multi-omics approach that combines longitudinal statistical modelling of clinical data with Bayesian machine learning variable selection to identify the most predictive markers of treatment response. The time course of clinical outcomes was fit using a non-linear mixed longitudinal model, that characterise each patient of the U-Act-Early trial (n=317) by three parameters: a baseline value, an asymptotic value and a velocity (describing the speed at which the asymptote is reached). Supervised machine learning methods (such as Bayesian sparse regression as well as tree-based algorithms) were subsequently employed to find predictive markers for each of the longitudinal model parameters. We specifically focused on 85 auto-inflammatory proteins and 450 auto-antibodies markers, including 41 citrullinated-peptides antigens. The analysis was repeated for the three treatment-arms since DAS28-subscores response markers may vary across treatment strategies and clinical outcomes. We finally investigated whether predictions are enhanced by incorporating known networks of protein-protein interactions, rather than considering the predictive power of each protein independently. Results Preliminary results are that some proteins (e.g. sCD14, VEGF) were moderately predictive of the disease status at baseline. Specifically, the available inflammatory proteins predicted baseline DAS28, swollen and tender joints and ESR with R2 values of 0.25, 0.32, 0.16 and 0 respectively. Surprisingly, these proteins did not improve the prediction of treatment-response (i.e. the velocity and asymptotic parameters) after controlling for the baseline disease status. Protein networks marginally improved prediction when compared to prediction of individual proteins. Citrullinated peptide antigens did not appear predictive of the baseline disease status nor the treatment response. Conclusion In our initial investigation inflammatory proteins, especially when considered as part of interaction networks, appear to be predictive of the baseline status of the disease but not of treatment-response. The most predictive marker of treatment response appears to be disease activity at baseline. References [1] Bijlsma JWJ, et al. Lancet. 2016;388:343-55. Acknowledgement This study was funded by F. Hoffmann-LaRoche Disclosure of Interests Francesco Brizzi Employee of: F. Hoffmann-La Roche, Suleiman A. Khan: None declared, Marco Prunotto Employee of: F. Hoffmann-La Roche, Jenny Devenport Employee of: F. Hoffmann-La Roche, Attila Pethoe-Schramm Shareholder of: F. Hoffmann-La Roche, Employee of: F. Hoffmann-La Roche, Michelle Borm Employee of: An employee of Roche Nederland BV, Johannes WJ Bijlsma Grant/research support from: The department of the author who included patients (JWJB) in the U-Act-Early trial received reimbursements from Roche Nederland BV. JWJB reported grants and fees from Roche, AbbVie, Bristol-Myers Squibb, Merck Sharp & Dohme, Pfizer, and UCB University Medical Center Utrecht, Utrecht University, Consultant for: SUN Pharma, Speakers bureau: Lilly, Roche, Johannes W. G. Jacobs Grant/research support from: Roche, Consultant for: Roche, Floris Lafeber Shareholder of: ArthroSave, Grant/research support from: FOREUM; Dutch Arthritis Society, Paco Welsing: None declared, Tero Aittokallio: None declared, Antoine Soubret Employee of: F. Hoffmann-La Roche
更多查看译文
AI 理解论文
溯源树
样例
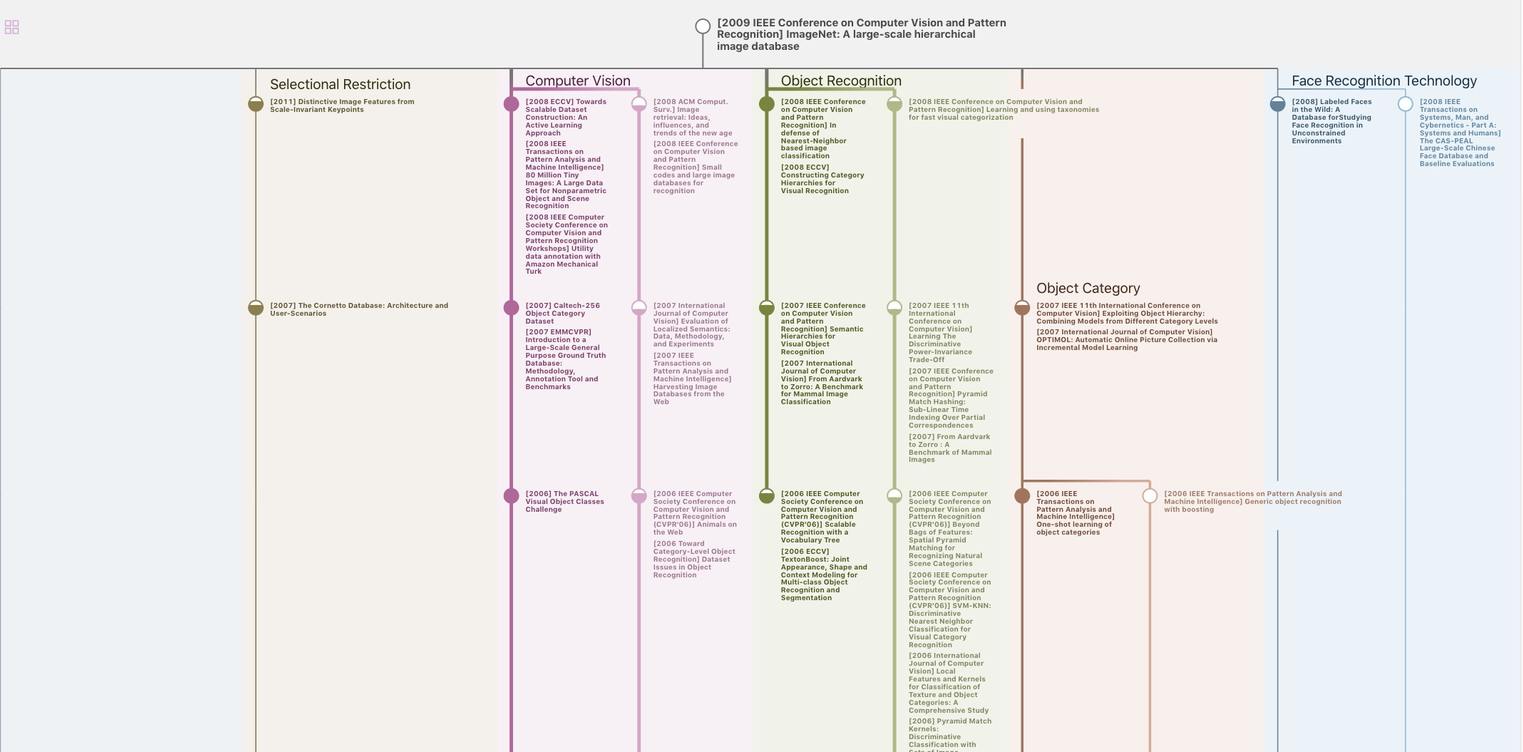
生成溯源树,研究论文发展脉络
Chat Paper
正在生成论文摘要