A novel hybrid network of fusing rhythmic and morphological features for atrial fibrillation detection on mobile ECG signals
Neural Computing and Applications(2019)
摘要
ial fibrillation (AF) is one of the most common arrhythmia diseases, the incidence of which is ascendant with age increase. What’s more, AF is a high-risk factor for stroke, ischemia myocardial and other malignant cardiovascular diseases, which would threaten people’s life significantly. Using a mobile device to screen AF segments is an effective way to reduce the mortality and morbidity of malignant cardiovascular diseases. However, most of existing AF detection methods mainly centered on clinical resting ECG signals and were incapable of processing mobile ECG signals with low signal-to-noise ratio which collected by mobile devices. In this paper, we take advantage of a fully convolutional network variant named U-Net for heart rhythmic information capturing by locating R peak positions as well as calculating RR intervals and a 34-layer residual network for waveform morphological features capturing from ECG signals. Combining both rhythmic information and waveform morphological features, two-layer fully connected networks are employed successively to discriminate AF, normal sinus rhythm , and other abnormal rhythm (other). The extensive experimental results show that our proposed AF our proposed AF screening framework named FRM-CNN can achieve F_1 value of 85.08 ± 0.99
更多查看译文
关键词
Atrial fibrillation,Arrhythmia detection,Fully convolutional network,Residual network,Location of R peak position
AI 理解论文
溯源树
样例
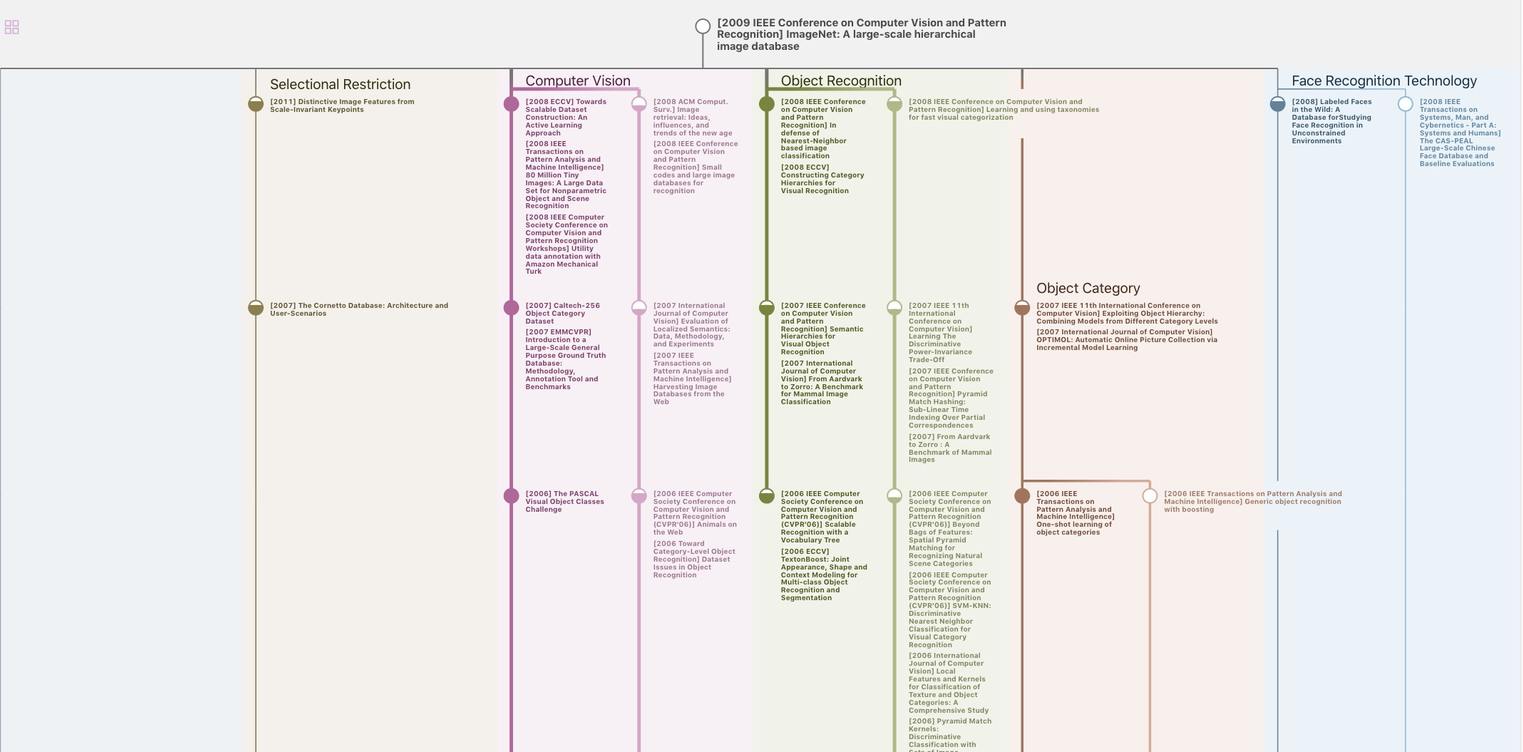
生成溯源树,研究论文发展脉络
Chat Paper
正在生成论文摘要