Solving Bayesian inverse problems from the perspective of deep generative networks
Computational Mechanics(2019)
摘要
Deep generative networks have achieved great success in high dimensional density approximation, especially for applications in natural images and language. In this paper, we investigate their approximation capability in capturing the posterior distribution in Bayesian inverse problems by learning a transport map. Because only the unnormalized density of the posterior is available, training methods that learn from posterior samples, such as variational autoencoders and generative adversarial networks, are not applicable in our setting. We propose a class of network training methods that can be combined with sample-based Bayesian inference algorithms, such as various MCMC algorithms, ensemble Kalman filter and Stein variational gradient descent. Our experiment results show the pros and cons of deep generative networks in Bayesian inverse problems. They also reveal the potential of our proposed methodology in capturing high dimensional probability distributions.
更多查看译文
关键词
Uncertainty quantification,Bayesian inverse problem,Machine learning
AI 理解论文
溯源树
样例
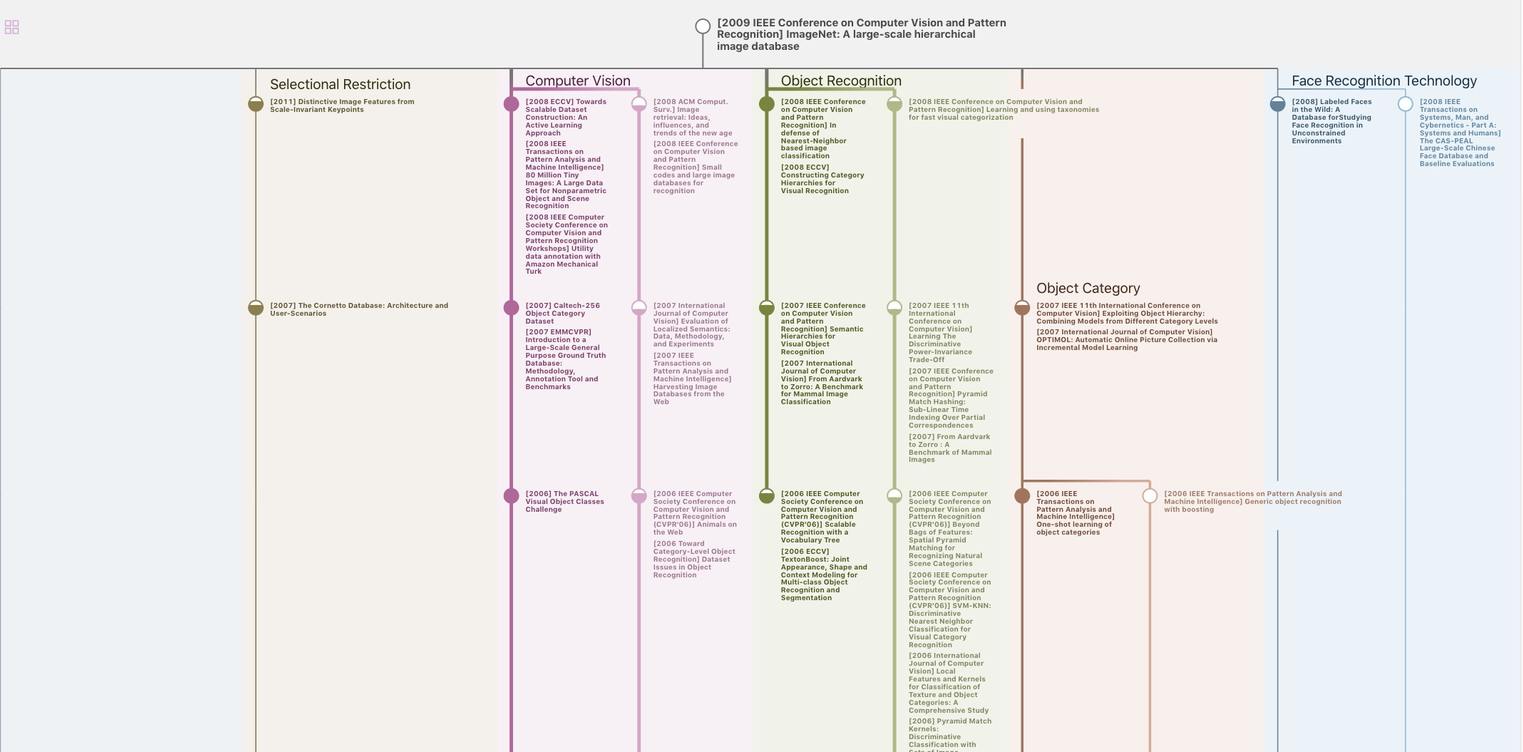
生成溯源树,研究论文发展脉络
Chat Paper
正在生成论文摘要