Flowshop scheduling with artificial neural networks
JOURNAL OF THE OPERATIONAL RESEARCH SOCIETY(2020)
摘要
For effective modelling of flowshop scheduling problems, artificial neural networks (ANNs), due to their robustness, parallelism and predictive ability have been successfully used by researchers. These studies reveal that the ANNs trained with conventional back propagation (CBP) algorithm utilising the gradient descent method are commonly applied to model and solve flowshop scheduling problems. However, the existing scheduling literature has not explored the suitability of some improved neural network training algorithms, such as gradient descent with adaptive learning (GDAL), Boyden, Fletcher, Goldfarb and Shanno updated Quasi-Newton (UQ-N), and Levenberg-Marquardt (L-M) algorithms to solve the flowshop scheduling problem. In this article, we investigate the use of these training algorithms as competitive neural network learning tools to minimise makepsan in a flowshop. Based on training and testing measures, overall results from extensive computational experiments demonstrate that, in terms of the solution quality and computational effort required, the L-M algorithm performs the best followed by the UQ-N algorithm, GDAL algorithm, and the CBP algorithm. These computational results also reveal that the average percent deviation of the makespan from its best solution obtained by using the ANN trained with the L-M algorithm is the least among all examined approaches for the benchmark problem instances.
更多查看译文
关键词
Flowshop scheduling,ANN,gradient descent with adaptive learning algorithm,BFGS update of Quasi-Newton algorithm,L-M algorithm,flowshop heuristics,computational comparisons
AI 理解论文
溯源树
样例
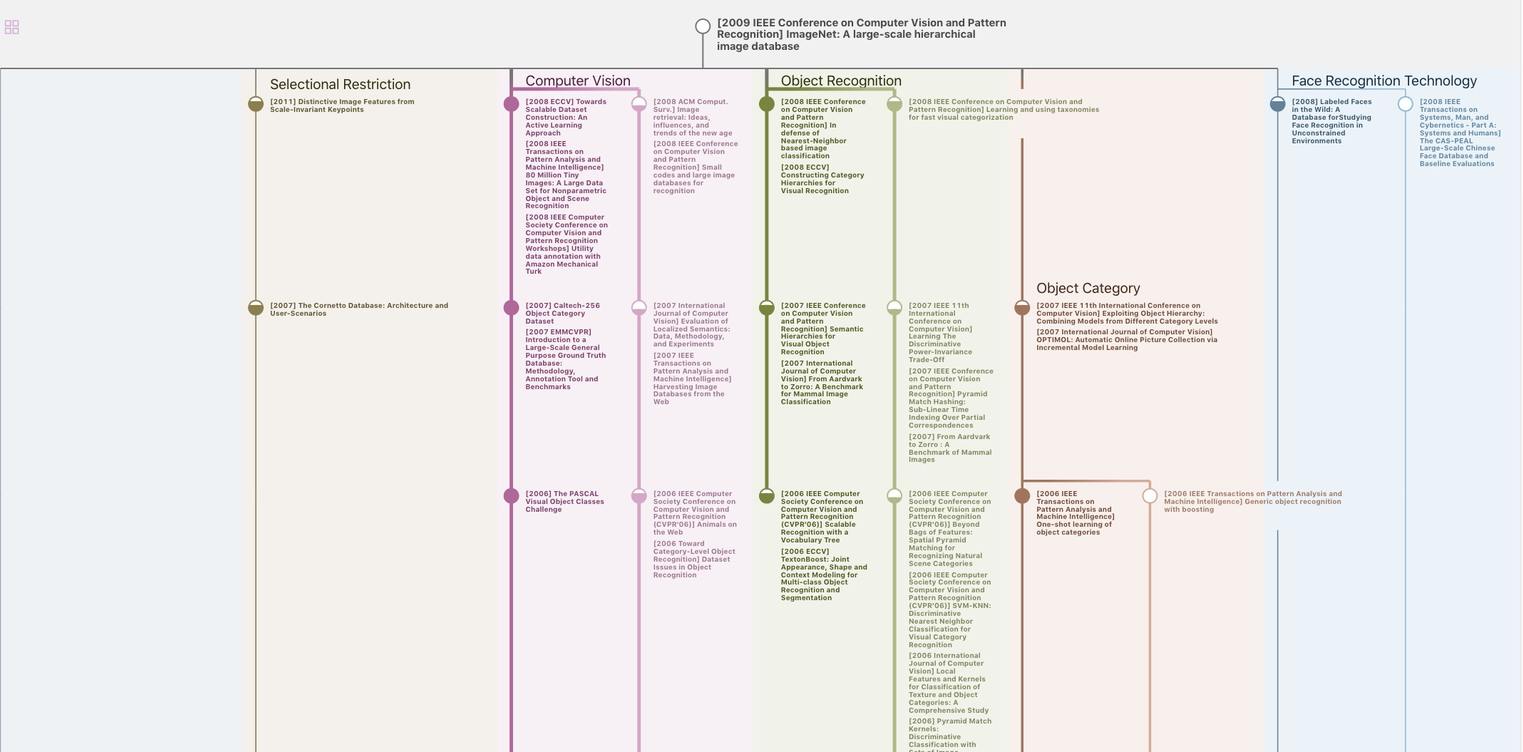
生成溯源树,研究论文发展脉络
Chat Paper
正在生成论文摘要