AB1282 A BIG-DATA APPROACH TO ELECTRONIC HEALTH RECORD DATA – USING DIMENSIONALITY REDUCTION AND CLUSTERING TECHNIQUES TO STUDY LONGITUDINAL RELATIONSHIPS BETWEEN DISEASES
ANNALS OF THE RHEUMATIC DISEASES(2019)
摘要
Background: Hypothesis-free, longitudinal collection of patient health data in the form of Electronic Health Records (EHR) offers a wealth of valuable information on complex, slow-developing diseases in regard to aetiology and comorbidities. Conventional analytical methods are ill suited for the highly dimensional, sparse data contained within EHR, highlighting a need for more sophisticated, high-throughput tools. As t-Distributed Stochastic Neighbour Embedding (t-SNE)[1] and Density-Based Spatial Clustering of Applications with Noise (DBScan)[2] are designed to identify patterns in high-dimensional data with possible non-linear relationships, we hypothesized that these methods can aid identification of associations in diseases with multiple aetiologies. Objectives: Proof of principle showcasing the value of t-SNE and DBScan in detecting longitudinal relationships between diseases in EHR data. Methods: The Partners HealthCare Biobank from Boston, Massachusetts, includes 64,819 patients with longitudinal visit data from hospitals and general practitioners between June 1987 and June 2017. Each visit and procedure (N = 24,377,442) is coupled to an ICD code (International Classification of Disease) describing a disease or examination. We randomly split the data into two datasets of 32,424 and 32,395 individuals: set 1 to optimise t-SNE and DBScan and set 2 for replication. To trim the overly detailed hierarchy of ICDs, we translated them to Phenotype Codes (PheCodes).[3] t-SNE further reduced dimensionality and indicated groups of patients based on their PheCodes and separated patients based on PheCode patterns rather than singular codes. Subsequently DBScan identified clusters of patients in t-SNE space, by grouping patients based on relative Euclidean distance. Finally transition-probability matrices were constructed for all codes in each cluster, from which probabilistic sequences could be constructed. We defined replication as an overlap in ≥25% of the PheCodes between a cluster of set 1 and 2. Similarity was further assessed by calculating the absolute dissimilarity in transition probabilities for codes shared by matched clusters. Results: The average (range) number of codes per individual was 376.3 (1 – 8,419) and 375.9 (1 – 10,315) spread over 4106 (1 - 10,781) and 4153 (1 – 10,746) days for set 1 and 2 respectively. Even though our input data was a sparse, high-dimensional (1,865) matrix of PheCodes, t-SNE and DBScan could clearly separate various unique patient groups with 284 and 295 clusters in set 1 and 2. Clusters consisted of patients with PheCodes of well-defined disease entities such as cardiovascular diseases and neurological disorders with objectively meaningful disease sequences. 34.5% of the clusters identified in set 1 were replicated in set 2 based on our replication criteria. Figure 1 shows the results of each step. Conclusion: Our proof of principle supports the use of unsupervised techniques such as dimensionality reduction and data clustering to identify longitudinal associations between medical events. These methods could proof useful in our quest to identify medical risk factors for incompletely understood diseases. References [1] L.J.P. v.d. Maaten, et al. Journal of Machine Learning Research 9(Nov):2579-2605, 2008. [2] M. Ester, et al. Proceedings of the Second International Conference on Knowledge Discovery and Data Mining: 226-231, 1996. [3] Denny JC, et al. Bioinformatics. 2010;26(9):1205–10 Acknowledgement: Joint last authorship by R. Knevel and E.B. van den Akker. Disclosure of Interests: Marc Maurits: None declared, Thomas Huizinga Consultant for: Merck, UCB, Bristol Myers Squibb, Biotest AG, Pfizer, GSK, Novartis, Roche, Sanofi-Aventis, Abbott, Crescendo Bioscience Inc., Nycomed, Boeringher, Takeda, Zydus, Epirus, Eli Lilly, Soumya Raychaudhuri: None declared, Marcel Reinders: None declared, Elizabeth Karlson: None declared, Erik van den Akker: None declared, Rachel Knevel: None declared
更多查看译文
AI 理解论文
溯源树
样例
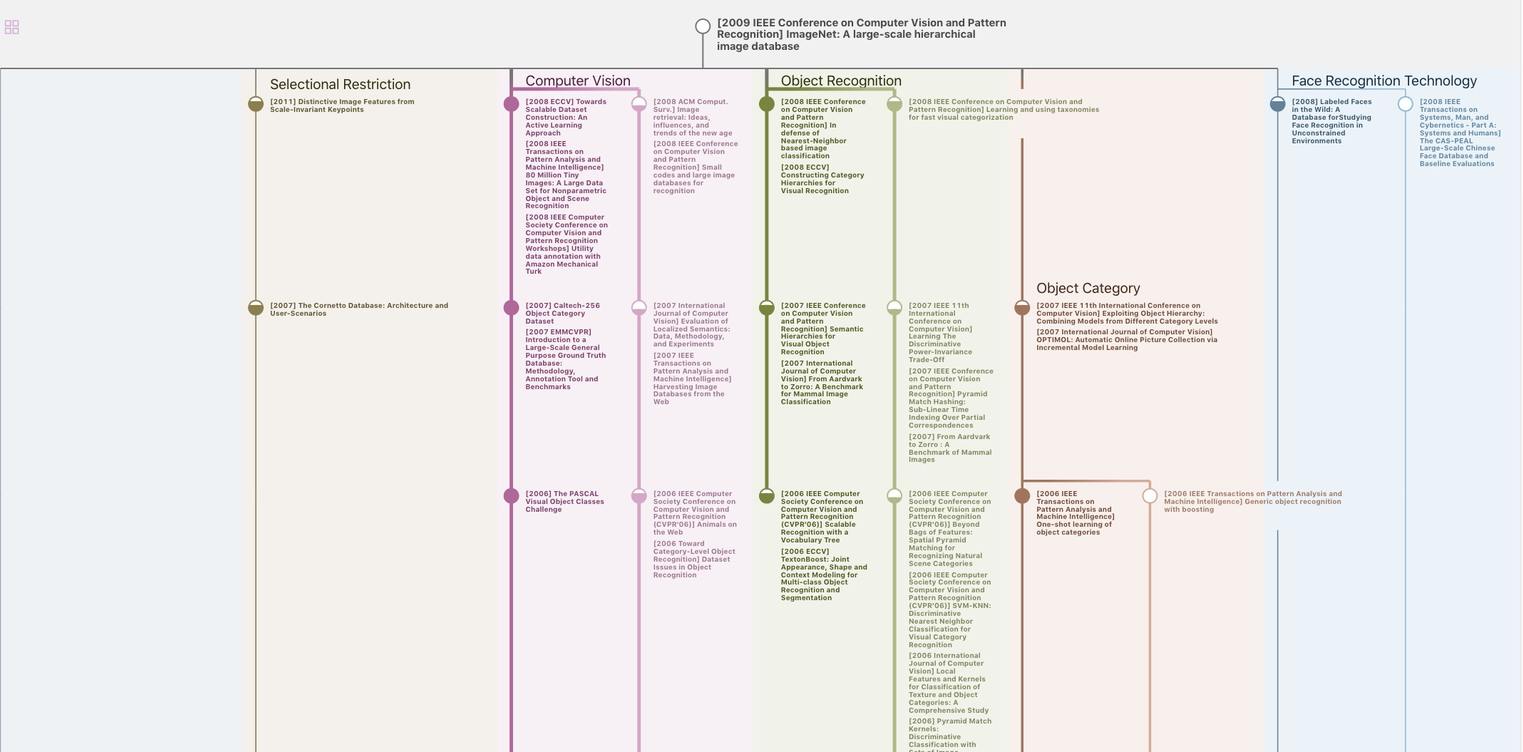
生成溯源树,研究论文发展脉络
Chat Paper
正在生成论文摘要